Introduction
The virtual explosion in digital data has set the stage for major technological breakthroughs in Virtual Reality (VR), Business Intelligence (BI) and Big Data analytics. VR and BI are now essential tools that help organizations make sense of large and diverse datasets in Big Data. This introduction prepares the ground to explore the VR, BI and Big Data synergies and how these technologies drive innovation, enhance decision making, and uncover hidden patterns from complex data systems. In this paper, Big Data, Business Intelligence (BI) and Virtual Reality (VR) will be defined and compared as well as their similarities and differences in order to discuss their importance in the Big Data era, so that the readers in the future will have an idea of the importance of the mentioned technologies in today’s rapidly changing technological world.
Methodology
This study used a systematic literature review to explore the integration of Virtual Reality (VR), Business Intelligence (BI) and Big Data analytics. The purpose was to reveal, assess and integrate the studies in order to determine how these technologies improve data visualization and decision making. A systematic review of the literature was conducted in Scopus, Web of Science, IEEE Xplore, ACM Digital Library and Google Scholar, using Boolean operators with key terms such as:
- “Virtual Reality” AND “Business Intelligence” AND “Big Data”
- “Immersive Analytics” AND “Business Intelligence”
- “Data Visualization” AND “Virtual Reality” AND “Big Data”
The inclusion criteria for the studies were peer-reviewed articles published in English between 2005 and 2023 that focused on the integration of VR, BI and Big Data analytics, while exclusion criteria included non-academic sources, duplicate studies, and unrelated research. The search process was an iterative one, in which the terms were refined based on the initial results, and snowballing technique was used to identify other potentially relevant sources.
Content analysis was used to categorize research according to focus, methodologies, and findings; comparative analysis was used to compare similarities, differences, and research gaps.
Thematic synthesis was used to integrate the findings to report the key themes in relation to how VR can improve data visualization, exploration and decision making. Key data from the selected studies were presented in the tables which contain author(s), year of publication, research focus, methodologies, application domains and findings to help reveal trends.
This research is constrained to English language papers, which may have failed to capture some important studies conducted in other languages. Nevertheless, some studies may not have been incorporated in the search strategy due to the dynamic nature of the research. Moreover, the results are mainly based on academic sources and may not offer a complete picture of the real-world implementation. However, by using a systematic and scientific approach in this study, the integration of VR, BI and Big Data analytics is thoroughly evaluated to strengthen the finding.
Literature Review
Virtual reality in the Era of Big Data
VR can be defined as virtual reality which is a kind of virtual world that is experienced through specific headsets to make the user engage and navigate through digital content in three dimensions as mentioned by Bouloukakis et al. (2018). The information delivery and reception have been revolutionized by Virtual Reality (VR) technologies, and this is more than the traditional 2D versions.
This chapter demonstrates the usage of VR in Big Data and how the use of VR technologies can change the way people interact with data, and discover patterns which were not visible before.
Related Work
The user experience is currently transformed by data visualization techniques and Virtual Reality (VR) and Augmented Reality (AR) technologies that enable immersive exploration of complex datasets with resulting insights.
This chapter looks at VR/AR data visualization applications and their value for decision making in addition to urban planning and industry transformation, specifically for consumer goods, biomedicine and other sectors.
Chen (2005) also highlights the synergy between data visualization and VR through his exploration of different techniques and technologies for visualizing data in virtual reality environments.
The authors Donalek et al. (2014) describe how to design collaborative data visualization on VR platforms, which can be used to demonstrate how such environments can help facilitate multidimensional data exploration and collaborative work among users.
Sharma et al. in 2021 and Hirve et al. in 2017 have also used augmented reality (AR) to support data visualization. Applying AR principles to data visualization, Hirve et al. provide improved data perception with digital information overlaid on physical environments. AR technologies are used to overlay data visualizations onto real environments for intuitive data exploration, as per the paper.
Kumar et al. (2020) describe how MR can be applied for big data visualization and how mixed reality environments, in which physical and digital elements coexist, can enrich data exploration and analysis.
Mariani and Fosso Wamba (2020) present the role of Big Data Analytics Companies (BDACs) in providing big data analytics for consumer goods industries and the impact of data visualization on competitiveness and innovation: Big data analytics. Big data visualization in virtual reality environment is discussed by Teras and Raghunathan (2015) and Moran et al. (2015), and the potential of embodied phenomenological perspectives of interaction and the potential for deeper insights and better decision making is highlighted.
The big data visualization platform that integrates AR and VR for immersive data visualization experiences was developed by Panahi (2017) for the purpose of contributing to the body of work. Venkatesan et al. (2021) examine the current trends and applications of VR and AR technologies in biomedical fields and their potential in medical training, surgical planning, patient care etc. In Internet of Things (IoT) context, Lv (2019) investigated the application of VR and explored how virtual reality technologies can enhance IoT applications and deliver a fully immersive experience for users interacting with IoT data. Zhang et al. (2022) lastly explore the integration of artificial intelligence-enabled sensing technology with VR and AR in the 5G/Internet of Things (IoT) era to contribute to the digital twin concept and enhance immersive experiences and data driven decision making processes. Thus, it can be concluded from the various studies and research works conducted in this area that VR and AR technologies have the ability to change the data visualization as they provide immersive and collaborative experiences that exceed traditional methods and develop new uses across different domains.
Business Intelligence and Big Data Analytics
Business Intelligence can be defined as the systematic use of tools and processes to gather and make sense of data in order to provide organizations with valuable and actionable information with which they can support their decision-making processes. Because of this, it can be concluded that BI systems are necessary in obtaining valuable information from big data sets. Business Intelligence (BI) represents the application of big data analytics for strategic decision making and competitive advantage.
Later in the following chapters, the work of Chen, Chiang, & Storey (2012), Debortoli et al. (2014), and Niu et al. (2021), will be used to explain the relationship between Big Data and Business Intelligence.
The main goal of this paper is to explain how organizations are able to extract valuable knowledge from the large and complex data sets with the help of Business Intelligence tools and methods and then develop business strategies and improve operational performance.
Secondly, it will discuss the skills and competencies of the BI and Big Data specialists (Debortoli et al., 2014) as well as typical problems and misconceptions of the BI and Big Data analytics (Kimble, Milolidakis, 2015). As shown in the BI literature, this chapter will hopefully help readers understand how BI can help foster organizational innovation and effectiveness in the Big Data environment.
Related Work
Large diverse data sets are a source of greater strategic insight through Business Intelligence (BI) and Big Data Analytics that enables organizations to make better strategic decisions and improve competitive standing. This chapter assembles a vast array of research and studies that detail the shift brought about by BI and its connection to the new Big Data Analytics environment.
Chen, Chiang, and Storey (2012) are about how the concept of BI and analytics is shifting with the rise of big data and how the BI tools assist organizations in extracting and utilizing decision-making relevant insights from large and complex data sets. The evolution of BI and its connection with Big Data Analytics is also analyzed by Debortoli, Müller, & vom Brocke (2014) who investigate the competencies of BI and big data specialists and the differences in the required skills to be successful in these positions.
Dedić & Stanier (2017) aim at defining the notion of Business Intelligence, big data, data analytics, and knowledge discovery and developing a conceptual framework for the integration of these concepts in the organizational decision-making process. The development of business intelligence and its use in big data as discussed by El Bousty et al. (2018) focuses on the need to combine business intelligence tools with big data analytics in order to produce valuable insights that can improve organizational performance.
The basic concepts, methods and applications of Business Intelligence and big data analytics are discussed in He’s (2016) paper while Jin & Kim (2018) present a case study of the use of BI tools in the logistics industry and how the integration of the tools can improve supply chain management and sustainability.
In their 2015 paper, Kimble & Milolidakis work to eliminate widespread misconceptions about Business Intelligence (BI) and big data while providing a critical evaluation of the incorrect beliefs demonstrated in the literature. The research landscape in Business Intelligence and big data analytics receives a detailed analysis of its trends along with research trajectories through Liang & Liu’s 2018 bibliometric study.
Mashingaidze and Backhouse (2017) present a summary of the literature concerning definitions of big data, business intelligence (BI), and business analytics and build on existing definitions to understand the changing nature of business intelligence and big data analytics. Organizational business intelligence decision-making roles are detailed by Niu et al. (2021) who demonstrate how big data analytics produces actionable insights that drive strategic outcomes.
Olszak (2020) discusses the connection between business intelligence and big data, explaining how organizations can use sophisticated analytics techniques to extract valuable insights from large datasets. The paper by Praful Bharadiya (2023) compares and contrasts Business Intelligence, Artificial Intelligence, and Big Data Analytics to understand their individual strengths and uses.
Ram, Zhang and Koronios (2016) perform a qualitative study to identify the implications of big data analytics for business intelligence practices in China; Sun, Zou and Strang (2015) examine the big data analytics as a service for business intelligence, including the opportunities and challenges of cloud-based business intelligence solutions.
In their 2017 paper, Wani & Jabin explore big data challenges and techniques for business intelligence applications and provide practical insights for organizations. Wixom et al. (2014) discuss the current academic state of business intelligence research by examining the effects big data has on business intelligence practices and methodologies.
The synergies between big data analytics and business intelligence and advanced analytics techniques like data mining and machine learning are explored by Yafooz et al. (2019) in the context of decision-making in different business fields.
The various studies and research initiatives clearly show that Business Intelligence and Big Data Analytics are vital to organizational success, providing effective tools that extract insights and power strategic decision-making in the digital age.
Big Data Analytics
Big Data describes datasets which traditional data processing applications cannot handle because they become too large and too complex. Big Data includes both structured and unstructured data collected from sources including social media platforms together with sensors and transaction logs (Praful Bharadiya, 2023).
Big Data has brought about the necessity of analytics as organizations seek to generate value from their data assets. Modern data-driven decision-making now depends on Big Data analytics which helps organizations extract valuable insights from extensive complex datasets. Big Data analysis functions as an essential method to unlock meaningful values from extensive diverse datasets.
Big Data allows organizations to extract important and relevant information that can be used to improve decision making and increase innovation through the use of machine learning and predictive analytics methods as recommended by Ram et al. (2016), Wixom et al. (2014). It is with the help of data analysis that organizations can gain some important knowledge on customer behavior as well as market movements and competition pressures according to Wani and Jabin (2017). To completely harness the potential of Big Data analytics, organizations must resolve issues regarding data quality as well as privacy risks and scalability problems according to Kimble and Milolidakis (2015).
Results
In this chapter, all research works presented in previous chapters will be analyzed. An overview of the selected literature will be made in tabular form, which will contain columns that are common to all of them. In this way, works can be categorized, and relevant conclusions can be drawn. All papers are sorted chronologically.
The first table will analyze works from the field of Virtual Reality.
The columns that the tables contain are:
– References
– Research Focus
– Methodologies
– Application Domains
Table 1: Characteristics of VR Literature
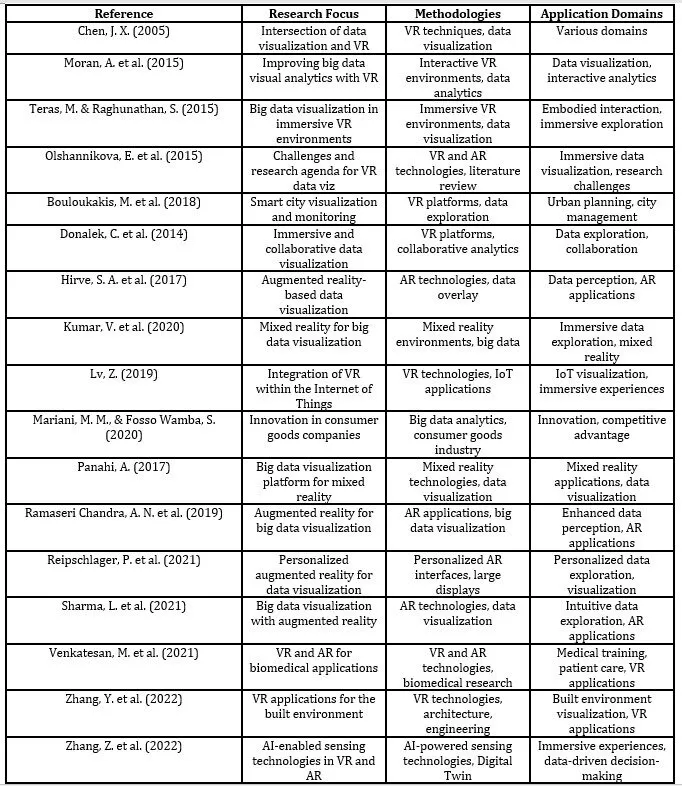
Big Data has enabled Virtual Reality (VR) literature research to advance data visualization along with exploration methods for decision-making processes. Here’s how these findings relate to the era of Big Data:
Diverse Application Domains
The applications of 3VR are to extend the capabilities of current technologies in smart city visualization, collaborative data exploration, and augmented reality-based visualization. These applications use the vast datasets from IoT sensors, social media and other sources to provide immersive experiences for users interacting with complex data environments. Of course there are other areas, but they are not highlighted in the selected works.
Methodological Approaches
The methods used in VR research such as VR platforms, immersive environments and systematic literature reviews, are generally aimed at collecting and representing large scale datasets which are a hallmark of Big Data. The goal of researchers is to create large VR solutions that can handle and visualize large amounts of data to obtain relevant findings.
Emphasis on Immersive Experiences
The VR technologies offer immersive experiences to enhance Big Data perception through interactive and intuitive visualization tools. Researchers are working to make users immersed in virtual environments so they can explore and analyze data to provide decision-makers the ability to detect patterns and trends in large complex datasets.
Focus on Challenges and Opportunities
The VR literature has mainly focused on how to address the complexities of Big Data visualization on challenges and research agendas. This is because researchers want to address these challenges for instance, scalability, interoperability, usability of Big Data so that the potential of VR in generating meaningful insights can be maximized.
Table 2: Characteristics of BI Literature
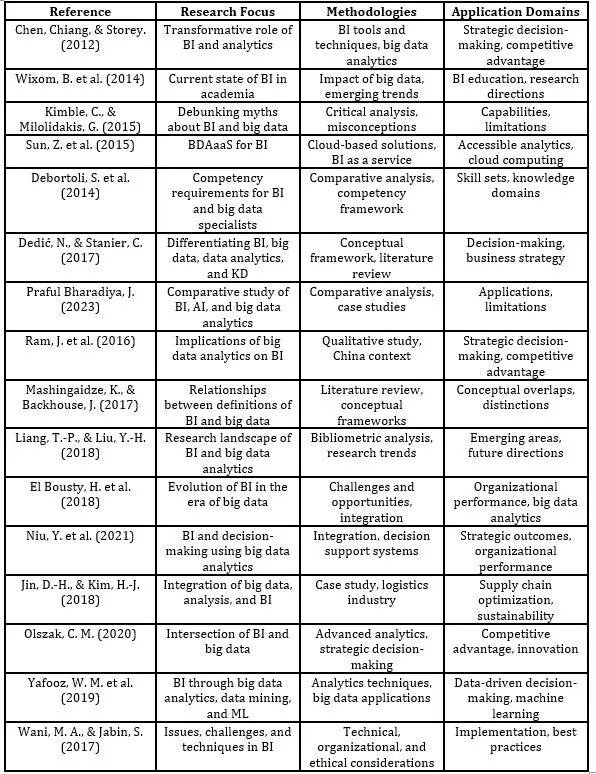
The emergence of Big Data has caused a significant shift in Business Intelligence (BI) research directions methods and applications according to existing BI literature. Here’s how BI findings are intertwined with the era of Big Data:
Strategic Decision-Making
The primary focus of BI research involves utilizing the analytical capabilities of Big Data to support strategic decision making and create competitive market positions. Through the analysis of extensive datasets, organizations obtain actionable insights which enable informed business strategy development and operational performance enhancement as data complexity continues to grow.
Methodological Diversity
Challenges and opportunities of Big Data are addressed through both qualitative research and comparative analyses, and methodically. Also, the existing literature reviews advanced analytics techniques, machine learning algorithms, and data mining methodologies for extracting valuable insights from massive datasets.
Focus on Competency and Skill Development
Big Data has shifted the attention of business intelligence (BI) literature to skill development for handling large, complex data sets. Research is directed toward how the nature of the BI roles is changing and the requirement for data-driven decision makers who can exploit Big Data analytics.
Integration with Big Data Analytics
Furthermore, BI literature is also reviewing the best practice in integrating BI tools and techniques with advanced Big Data analytics methodologies according to the literature review. Here, the researchers are focusing on how organizations can utilize big data platforms with predictive analytics and real time data processing to get actionable insights and drive innovation in different industries.
Addressing Challenges and Myths
In BI literature, the researchers focus on the most popular challenges and misconceptions in Big Data analytics; majorly, these are related to data quality, privacy, and scalability issues. This paper investigates and disputes common misconceptions about Big Data and its applications in Business Intelligence and the actual capabilities and limitations of Big Data. The VR and BI literature helps to identify Big Data potential for strategic decision-making and organizational innovation through the use of immersive data visualization for both fields. The research by professionals in the field brings forward innovative technological and conceptual strategies to overcome the difficult organisational digital environment challenges to unlock the Big Data analytical capabilities and solve operational challenges to create long-term market advantages.
Discussion
Integration of VR, BI, and Big Data Analytics
Big Data Analytics, Business Intelligence and Virtual Reality are closely related when used within their specific domain to support data visualization and exploration and decision making within that domain. Big data and strategic outcomes can be analyzed with relevant insights by organizations and strategic outcomes enhanced when Business Intelligence and Big Data Analytics are combined with Virtual Reality.
Enhancing Data Visualization and Exploration
VR technologies offer immersive experiences which enhance data visualization and exploration practices. Organizations can convert extensive datasets into dynamic interactive visualizations using VR platforms that allow users to uncover new data perspectives with hidden patterns. The application of VR in smart city visualization and collaborative data exploration has been verified as per the studies of Bouloukakis et al. (2018) and Donalek et al. (2014).
Driving Informed Decision-Making
BI together with Big Data Analytics helps organizations to gain relevant and useful patterns and trends from large and complex datasets which are used to support strategic decision making. Chen, Chiang, & Storey (2012) have also looked at the role of BI and analytics in exploiting the value of big data for organizational efficiency. This opens up the possibility for organizations to improve on their market standing and promote digital innovation through the use of BI tools with traditional analytics techniques.
Future Directions and Research Opportunities
The following are several acquirable areas for future research and innovation in the integration of VR, BI and Big Data Analytics. Other areas such as personalized data visualization, AI-enabled sensing technologies, and cloud-based analytics platforms can be used to improve the effectiveness of VR and BI in employing big data for organizational effectiveness. Additionally, the ethical implications of immersive data visualization and decision-making processes are an important area of research.
Study Limitations
Even with the numerous benefits that can be derived from the use of VR, BI, and Big Data Analytics, there is the problem of scalability, interoperability, and data privacy issues. Research done by Olshannikova et al. (2015) and Wani & Jabin (2017) stress both the problems and benefits associated with the use of immersive data visualization for decision making in the era of Big Data analytics. It is only when researchers, practitioners, and policy makers cooperate that we can create solutions that are scalable, easy to use, ethical, and legal.
Conclusion
In this study, we aimed to explore how Virtual Reality (VR), Business Intelligence (BI), and Big Data connect with each other and how they transform their connection into actionable insights in data-driven decision-making today. By studying the literature in detail, we have learned multiple important findings as well as multiple themes and differences among the three domains.
As a result, VR can act as a powerful tool that enhances data visualization and produces more in-depth insights and better communication of complex datasets through its ability to provide immersive and interactive experiences. The application of VR studies in smart city visualization, biomedical research, and consumer goods innovation presents ways in which it can revolutionize data interaction and comprehension.
Business Intelligence (BI) remains an essential part of organizational decision-making processes, using more advanced analytics to extract valuable information from large and complex data sources. In the light of the challenges and opportunities that come with big data, BI tools and methodologies are crucial in harnessing the potential of data to achieve strategic objectives and gain a competitive edge. Big Data serves as the bedrock that makes the integration of VR with Business Intelligence possible and is the source of data that yields valuable insights.
The rising need for better data management strategies comes from explosive growth in data volume velocity and variety. Organizations that adopt big data technologies and methodologies are able to reveal valuable insights, optimize operations and drive innovation.
Implications for Practice and Future Research: The application of VR, BI, and Big Data offers organizations a unique chance to enhance their understanding of data, support better decision-making, and promote innovative practices. Data privacy, security, and ethical risks need thorough examination to prevent maladaptive uses of data-driven technologies. Further study could focus on new VR methods for data visualization, BI tool development, and analysis of big data trends.
Limitations and Scope for Further Exploration: There is no such problem as the research being incomplete, although this study presents comprehensive findings about the VR, BI and Big Data intersection. The continually changing worlds of technology and data analytics present challenges to maintaining alignment with current trends and developments. Future work could focus on detailed industry studies, interdisciplinary strategies, and the evaluation of how new technologies such as artificial intelligence and machine learning affect VR, BI and Big Data integration.
The integration of Virtual Reality, Business Intelligence and Big Data provides significant prospects for transforming decision-making and innovative practices to shape enterprises of the future. Through a coordinated application of these domains and challenge mitigation, organizations will gain access to new digital age opportunities which drive growth and enhance competitiveness and value creation.
References
- Bouloukakis, M., Partarakis, N., Drossis, I., Kalaitzakis, M. i Stephanidis, C. (2018). ‘Virtual reality for smart city visualization and monitoring’, Progress in IS, 1-18. https://doi.org/10.1007/978-3-319-99444-4_1
- Chen, H., Chiang, R. H. L. i Storey, V. C. (2012). ‘Business intelligence and analytics: From big data to big impac’, MIS Quarterly, 36(4), 1165-1188. https://doi.org/10.2307/41703503
- Chen, J. X. (2005). ‘Data visualization and virtual reality’, Handbook of Statistics, 24, 539-563. https://doi.org/10.1016/s0169-7161(04)24017-2
- Debortoli, S., Müller, O. i vom Brocke, J. (2014). ‘Vergleich von Kompetenzanforderungen an Business-Intelligence- und Big-Data-Spezialisten’, Wirtschaftsinformatik, 56(5), 315-328. https://doi.org/10.1007/s11576-014-0432-4
- Dedić, N. i Stanier, C. (2017). ‘Towards differentiating business intelligence, big data, data analytics and knowledge discovery’, Innovations in Enterprise Information Systems Management and Engineering, 114-122. https://doi.org/10.1007/978-3-319-58801-8_10
- Donalek, C., Djorgovski, S. G., Cioc, A., Wang, A., Zhang, J., Lawler, E., Yeh, S., Mahabal, A., Graham, M., Drake, A., Davidoff, S., Norris, J. S. i Longo, G. (2014). ‘Immersive and collaborative data visualization using virtual reality platforms’, 2014 IEEE International Conference on Big Data (Big Data), 1-8. https://doi.org/10.1109/bigdata.2014.7004282
- El Bousty, H., Krit, S., Elasikri, M., Dani, H., Karimi, K., Bendaoud, K. i Kabrane, M. (2018). ‘Investigating business intelligence in the era of big data’, U: Proceedings of the Fourth International Conference on Engineering & MIS 2018.https://doi.org/10.1145/3234698.3234723
- He, X. J. (2016). ‘Business intelligence and big data analytics: An overview’, Communications of the IIMA, 14(3), 1-10.https://doi.org/10.58729/1941-6687.1358
- Hirve, S. A., Kunjir, A., Shaikh, B. i Shah, K. (2017). ‘An approach towards data visualization based on AR principles’, 2017 International Conference on Big Data Analytics and Computational Intelligence (ICBDAC), 1-6.https://doi.org/10.1109/icbdaci.2017.8070822
- Jin, D.-H. i Kim, H.-J. (2018). ‘Integrated understanding of big data, big data analysis, and business intelligence: A case study of logistics’, Sustainability, 10(10), 3778. https://doi.org/10.3390/su10103778
- Kimble, C. i Milolidakis, G. (2015). ‘Big data and business intelligence: Debunking the myths’, Global Business and Organizational Excellence, 35(1), 23-34. https://doi.org/10.1002/joe.21642
- Kumar, V., Sharma, D. K. i Mishra, V. K. (2020). ‘Visualizing big data with mixed reality’, 2020 9th International Conference on System Modeling and Advancement in Research Trends (SMART), 1-6. https://doi.org/10.1109/smart50582.2020.9337072
- Liang, T.-P. i Liu, Y.-H. (2018). ‘Research landscape of business intelligence and big data analytics: A bibliometrics study’, Expert Systems with Applications, 111, 2-10. https://doi.org/10.1016/j.eswa.2018.05.018
- Lv, Z. (2019). ‘Virtual reality in the context of internet of things’, Neural Computing and Applications, 32(13), 9593-9602. https://doi.org/10.1007/s00521-019-04472-7
- Mariani, M. M. i Fosso Wamba, S. (2020). ‘Exploring how consumer goods companies innovate in the digital age: The role of big data analytics companies’, Journal of Business Research, 121, 338-352. https://doi.org/10.1016/j.jbusres.2020.09.012
- Mashingaidze, K. i Backhouse, J. (2017). ‘The relationships between definitions of big data, business intelligence and business analytics: A literature review’, International Journal of Business Information Systems, 26(4), 488-500. https://doi.org/10.1504/ijbis.2017.087749
- Moran, A., Gadepally, V., Hubbell, M. i Kepner, J. (2015). ‘Improving big data visual analytics with interactive virtual reality’, 2015 IEEE High Performance Extreme Computing Conference (HPEC), 1-6. https://doi.org/10.1109/hpec.2015.7322473
- Niu, Y., Ying, L., Yang, J., Bao, M. i Sivaparthipan, C. B. (2021). ‘Organizational business intelligence and decision making using big data analytics’, Information Processing & Management, 58(6), 102725. https://doi.org/10.1016/j.ipm.2021.102725
- Olshannikova, E., Ometov, A., Koucheryavy, Y. i Olsson, T. (2015). ‘Visualizing big data with augmented and virtual reality: Challenges and research agenda’, Journal of Big Data, 2(1), 1-20. https://doi.org/10.1186/s40537-015-0031-2
- Olszak, C. M. (2020). Business intelligence and big data. https://doi.org/10.1201/9780429353505
- Panahi, A. (2017). Big data visualization platform for mixed reality. Virginia Commonwealth University, Virginia.
- Praful Bharadiya, J. (2023). ‘A comparative study of business intelligence and artificial intelligence with big data analytics’, American Journal of Artificial Intelligence. https://doi.org/10.11648/j.ajai.20230701.14
- Ram, J., Zhang, C. i Koronios, A. (2016). ‘The implications of big data analytics on business intelligence: A qualitative study in China’, Procedia Computer Science, 87, 221-226. https://doi.org/10.1016/j.procs.2016.05.152
- Ramaseri Chandra, A. N., El Jamiy, F. i Reza, H. (2019). ‘Augmented reality for big data visualization: A review’, 2019 International Conference on Computational Science and Computational Intelligence (CSCI), 1-6. https://doi.org/10.1109/csci49370.2019.00238
- Reipschlager, P., Flemisch, T. i Dachselt, R. (2021). ‘Personal augmented reality for information visualization on large interactive displays’, IEEE Transactions on Visualization and Computer Graphics, 27(2), 1182-1192. https://doi.org/10.1109/tvcg.2020.3030460
- Sharma, L., Anand, S., Sharma, N. i Routry, S. K. (2021). ‘Visualization of big data with augmented reality’, 2021 5th International Conference on Intelligent Computing and Control Systems (ICICCS), 1-6. https://doi.org/10.1109/iciccs51141.2021.9432383
- Sun, Z., Zou, H. i Strang, K. (2015). ‘Big data analytics as a service for business intelligence’, Open and Big Data Management and Innovation, 200-211. https://doi.org/10.1007/978-3-319-25013-7_16
- Teras, M. i Raghunathan, S. (2015). ‘Big data visualisation in immersive virtual reality environments: Embodied phenomenological perspectives to interaction’, ICTACT Journal on Soft Computing, 05(04), 1009-1015. https://doi.org/10.21917/ijsc.2015.0141
- Venkatesan, M., Mohan, H., Ryan, J. R., Schurch, C. M., Nolan, G. P., Frakes, D. H. i Coskun, A. F. (2021). ‘Virtual and augmented reality for biomedical applications’, Cell Reports Medicine, 2(7), 100348. https://doi.org/10.1016/j.xcrm.2021.100348
- Wani, M. A. i Jabin, S. (2017). ‘Big data: Issues, challenges, and techniques in business intelligence’, Advances in Intelligent Systems and Computing, 613-628. https://doi.org/10.1007/978-981-10-6620-7_59
- Wixom, B., Ariyachandra, T., Douglas, D., Goul, M., Gupta, B., Iyer, L., Kulkarni, U., Mooney, J. G., Phillips-Wren, G. i Turetken, O. (2014). ‘The current state of business intelligence in academia: The arrival of big data’, Communications of the Association for Information Systems, 34. https://doi.org/10.17705/1cais.03401
- Yafooz, W. M., Bakar, Z. B., Fahad, S. K. i M. Mithun, Ahamed. (2019). ‘Business intelligence through big data analytics, data mining and machine learning’, Data Management, Analytics and Innovation, 217-230. https://doi.org/10.1007/978-981-13-9364-8_17
- Zhang, Y., Liu, H., Kang, S.-C. i Al-Hussein, M. (2020). ‘Virtual reality applications for the built environment: Research trends and opportunities’, Automation in Construction, 118, 103311. https://doi.org/10.1016/j.autcon.2020.103311
- Zhang, Z., Wen, F., Sun, Z., Guo, X., He, T. i Lee, C. (2022). ‘Artificial intelligence enabled sensing technologies in the 5G/internet of things era: From virtual reality/augmented reality to the digital twin’, Advanced Intelligent Systems, 4(7). https://doi.org/10.1002/aisy.202100228