Introduction
Technology adoption has become a crucial aspect of the integration of educational institutions. The rapid increase in the adoption of information and communication technologies by educational institutions is an indication of the perceived benefits that the educational institutions have of these resources in aiding them to carry out academic and administrative roles (Yakubu & Dasuki, 2019). However, there continues to be a growing interest in how to improve the adoption and application of these technologies for teaching and learning (Suki & Suki, 2017).Traditional teaching approaches such as lecturing, tutorials and mentoring are some of the principal approaches used for teaching and learning in universities. However, more universities are now investing massively in developing learning technologies (Mahdizadeh, Biemans & Mulder, 2008). Personal computers, the internet as well as other technology-based learning tools are now common resources used in the universities, as academics and students continue to use them to communicate, collaborate and carry out research in a system generally referred to as a computer-assisted learning, virtual learning, electronic or e-learning (Ozkan, Koseler & Baykal, 2009). E-learning has become a necessity to meet the challenges posed by the development of information technology and the propensity for increased access to knowledge. There is much examination of the effects of communication and information technology (IT) on learning. Creative and innovative strategies are now being sought for improvement of a higher rate of learners’ success and achievement in higher institutions of learning as well as the goal of creating a tailored learning environment where in learners are able to pursue continuous learning in a motivated manner with the use of various technology or system.
Venkatesh et al. (2003) proposed the Unified Theory of Acceptance and Use of Technology (UTAUT) to explain the usage and the behavioural intention to use technology. In the model, they proposed and identified four direct determinants of behavioural intention and use of technology, i.e. performance expectancy, effort expectancy, social influence and facilitating conditions. Suki and Suki (2017) report that the model has been scrutinized in wide-ranging educational environments such as virtual learning technologies (Šumak, Polancic & Hericko, 2010), cloud-based virtual learning environments (Hew & Kadir, 2016), desktop web-conferencing (Lakhal & Khechine, 2016), interactive whiteboards (Šumak & Šorgo, 2016 and Tosuntaş, Karadağ & Orhan, 2015), classroom instruction (Ifenthaler & Schweinbenz, 2013) and Moodle learning management system (Hsu, 2012 and Tarhini et al. 2017). Although previous studies have documented the factors that stimulate and impede students from reaping the benefits of e-learning, there is little known about the factors that influence the adoption of e-learning in HEIs in developing countries especially from a student’s perspective, It is, therefore, important to note that many of these studies have focused on the developed country context, while the few that have been centred on developing countries have concentrated on the provision of the infrastructure and not on how the technology has been adopted (Macharia, 2011). Hence, examining e-learning adoption becomes a critical priority for HEIs. Thus, the propositions of the Unified theory of Acceptance and Use of Technology UTAUT model have been severally tested in western and developed nation contexts, but there are inadequate empirical validations of the propositions of the UTAUT model in the non-western context as well as in countries categorized as developing nations. Therefore, this study would determine the direct effects of the four determinants of e-learning adoption on the behavioural intention to use e-learning from students’ perspectives. Furthermore, the study would also examine the mediating effect of students’ behavioural intention to use e-learning in the relationship between the four determinant of e-learning adoption and the actual use of e-learning.
Literature Review
The Unified Theory of Acceptance and Use of Technology Model (UTAUT)
The Unified Theory of Acceptance and Use of Technology (UTAUT) concept attempts to clarify the intention of using information systems follow-up use behaviour. The theory believes that the performance of key structures; expected value, expected workload, social inspiration and favourable environments are all through to the elements of purpose and use performance of information systems (Venkatesh et al. 2003). Venkatesh et al. (2003) proposed that sex, age, involvement and age voluntary procedure mitigate the influence of the four key structures on use intentions and performance.
Performance expectancy, according to Venkatesh et al. (2003), is defined as “the degree to which an individual believes that using the system will help him or her attain gains in job performance” (p. 447). Venkatesh et al. (2003) assert that performance expectancy is the strongest predictor of the behavioural intention. Several scholars have found this to be valid in a certain research context, while others have also found other constructs to be the strongest determinants of behavioural intention in other contexts. For example, Šumak and Šorgo (2016) reported that performance expectancy has a positive and significant effect on the behavioural intentions of teachers to use interactive whiteboards. Alternatively, Raman et al. (2014) reported that effort expectancy is the strongest predictor of behavioural intention.
Effort expectancy is defined as the ease associated with the use of a system (Venkatesh et al. 2003). It is also a strong predictor of the behavioural intention at the initial stage of using the system. According to Yakubu and Dasuki (2019), effort expectancy becomes less significant with the increased use of the system as the users will have been better accustomed to using the system. Prior studies have shown that effort expectancy positively affects the behavioural intentions to use a system as well as the actual usage of a system or technology (Suki & Suki, 2017; Šumak et al., 2010; Tosuntaş et al., 2015; Venkatesh et al., 2003 and Yakubu & Dasuki, 2019).
Social influence is described as an individual’s perception that those who are important to him/her believe that he/she should use the system (Venkatesh et al. 2003). In other words, social influence is the extent to which an individual perceives that important social groups or elements believe that such individual should use the new system. In this study, social influence refers to the influence and support from people such as friends, peers, social cycle, educators, management of universities as well as academic administrators to use e-learning as part of their learning tools. Yakubu and Dasuki (2019) assert that social influence had a direct effect on students’ behavioural intentions with voluntariness of use moderating the relationship between social influence and behavioural intention to use the system.
Facilitating conditions: is defined as the degree to which an individual believes that the organizational and technical infrastructure exist to support the use of a system (Venkatesh et al. 2003). Hence, in this study, facilitating conditions is regarded as the accessibility of an appropriate learning environment and infrastructure within the university that can foster the use of the considered technologies. Such conditions include individuals’ knowledge and skills, and an environment that stimulates and supports students’ willingness to use e-learning. In the original UTAUT model, Venkatesh et al. (2003) report that facilitating conditions was one of the major determinants of the behavioural intentions.
The UTAUT framework postulates that performance expectancy, effort expectancy, social influence and facilitating conditions influence individuals’ behavioural intentions to use a technology (Venkatesh et al. 2003). According to Venkatesh et al. (2003), behavioural intention is described as an individual’s likelihood to participate in a specific behaviour. In other words, it is a person’s subjective probability that he or she will perform the behaviour in question (Venkatesh et al. 2003). In the context of this study, behavioural intention was conceptualized as the subjective probability that students will use e-learning as part of their learning. Yakubu and Dasuki (2019) opine that behavioural intention gauges the strength of an individual’s commitment to engage in a particular behaviour.
Framework of the study
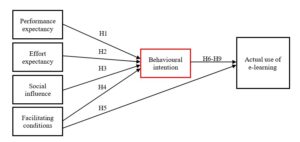
Figure 1: Framework of the study
H1: performance expectancy has a positive effect on students’ behavioural intention to use e-learning.
H2: effort expectancy has a positive effect on students’ behavioural intention to use e-learning.
H3: Social influence has a positive effect on students’ behavioural intention to use e-learning.
H4: facilitating conditions has a positive effect on students’ behavioural intention to use e-learning.
H5: facilitating conditions has a negative effect on students’ actual usage of e-learning, if age and experience are not moderated.
H7: behavioural intention mediates the relationship between Performance expectancy and the actual use of e-learning.
H7: behavioural intention mediates the relationship between effort expectancy and the actual use of e-learning.
H8: behavioural intention mediates the relationship between social influence and the actual use of e-learning.
H9: behavioural intention mediates the relationship between facilitating conditions and the actual use of e-learning
Methodology
The propositions of the UTAUT model have been severally tested in western and developed nation contexts (Salloum & Shaalan, 2018 and Suwaidi, 2019). However, there are inadequate empirical validations of the propositions of the UTAUT model in a non-western context as well as in countries categorized as developing nations. Hence, the purpose of this study was to examine the direct and indirect effects of the four determinants of technology adoption on students’ behavioural intentions and the actual use of e-learning in HEIs in the UAE. Using a quantitative approach and a survey research design, data was obtained from a sample of 406 students selected from the higher colleges of technology in the UAE. Therefore, Smart-PLS 3.0 Structural Equation Modelling software was used to explore statistical relationships among the items of each factor and between the factors of independent and dependent variables.
Results
Convergent Validity And Reliability
Convergent validity is the degree to which the indicators of a specific construct converge or share a high proportion of the variance for that construct (Hair, Ringle & Sarstedt, 2011). In other words, it refers to the level by which a measure positively relates to other measures within the same construct, i.e. the degree to which a latent construct explains the variance of its indicators. According to Hair et al. (2014a), convergent validity can be assessed using the factor loadings, composite reliability (CR) and the Average Variance Extracted (AVE). To achieve convergent validity, each construct should have an AVE of ≥ 0.50, and the factor loadings should be preferably greater than 0.70. Although Byrne (2013) suggest that factor loadings of 0.50 are acceptable if the summations of the loading results in high loading scores contributing to AVE scores of 0.50 or greater. Table 1 shows the factor loadings and AVE of each of the constructs in the study. All measured constructs had AVE scores greater than the threshold value of 0.50 and factor loadings ranging from 0.565 to 0.875 consecutively, indicating that convergent validity had been established (Byrne, 2013 and Gefen, Straub & Boudreau, 2000).
Table 1: Convergent Validity and Reliability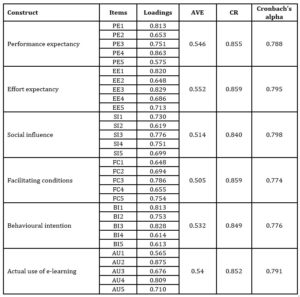
Reliability is the extent to which an instrument is free from random errors and the extent to which such instrument produces consistent results if repeated in other settings or contexts (David & Sutton, 2011 and Pallant, 2011b). This implies that reliability and error are related, in the sense that the higher the error, the less reliable an instrument is and vice versa. In this study, the internal consistency reliability test and composite reliability were used to determine the reliability of the scales. Table 1 shows the composite reliability scores and Cronbach alpha values for each of the measured variables. All constructs had composite reliability ranging from 0.840 to 0.859 and Cronbach alpha values ranging from 0.774 to 0.798, respectively. According to George and Mallery (2003), scales with Cronbach’s Alpha value are considered poor when the alpha value is < 0.60, fairly reliable when the alpha value is between 0.60 to 0.69, good when it falls between 0.70 to 0.79 and excellent when the value is 0.80 and above. While composite reliability scores of 0.6 and above is considered acceptable, especially by (Bagozzi & Yi, 1988 and Hair et al. 2014b). Hence, given that the Cronbach alpha reliability scores and composite reliability scores exceeded the minimum threshold values as reported by experts in the field, then the reliability of the scales have been established.
Discriminant Validity
Discriminant validity, according to Ramayah et al. (2016), is the degree to which indicators differentiate across constructs or measures distinct concepts by examining the correlations between the measures of potentially overlapping constructs. The significance of conducting a discriminant validity test is to assess whether the constructs are distinct from others in the structural models or not. Gefen et al. (2000) and Fornell and Larcker (1981) explained that discriminant validity is assessed by examining the correlation between the construct. Discriminant validity is traditionally assessed using three known criteria namely: Fornell and Larker criterion, the cross-loadings criterion and the Heterotrait Monotrait ratio (Hair et al., 2014a and Ramayah et al. 2016). Hence, discriminant validity, in this study, was assessed using all three criteria. Hence, Discriminant validity is established from the Fornell and Lacker Criterion since the square root of the AVEs for the constructs actual use, behavioural intention, effort expectancy, facilitating conditions, performance expectancy and social influence is higher than their respective highest correlation as shown in each column in Table 2 below.
Table2: Discriminant validity using Fornell and Lacker Criterion
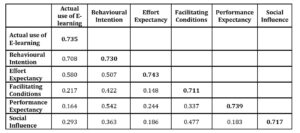
Similarly, the factor loading criterion was also used to assess discriminant validity. Table 2 shows that all indicator loadings loaded highly on their own respective constructs (as indicated by bold text) compared to other constructs, indicating that there was no issue of high cross-loading between one another. Hence, discriminant validity is established.
Table 3: Discriminant validity using the HTMT ratio criterion
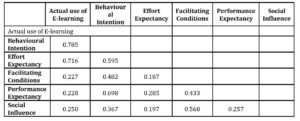
Lastly, the Heterotrait Monotrait ratio criterion was also used to establish discriminant validity. Table 3 shows the HTMT ratios for the constructs. The highest HTMT ratio was between the behavioural intention and the actual use of e-learning with a value of 0.785. Discriminant validity is established since all the ratios were below the threshold values of HTMT.85 and HTMT .90 as indicated by (Kline, 2015) and (Gold, Malhotra & Segars, 2001) respectively.
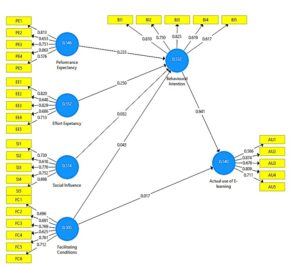
Figure 2: Research structural model
Table 4: Significance test for the Structural model path coefficient, t-value and p-values
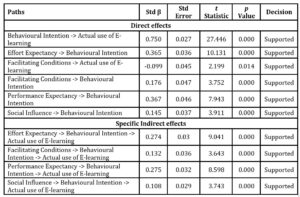
From Table 4, it can be seen that there are six direct path coefficients of which all were significant with t-values exceeding the t-critical value of 1.96 and p-values value less than 0.05. Three of the direct effects had a strong positive relationship with the exception of the relationship between facilitating conditions and the actual use of e-learning, which had a negative relationship. This may be due to the exclusion of the moderating variables; age and experience from the original UTAUT model in this study. Detailed discussions regarding this will be provided in a subsequent section. Similarly, there were four indirect effects (i.e. mediation) that were tested in the study. All four mediation analyses were significant, implying that the quality of the structural model is good.
Discussion And Conclusions
Findings from the analysis revealed that performance expectancy (β = 0.367, p <0.05) had a positive effect on HCT’s students’ behavioural intention to use e-learning. Venkatesh et al. (2003) described performance expectancy as the extent to which an individual believes that using a system will help him or her attain gains in the job performance. In the context of this study, performance expectancy was described as a student’s belief that using e-learning will be beneficial and interesting in achieving high performance in learning. Since findings from the data analysis in this study revealed that performance expectancy was positively correlated with the behavioural intention to use e-learning as evident in the strong positive path coefficient, it then implies that as students perceive that using e-learning will help improve their performances in learning, the more students intend to adopt it. Therefore, positive perceptions of the gains of e-learning will ultimately result in the intention to adopt it, whereas a negative perception of the gains of e-learning will result in students’ intent to reject it as part of their learning activities. Thus, this finding is consistent with those reported in the extant literature (Alsumait & Al-Musawi, 2013; Chauhan & Jaiswal, 2016; Lowe & Boucheix, 2016; Luzón & Letón, 2015; Stebner et al., 2017; Suki & Suki, 2010, 2017 and Tosuntaş et al. 2015). Interestingly, in the adapted UTAUT model utilized in this study, the role of moderating variables such as age, gender, experience and voluntariness of use was not examined. However, a significant and positive effect between performance expectancy and students’ behavioural intention to use e-learning was still obtained. This, therefore, implies that in as much as age and gender moderates the relationship between performance expectancy and behavioural intention in the original UTAUT model; in an e-learning context, an unmoderated relationship between the latent variable (performance expectancy) and students’ behavioural intention to use e-learning is still supported as evident in the results of this study. Hence, the hypothesis H1 was supported.
Likewise, (H2) effort expectancy has a positive effect on student’s behavioural intention to use e-learning. The hypothesis was centred on testing students’ perception of how easy it was to use e-learning technology and how this perception influenced their behavioural intention to use the technology. Findings from the study revealed that there was a positive linkage between effort expectancy (β = 0.365, p <0.05) and students’ behavioural intention to use e-learning. Venkatesh et al. (2003) described effort expectancy as the “degree of ease” associated with the use of a system. In this study, effort expectancy was conceptualized as students’ belief that using e-learning in facilitating their learning will be easy for them, i.e. it will require little effort. This, therefore, indicates that effort expectancy is a strong determinant of the behavioural intention to adopt or reject e-learning. This finding is consistent with those of the original UTAUT model by (Venkatesh et al. 2003), as well as most of the studies reported in the e-learning literature (Dečman, 2015; Raman et al., 2014; Tosuntaş et al., 2015 and Yakubu & Dasuki, 2019). For instance, Yakubu and Dasuki (2019) in a study carried out in Nigeria, also reported that effort expectancy positively influenced students’ behavioural intention to use e-learning. Effort expectancy, in this study, was the second strongest determinants of the behavioural intention after performance expectancy. Therefore, it can be said that as students perceive that the e-learning system is easy to use and requires little of minimal effort to operate, then they will be more inclined to adopt e-learning as part of their learning tools.
In addition, (H3) Social influence has a positive effect on students’ behavioural intention to use e-learning. This hypothesis was concerned with the impact of the social influence on students’ behavioural intention to adopt e-learning. Social influence is a non-system specific behaviour. Findings from the data analysis revealed that there was a positive correlation between social influence (β = 0.145, p <0.05) and students’ behavioural intention to use e-learning. Venkatesh et al. (2003) described social influence as the degree to which individuals believe that significant others expect the individual to use the system. In the context of this study, social influence was described as the extent in which students perceive that significant others (i.e. friends, social cycle, university management, academic administrators, educators etc.) expect them to use the e-learning system. This, therefore, implies that social influence is a moderate determinant of the behavioural intention to use e-learning.
Hypothesis (H4) is focused on testing whether the provision of an appropriate learning environment, as well as e-learning knowledge and skills, would influence students’ behavioural intention to use e-learning. Findings from the data analysis revealed that there was a positive association between facilitating conditions (β = 0.176, p <0.05) and students’ behavioural intention to use e-learning, although to a lesser degree than performance expectancy and effort expectancy as evident in the path coefficient obtained.
Facilitating conditions is defined as the degree to which an individual believes that the organizational and technical infrastructure exists to support the use of a system (Venkatesh et al. 2003). In this study, facilitating conditions is regarded as the accessibility to an appropriate learning environment and infrastructure within the university that can foster the use of the considered technology. These conditions include an access to the knowledge and skills required to operate the technology, as well as an environment that stimulates and supports students’ willingness to use the technology (i.e. e-learning). Hence, the results show that facilitating conditions is a moderate determinant of the behavioural intention to use e-learning.
This finding is consistent with those reported in the literature (Mahande & Malago 2019; Raman et al., 2014 and Suki & Suki, 2010). For instance, Mahande and Malago (2019) reported that facilitating conditions positively and significantly affect the behavioural intention to use e-learning. This implies that the support that students receive from peers, their social cycle, university administrators and educators alike influences their behavioural intention to adopt e-learning. Conversely, this finding is in contrast to what was reported by Venkatesh et al. (2003), as they reported that facilitating conditions had a positive effect on the use and not on the behavioural intention to use a system. Thus, this contradicts the findings of this study, as data from this study revealed that facilitating conditions positively influence the behaviour to use. Venkatesh et al. (2003) described the behavioural intention as a person’s subjective probability that he or she will perform the behaviour in question. Evidently, the behaviour to use was conceptualized as the subjective probability that students will use e-learning as part of their learning. Hence, when students are provided with the needed resources to use a system (in this case e-learning), the knowledge and skills to operate e-learning and support from their social cycle including peers and educators, data from this study show that such students will be more inclined to use that system. Hence, this study shows that facilitating conditions does not automatically translate into the actual use of e-learning.
Additionally, (H5) facilitating conditions has a negative effect on students’ actual usage of e-learning if age and experience are not moderated. Given that in the original UTAUT model, facilitating conditions through the moderating role of age and experience had a positive and significant effect on the use of a system, the researchers thus hypothesized that if the provided resources, earning environment and support are not specifically tailored to the actual age and experience of the learners, it may deter their actual use of the system. This implies that if age and experience are not moderated, there may be a negative effect of facilitating conditions on the actual use of e-learning. Hence, hypothesis (H5) was tested to determine if the assumptions held by the researcher were valid and supported by the data. Findings from the data analysis support the hypothesis (H5) that there was a weak and a negatively significant effect of facilitating conditions (β = -0.099, p <0.05) on the actual use of e-learning. This finding is consistent with several studies in the literature (Fang, 2014; Isaac et al., 2019 and Wirba Singeh et al. 2013). Isaac et al. (2019) reported in their study that facilitating conditions does not influence the actual usage of the internet. The authors explained that this might be because providing individuals with the hardware and software to use the internet may not be necessary since, in this information age, individuals access the internet through their own laptops, smartphones or similar devices. Regarding the finding, the researcher takes a different direction in explaining the negative effect of facilitating conditions on students’ actual use of e-learning. Facilitating conditions as described earlier is the degree to which an individual believes that the organizational and technical infrastructure exists to support the use of a system (Venkatesh et al. 2003). In this study, facilitating conditions is regarded as the accessibility to an appropriate learning environment and infrastructure within the university that can foster the use of the technology considered. If students are provided with the needed resources to use a system (in this case e-learning), the knowledge and skills to operate e-learning, and support from their social cycle including peers and educators, while these resources are not tailored specifically to the age and experiences of these students, the result will be a decline to use such a system (i.e. e-learning). Therefore, the researcher believes that the absence of students’ age and experience to moderate the effect of facilitating conditions on the actual use of learning results in the negative relationship association as shown in the result from the data analysis. Hence, the hypothesis (H5) was supported.
Findings from the analysis revealed that the behavioural intention mediates the relationship between performance expectancy (β = 0.275, p < 0.05) and actual use of e-learning, effort expectancy (β = 0.274, p < 0.05) and actual use of e-learning, social influence (β = 0.108, p < 0.05) and actual use of e-learning, facilitating conditions (β = 0.132, p < 0.05) and e-learning. Together, all the latent variables explained about 50.5% of the variance in the behavioural intention with the adjusted R2 = 0.505, while the behavioural intention explained about 50.7% of the variance in actual use with the adjusted R2 = 0.507. This implies that hypotheses H6-H9 were supported by the data. This finding is consistent with the findings of several studies reported in the literature (Chauhan & Jaiswal, 2016; Isaac et al., 2019; Suki & Suki, 2017; Tosuntaş et al., 2015 and Yakubu & Dasuki, 2019). Therefore, it can be concluded that performance expectancy, effort expectancy, social influence and facilitating conditions influence students’ behavioural intention to use e-learning as these four dimensions explain 50.5% of the variance in the behavioural intention to use e-learning. Students’ behavioural intention to use learning, in turn, influences students’ actual use of e-learning as 50.7% of the variance in actual use was explained by the behavioural intention to use e-learning. Hence, in the context of this study, both the direct and indirect relationships hypothesized were supported by the data.
Research Contributions and Implications
The study was a confirmatory analysis of the UTAUT model in a developing country context (i.e. the UAE) specifically aimed at ascertaining the direct and mediating effect of four determinants of technology acceptance on students’ behavioural intention to use e-learning and their actual use of e-learning likewise. The researcher first sought to determine the direct effects of the four determinants of technology acceptance proposed in the UTAUT model (i.e. performance expectancy, effort expectancy, social influence and facilitating conditions) on students’ behavioural intention to use e-learning. Thereafter, the researcher examined the mediating effect of students’ behavioural intention to use e-learning in the relationship between performance expectancy, effort expectancy, social influence, facilitating conditions and actual use of learning.
Findings from the study showed that performance expectancy, effort expectancy, social influence and facilitating conditions had positive and significant effects on students’ behavioural intention to use e-learning. The uniqueness of this finding was that the model did not include the moderating variables; age, gender, experience and voluntariness of use as proposed in the original UTAUT model by (Venkatesh et al. 2003). Interestingly, it was also shown from the study that facilitating conditions had a significant but negative effect on students’ actual use of e-learning. This was explained to be because students’ age and experiences were not used as moderators to test the effect of facilitating conditions on students’ actual use of e-learning as in the original UTAUT model. The implication of this is that if the technical and operational infrastructure provided is not tailored to the appropriate age of the students, such that the provided support is meaningful and useable by such students, then the result would be of a negative effect, indicating that students would be deterred from actually using the technology if they don’t find the provided support meaningful and useful. Furthermore, if the support provided is not built on students’ past experiences, then the result would be of a negative effect on the actual use of the technology, in this case, e-learning. Finally, the revised model for e-learning adoption shows that behavioural intention mediates the effects between performance expectancy, effort expectancy, social influence and facilitating conditions and actual use of e-learning, indicating that students are more likely to adopt and use e-learning as part of their learning tools if they perceive that e-learning would be beneficial to them, easy to use, their peers and social cycle expect them to use the system, and that the right support is provided and tailored to their age and specific experiences.
Limitations of the Study
Findings from this study were established using a quantitative research approach, which uses numerical data to make an inference from a sample to a population. While quantitative approaches are in themselves important research methodologies, they are limited in terms of providing deeper insights and understanding regarding the variables studied. Therefore, future studies can use mixed-methodology approaches in weighing the perceptions, ideas and views of students and faculty members alike in understating the factors that affect e-learning adoption. The instruments used in the study were self-reported instruments that measured students’ perceptions of the variables of the study. Self-reported instruments could be argued to be proxy measures of perception and may pose threats to the internal validity of the study. Perhaps a cross-validated instrument could be used in future studies where two categories of the respondent are surveyed to provide perceptions regarding the variables measured. Another approach could be the use of experimental approaches to study the performance of participants regarding the measured variables.
Recommendations for Further Research
Future studies can include more variables such as culture, language and other contextual variables to study the effects of the determinants of technology adoption on students’ use of e-learning systems. This will be particularly interesting if the role of culture and language can be explored in e-learning adoption. Such a study would lead to the development of a more comprehensive model for e-learning adoption that highlights the role of contextual variables such as culture and language. The moderating roles of age, gender, experience and voluntariness of use were not intentionally explored in this study as the researcher was only interested in the direct and mediating relationship between the variables. Therefore, examining the roles of the d=moderators can help understand the intricacies of the effects of the independent variables on the dependent variables.
Conclusion
In conclusion, this study has addressed a significant gap in e-learning system adoption the in UAE. This is achieved by examining the direct and mediating effects of performance expectancy, effort expectancy, social influence, facilitating conditions, behavioural intentions and actual use. Nine research hypotheses were tested in a cross-sectional survey to help provide insights regarding the measured variables of the study. As a researcher, the findings of this study have increased my understanding of the nature of e-leading adoption in a developing country context. As a teacher, the findings of this study will help shape my practice in terms of integrating e-learning into my teaching and learning activities, since the study has revealed the major determinants of e-learning adoption from a student’s perspective. It hence concluded that in the higher education context, performance expectancy, effort expectancy, social influence and facilitating conditions all have a positive effect on students’ behavioural intention to use e-learning which in turn affects their actual use of e-learning. Furthermore, the facilitation conditions (i.e. technical and operational support provided must be specifically tailored to the age and experience of the students to have a positive effect on students’ actual use of the technology, otherwise if the support provided is not considered relevant and suited to students’ age and experience, then students would be deterred from using the technology.
(adsbygoogle = window.adsbygoogle || []).push({});
References
- Alhebsi, A., Pettaway, L., & Waller, L. (2015). A history of education in the United Arab Emirates and trucial sheikdoms. The Global eLearning Journal, 4(1), 1-6.
- Alsumait, A., & Al-Musawi, Z. S. (2013). Creative and Innovative E-Learning Using Interactive Storytelling. International Journal of Pervasive Computing and Communications, 9(3), 209-226.
- Al Shehhi, R. H. R., & Azam, S. F. (2019). Measuring The Mediating Role Of Project Management Between Total Quality Management And Organisational Success In Sultanate Of Oman. European Journal of Human Resource Management Studies.
- Bagozzi, R. P., & Yi, Y. (1988). On the Evaluation of Structural Equation Models. Journal of the academy of marketing science, 16(1), 74-94.
- Blaikie, P., Cannon, T., Davis, I., & Wisner, B. (2004). At risk: natural hazards, people’s vulnerability and disasters. Routledge.
- Boateng, R., Mbrokoh, A. S., Boateng, L., Senyo, P. K., & Ansong, E. (2016). Determinants of E-Learning Adoption among Students of Developing Countries. The International Journal of Information and Learning Technology, 33(4), 248-262.
- Byrne, B. M. (2013). Structural Equation Modeling with Mplus: Basic Concepts, Applications, and Programming: Routledge.
- Chang, C. C., Tseng, K. H. and Tseng, J. S. (2011). Is Single or Dual Channel with Different English Proficiencies Better for English Listening Comprehension, Cognitive Load and Attitude in Ubiquitous Learning Environment? Computers and Education, 57, 2313-2321.
- Chauhan, S., & Jaiswal, M. (2016). Determinants of Acceptance of Erp Software Training in Business Schools: Empirical Investigation Using Utaut Model. The International Journal of Management Education, 14(3), 248-262.
- Chet Hosmer, Carlton Jeff coat, Matthew Davis, Thomas Mc Gibbon, 2011, Use of Mobile Technology for Information Collection and Dissemination, Data &Analysis Center for Software, UK.
- Crompton, H. (2013). A historical overview of mobile learning: Toward learner-centered education. In Z. L. Berge & L. Y. Muilenburg (Eds.), Handbook of mobile learning (pp. 3–14). Florence, KY: Routledge.
- Zhang,L. Zhou, R.O. Briggs &J.F. Jr. Nunamaker, (2010). Instructional video in m-learning: Assessing the impact of interactive video on learning effectiveness, Journal of Information &Management, Vol. 43 No (20), pp. 15–27.
- David, M., & Sutton, C. D. (2011). Social Research: An Introduction: Sage.
- Davis, F. D. (1989). Perceived Usefulness, Perceived Ease of Use, and User Acceptance of Information Technology. MIS quarterly, 319-340.
- Davis, L. D., Bagozzi, R. P. and Warshaw, P. R. (1989). User acceptance of computer technology: a comparison of two theoretical models. Management Science, 35, 8, 982–1002.
- Davis, F. D., Bagozzi, R. P. and Warshaw, P. R. (1992). Extrinsic and Intrinsic Motivation to Use Computers in the Workplace. Journal of Applied Social Psychology, 22, 14, 1111–1132.
- Davis, J. S., Garcia, G. D., Jouria, J. M., Wyckoff, M. M., Alsafran, S., Graygo, J. M., Withum, K. F. and Schulman, C. I. (2013). Identifying Pitfalls in Chest Tube Insertion: Improving Teaching and Performance. Journal of Surgical Education, 70, 334-339.
- Hew, T.-S., & Kadir, S. L. S. A. (2016). Behavioural Intention in Cloud-Based Vle: An Extension to Channel Expansion Theory. Computers in human behavior, 64, 9-20.
- Horton, W. (2011). E-learning by design. John Wiley & Sons.
- Horton, W. K. (2012). Designing web-based training: How to teach anyone anything anywhere anytime (Vol. 1). New York, NY: Wiley.
- Hsu, Y. C. and Ching, Y. H. (2012). Mobile Microblogging: Using Twitter and Mobile Devices in an Online Course to Promote Learning in Authentic Contexts. International Review of Research in Open and Distance Learning, 13, 211-227.
- Hsu, H.-H. (2012). The Acceptance of Moodle: An Empirical Study Based on Utaut. Creative Education, 3, 44.
- Ifenthaler, D., & Schweinbenz, V. (2013). The Acceptance of Tablet-Pcs in Classroom Instruction: The Teachers’ Perspectives. Computers in human behavior, 29(3), 525-534.
- Isaac, O., Abdullah, Z., Aldholay, A. H., & Ameen, A. A. (2019). Antecedents and Outcomes of Internet Usage within Organisations in Yemen: An Extension of the Unified Theory of Acceptance and Use of Technology (Utaut) Model. Asia Pacific Management Review.
- Kline, R. B. (2015). Principles and Practice of Structural Equation Modeling: Guilford publications.
- Lakhal, S., & Khechine, H. (2016). Student Intention to Use Desktop Web-Conferencing According to Course Delivery Modes in Higher Education. The International Journal of Management Education, 14(2), 146-160.
- Levis, P., Lee, N., Welsh, M., & Culler, D. (2012). TOSSIM: Accurate and scalable simulation of entire Tinyos applications. In Proceedings of the 1st international conference on Embedded networked sensor systems (pp. 126-137). ACM.
- Lowe, R. K., & Boucheix, J.-M. (2016). Principled Animation Design Improves Comprehension of Complex Dynamics. Learning and Instruction, 45, 72-84.
- Luzón, J. M., & Letón, E. (2015). Use of Animated Text to Improve the Learning of Basic Mathematics. Computers & Education, 88, 119-128.
- Macharia, A. W. (2011). Towards Adoption of Electronic Learning: An Empirical Investigation of Faculty Behavioral Intentions. Capella University.
- Mahande, R. D., & Malago, J. D. (2019). An E-Learning Acceptance Evaluation through Utaut Model in a Postgraduate Program. Journal of educators online, 16(2).
- Mahdizadeh, H., Biemans, H., & Mulder, M. (2008). Determining Factors of the Use of E-Learning Environments by University Teachers. Computers & Education, 51(1), 142-154.
- Memon, A. H., & Rahman, I. A. (2013). Analysis of Cost Overrun Factors for Small Scale Construction Projects in Malaysia Using Pls-Sem Method. Modern applied science, 7(8), 78.
- MostafaAl-Emran, Hatem M. Elsherif, Khaled Shaalan, 2016, Investigatingattitudes towards the use of mobile learning in higher education, Elsiver Journal, Vol (56) No. (93)
- Ozkan, S., Koseler, R., & Baykal, N. (2009). Evaluating Learning Management Systems: Adoption of Hexagonal E-Learning Assessment Model in Higher Education. Transforming Government: People, Process and Policy, 3(2), 111-130.
- Pallant, J. (2011a). Spss Survival Manual 4th Edition: A Step by Step Guide to Data Analysis Using Spss Version 18. Maidenhead, Berkshire: Open University Press. Retrieved on from.
- Raman, A., Don, Y., Khalid, R., & Rizuan, M. (2014). Usage of Learning Management System (Moodle) among Postgraduate Students: Utaut Model. Asian Social Science, 10(14), 186.
- Ramayah, T., Cheah, J., Chuah, F., Ting, H., & Memon, M. A. (2016). Partial Least Squares Structural Equation Modeling (Pls-Sem) Using Smartpls 3.0: An Updated and Practical Guide to Statistical Analysis. In: Pearson Singapore.
- Ruohotie, P. (2012). Professional growth and development. In International handbook of educational leadership and administration (pp. 419-445). Springer, Dordrecht.
- Salloum, S. A., Mhamdi, C., Al Kurdi, B., & Shaalan, K. (2018). Factors affecting the Adoption and Meaningful Use of Social Media: A Structural Equation Modeling Approach. International Journal of Information Technology, 2(3), 96-109.
- Smith, M. E., & Farah, M. J. (2011). Are prescription stimulants “smart pills”? The epidemiology and cognitive neuroscience of prescription stimulant use by normal healthy individuals. Psychological bulletin, 137(5), 717.
- Stebner, F., Kühl, T., Höffler, T. N., Wirth, J., & Ayres, P. (2017). The Role of Process Information in Narrations While Learning with Animations and Static Pictures. Computers & Education, 104, 34-48.
- Strijbos, J. W., Martens, R. L., &Jochems, W. M. (2009). Designing for interaction: Six steps to designing computer-supported group-based learning. Computers & Education, 42(4), 403-424Suki, N. M., & Suki, N. M. (2010). Examining Students’attitudes to the Mobile Phone as an Educational Tool. Journal of Education Research, 4(1).
- Suki, N. M., & Suki, N. M. (2017). Determining Students’ Behavioural Intention to Use Animation and Storytelling Applying the Utaut Model: The Moderating Roles of Gender and Experience Level. The International Journal of Management Education, 15(3), 528-538.
- Šumak, B., Polancic, G., & Hericko, M. (2010). An Empirical Study of Virtual Learning Environment Adoption Using Utaut. Paper presented at the 2010 Second international conference on mobile, hybrid, and on-line learning.
- Šumak, B., & Šorgo, A. (2016). The Acceptance and Use of Interactive Whiteboards among Teachers: Differences in Utaut Determinants between Pre-and Post-Adopters. Computers in human behavior, 64, 602-620.
- Tarhini, A., Masa’deh, R. e., Al-Busaidi, K. A., Mohammed, A. B., & Maqableh, M. (2017). Factors Influencing Students’ Adoption of E-Learning: A Structural Equation Modeling Approach. Journal of International Education in Business, 10(2), 164-182.
- Tavangarian, D., Leypold, M.E., Nölting, K., Röser, M. & Voigt, D. (2010). Is eLearning the Solution for Individual Learning? Electronic Journal of e-Learning, Vol. 2 (2), pp. 273-280.
- Tenenhaus, M., Amato, S., & Esposito Vinzi, V. (2004). A Global Goodness-of-Fit Index for Pls Structural Equation Modelling. Paper presented at the Proceedings of the XLII SIS scientific meeting.
- Tosuntaş, Ş. B., Karadağ, E., & Orhan, S. (2015). The Factors Affecting Acceptance and Use of Interactive Whiteboard within the Scope of Fatih Project: A Structural Equation Model Based on the Unified Theory of Acceptance and Use of Technology. Computers & Education, 81, 169-178.
- Van Raaij, E. M., & Schepers, J. J. (2008). The Acceptance and Use of a Virtual Learning Environment in China. Computers & Education, 50(3), 838-852.
- Venkatesh, V., Morris, M. G., Davis, G. B. and Davis, F. D. (2003). User acceptance of information technology: toward a unified view. MIS Quarterly, 27, 3, 425–478.
- Wirba Singeh, F., Abrizah, A., & Harun Abdul Karim, N. (2013). Malaysian Authors’ Acceptance to Self-Archive in Institutional Repositories: Towards a Unified View. The Electronic Library, 31(2), 188-207.
- Yakubu, M. N., & Dasuki, S. I. (2019). Factors Affecting the Adoption of E-Learning Technologies among Higher Education Students in Nigeria: A Structural Equation Modelling Approach. Information Development, 35(3), 492-502.