Introduction
The situation of the pandemic in the whole world caused by COVID-19 coronavirus disease has directed the whole population towards a situation of quarantine, which has triggered a rise in stress. Many universities, faced with this situation, had to alter to the deviations due to the pandemic as well as to face a new educational model in teaching namely e-learning, for which not all faculty members and students were prepared. At this time of change, an increase in stress has developed among people due to the uncertainty because of the virus.
Due to this crisis, universities in Egypt had to suspend the physical or offline educational activity. As a result, the digital delivery of education was considered, and it was necessary to integrate technology with learning online. Furthermore, the situation developed by COVID-19 had negative impact on students as the university context was stressful. In addition, the period of the pandemic was full of uncertainty and unawareness about the disease which was really high. This pandemic was and is still of a great worry for the Egyptian population and specifically for the students as they are faced by many challenges during their learning process.
E-learning is defined as the learning tool delivered via computers for the intention to develop education (Mayer, 2003), that has expanded quickly over last years with the upgrades in technology and its integration in the educational curricula. (Smart and Cappel, 2006). E-learning is essential to develop good calibers well educated, as well as the rapid need of society for long term learning that is delivered in an appropriate form. Learning online can take many forms from fully online, blended or web-assisted, no matter what the delivery technique of learning; however, a number of different tools are available at the students’ and faculty members’ hands. (Alshehri, 2017). In fact, online learning is a tool that is globally used for learning with standards and it is not required from the learner or the student to attend the class physically and is suitable for students who are interested in studying while they have other commitments. (El-Ebiary et al., 2016).
Students liked this way of learning online and sometimes it appeared to be more effective than face to face teaching. While students have an online meeting with their instructors, the option of sharing their devices’ screens may help the students to sense less feeling when asking for help compared to a physical class within groups. The students may select a quicker way to ask for help such as posting their questions on the online chat. This technique of asking questions may allow the student to feel more comfort than raising up their hands in front of their colleagues in a computer lab. Similar recent studies have reported (Davison, 2020) that student interaction was motivated through the use of online chat functionality. (Dwivedi et al., 2020).
In light of the dramatic increase in the use of online courses, in higher education especially after the pandemic of COVID-19, the current study deals with identifying the impact of academic self-efficacy on both students’ satisfaction and commitment in an online learning environment. It also investigates the impact of the moderating variables namely digital nudging and gender on the students’ satisfaction and commitment. Some studies tackled the effect of self-efficacy on satisfaction and commitment but without considering the moderating effect of gender and digital nudging. Therefore, the two moderating variables will be tested with the effect of the other variables. Accordingly, a preliminary model will be developed as shown in Figure 1.
Literature Review
The relationship between self-efficacy, satisfaction, and commitment
There are several studies that mentioned the relationships between self-efficacy, satisfaction, and commitment. The theory of self-efficacy can be well-defined as a person’s beliefs that he or she are able of attaining certain goals and performing specific activities (Bandura, 2002); (Hefferon, 2011); (Luszczynska et al., 2005); (Robbins et al., 2017); (Schermerhorn et al., 2011); (Demir, 2020). Adewale et al. (2017)) mentioned that self-efficacy is the people’s decision about their ability to attain a specific task or duty (Almutairi, 2020).
Moreover, a person’s satisfaction is defined as the extent to which an individual has different feelings about their colleagues and the work settings (Schermerhorn et al., 2011). In addition, organizational commitment is defined as the extent a person is devoted to his/her organization and its goals (Schermerhorn et al., 2011). Organizational commitment theory has three types named as affective commitment, normative commitment, and continuous commitment (Allen and Meyer, 1990, Allen and Meyer, 1991). Affective commitment includes confident feelings such as a people attached emotionally with their organization. Continuance commitment is described as the degree of commitment which is concerned with the loss of leaving the organization. Finally, normative commitment is defined as the tenacity to remain in an organization because of the feeling of obligation (Allen and Meyer, 1990) (Allen and Meyer, 1996); (Allen and Meyer, 1991)); (Demir, 2020).
Zeb and Nawaz. (2016) found that self-efficacy has a positive relation with the organizational commitment of members of academic staff in universities. Similarly, self-efficacy is interrelated with the organizational commitment of employees in small scale industries in China (LiLin and Shiqian, 2018); (Almutairi, 2020).
In an academic context, understanding faculty members’ behaviors involves more consideration as the performance of universities depends upon their members, which ought to be commitment and satisfaction (Tsui and Cheng, 1999). Grace and Khalsa (2003) recognized professional development and salary packages as the satisfaction factors that lead to commitment, while, on the other hand, Lee and Miller (2001) and VanderPutten and Wimsatt (1999) explained university funding, income, locus of control, employment options and supervision as the factors of satisfaction that are to be considered in faculty positions at any academic institution. A creative university environment results into academic members’ better satisfaction (Anari, 2012), increases their commitment and develops the output of the universities (Sabri et al., 2011); (Bashir and Ganai, 2019).
Many studies have supported the significant impact of self-efficacy beliefs on students’ well-being and course satisfaction (Pajares and Schunk, 2001); (DeWitz and Walsh, 2002). High self-efficacy students tend to experience more satisfaction than the students with low self-efficacy. Self-efficacy as a concept is considered as being domain-specific (Bandura, 2006). But some researchers have proposed a comprehensive view of self-efficacy which states to a person’s confidence in the ability of completing tasks across demanding or novel situations (Schwarzer and Jerusalem, 1995). The comprehensive view of self-efficacy could be an applicable mediator for students’ overall satisfaction with school (Simonsen and Rundmo, 2020).
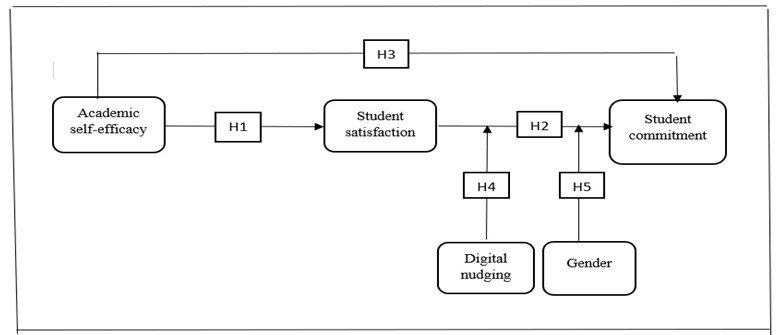
Fig 1: The proposed theoretical framework
H1: Academic self-efficacy has a significant impact on student satisfaction.
H2: Student satisfaction has a significant impact on student commitment.
H3: Academic self-efficacy has a significant impact on student commitment.
H4: Digital Nudging has a significant moderating impact on student commitment.
H5: Gender has a significant moderating impact on student commitment.
Methodology
The tool used for statistical analysis was SmartPLS for SEM-PLS modeling. The target population were students from one of the top accredited universities in Egypt and the sample size was 410 students. Participants of the study were students from different colleges inside the university who learnt online through the pandemic of COVID-19.
A questionnaire was distributed to the students online through a link on SurveyMonkey. The respondents to the questionnaire were 410 students from different colleges in the Arab Academy for Science, Technology and Maritime Transport such as College of Management and Technology, College of International Transport & Logistics, College of Language & Communication, College of Computing & Information Technology and College of Engineering & Technology.
The survey instrument to explain the independent variable was adopted by Sachitra and Bandara (2017); the mediating variable that has been identified before was adopted from the study by Harsasi and Sutawijaya (2018) while the dependent variable was adopted from Wilson et al. (2016). On the other hand, the moderating variable named digital nudging was adopted from Graham et al. (2017). Each variable’s indicators are measured using 5 points Likert scale with the following scale:
1=Strongly disagree
2=Disagree
3=Neither agree nor disagree
4=Agree
5=Strongly agree
Results
This section presents the data analysis part of this paper. The analysis of this paper was done using the statistical package for social sciences (SPSS V26) for basic descriptive statistics, and (SmartPLS 3.2.7) for SEM-PLS modeling. The first section deals with data preparation. The measurement model was evaluated for the reliability and validity of the instruments in section two. Several descriptive statistics and bivariate correlations are constructed in section three. Finally, the structural model for hypothesis testing and both moderation and mediation analysis were presented in section four.
Data Preparation
Hair et al. (2017) emphasize that the topics of the date being collected include odd response patterns, unpledged respondents, missing data, outliers, and data distribution, should be checked. Therefore, those main data problems are inspected using SPSS. The issue of missing data was inspected and found that some indicators have missing data; to overcome this problem, some techniques were proposed in the literature to remedy this problem. Among these methods, it was hypothesized that the EM algorithm and regression imputation would provide the most accurate estimates at all levels of missing data (Roth, 1994); (Little and Rubin, 2019). The EM algorithm was adopted for handling missing data using SPSS. The imputed dataset was then imported into SmartPLS software for further analysis.
Descriptive statistics
Table (1) illustrates the descriptive statistics for various concepts. In respect to the online student satisfaction, the mean score varies from 3.601 to 4.044 concerning digital nudging. The correlation coefficients fluctuate from 0.468 to 0.645 between the constructs, indicating medium correlations between them.
Table 1: Descriptive statistics and multiple correlations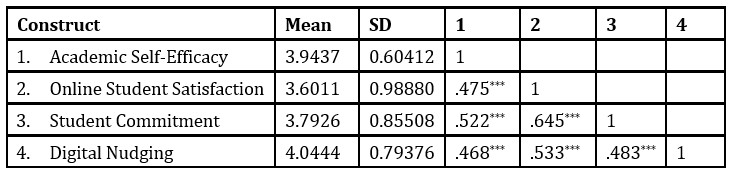
***P < 0.001
Assessing the Measurement Model
Structural equation modeling is an analysis approach that tests both the measurement model and the structural model that helps to develop more realistic assumptions (Abdi, 2010); (Hair et al., 2017). The measurement models describe the relationships between the constructs and their items. The assessment of the reflective measurement models in PLS-SEM requires evaluating the internal consistency reliability, convergent validity, and discriminant validity. The internal consistency reliability examines whether all of the indicators associated with a construct are actually measuring it (Pallant and Manual, 2010).
Table 2: The assessments of the measurement model (validity and reliability)
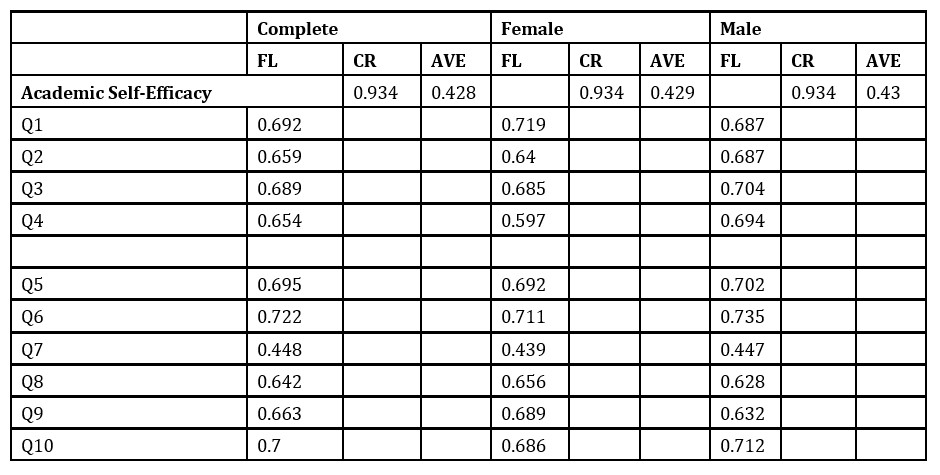
Table 3: The assessments of the measurement model (validity and reliability) (continued)
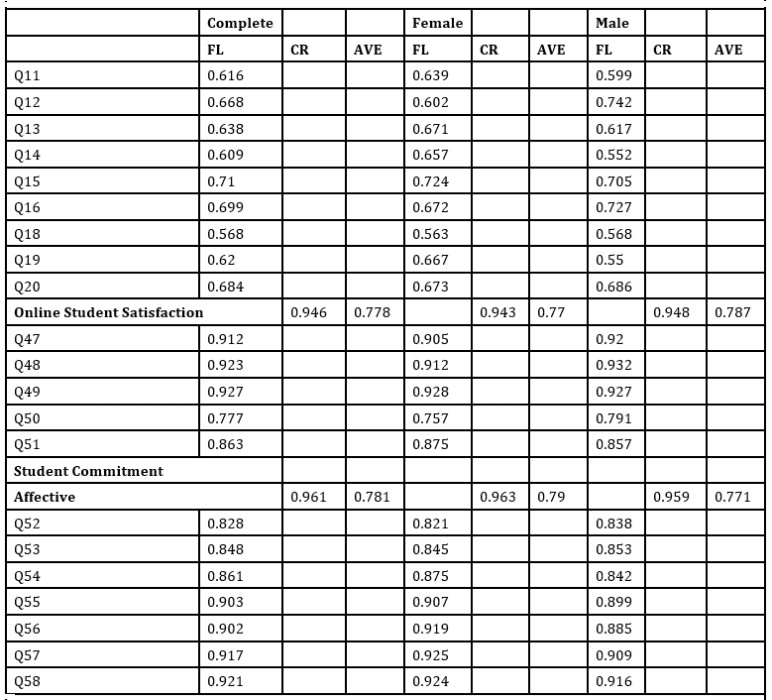
Table 4: The assessments of the measurement model (validity and reliability) (continued)
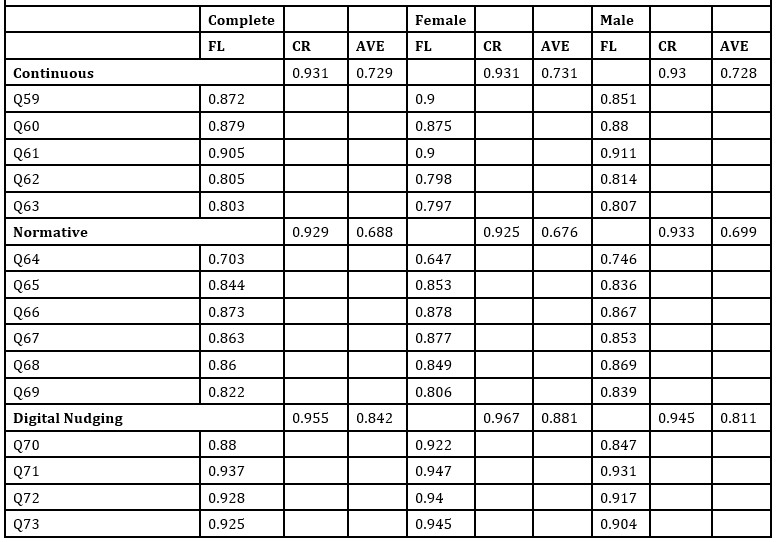
FL = Factor Loading, CR = Composite Reliability, AVE = Average Variance Extracted
The results of the measurement model for the pooled sample were measured for each group separately. (Hair et al., 2019). Tables (2,3 and 4) show the factor loadings (FL), composite reliability (CR) and average variance extracted (AVE) values. Most of the factor loading’s values were higher than 0.70 threshold, while the other value where between the accepted range of (0.4-0.7) except for Q17 which was below 0.4, is deleted. We examine the effect of deleting the items inside the range of (0.4-0.7) and found that no significance changes in the reliability and validity measures, so as a result, the items having FL inside the aforementioned range were retained. Composite reliability is another popular measure; it tests the internal consistency
while considering that each indicator has a different outer loading. All values of CR were greater than the threshold of 0.7, indicating that the measurement model was reliable inside (Hair et al., 2013). The AVE is a common measure used to establish convergent validity which represents the outstanding mean of the squared loadings of the indicators measuring a construct. The AVE of a construct should be 0.50 or higher to be considered significant. However, AVE values greater than 0.4 are also acceptable if CR values are greater than 0.6 (Fornell and Larker, 1981). Following the previous guidelines, the convergent validity through AVE was established as shown in tables (2,3 and 4).
Table 5: The assessments of measurement model II (discriminant validity)
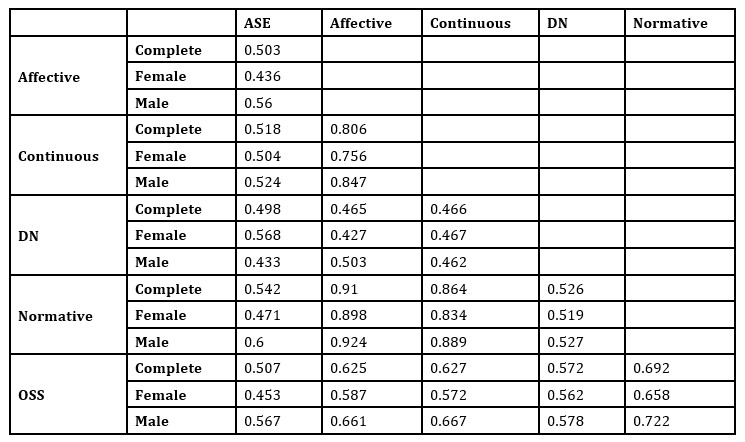
Discriminant validity examines how much a construct differs from other constructs. Discriminant validity is established using Hetrotrait-Monotrait ratio (HTMT). The HTMT is “the ratio of the between-trait correlations to the within-traits correlations” (Hair et al., 2016). The HTMT value should be lower than 0.90 (Henseler et al., 2015). Following these guides, the discriminant validity was constructed since all of the constructs have HTMT values less than the defined threshold except for the ratio between normative and affective.
Considering the different constructs to test the measurement invariance between females and males, the measurement invariance of composite models (MICOM) procedure which was developed by Henseler et al. (2016) was measured. The MICOM procedure includes three stages: (1) configural invariance; (2) compositional invariance; and (3) the equality of the composite mean values and variances (Hair et al., 2018). After following a separate assessment of the measurement models for the male and female samples (Tables 5), validity and reliability measures were found to be acceptable for both samples.
Table 6: Compositional Invariance
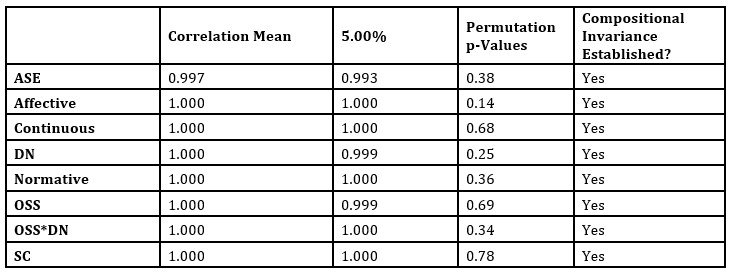
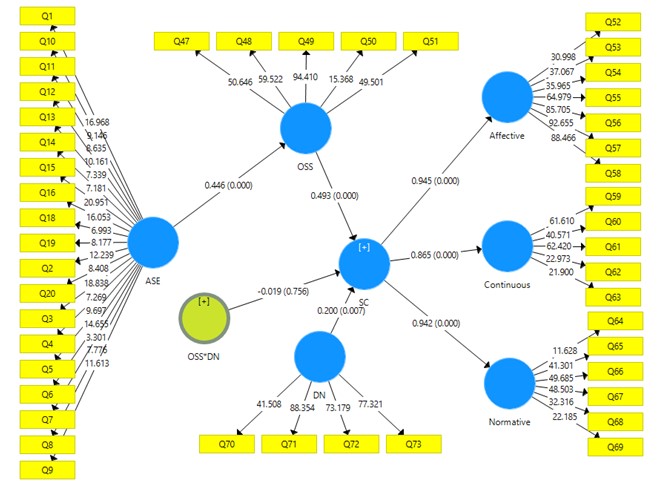
Fig 2: Path coefficients for female group data
Assessment of the structural model
After confirming that the measurement models were valid and reliable and that measurement invariance could be recognized, the structural model was measured. There were no collinearity subjects and the values were within a range of 1.000–1.617 based on a confirmation of checking the variance influence factor (VIF). The results confirm the nonappearance of collinearity issues since the VIF values are less than the threshold of 5 (Hair et al., 2017). Table (7) presents the results of the path relationships for the complete group. It can be observed that there is a significant direct positive relationship between academic self-efficacy and online student satisfaction since

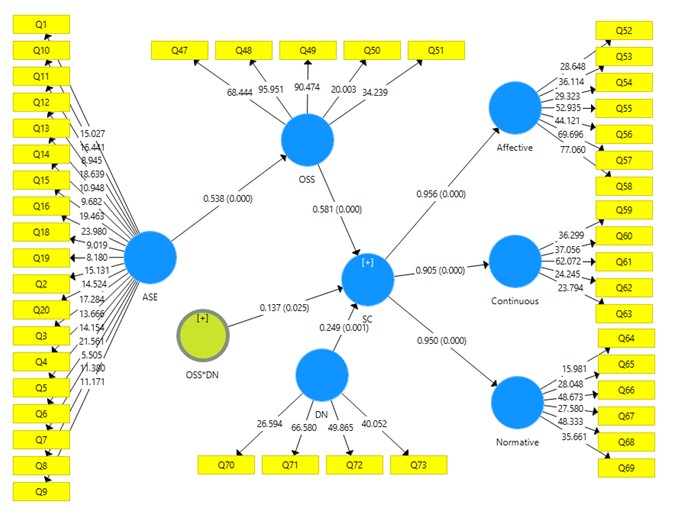
Fig 3: Path coefficients for male group data
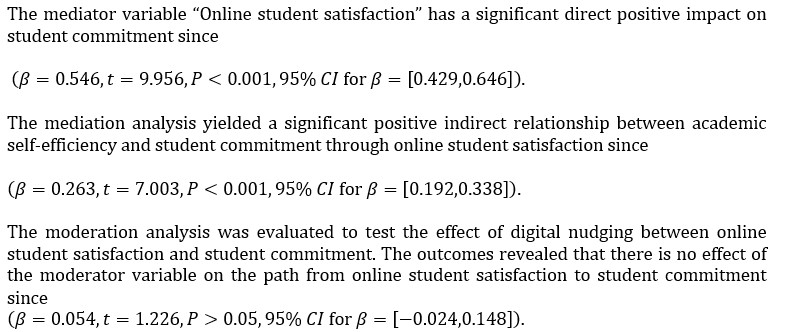
Table 7: Hypothesis Testing
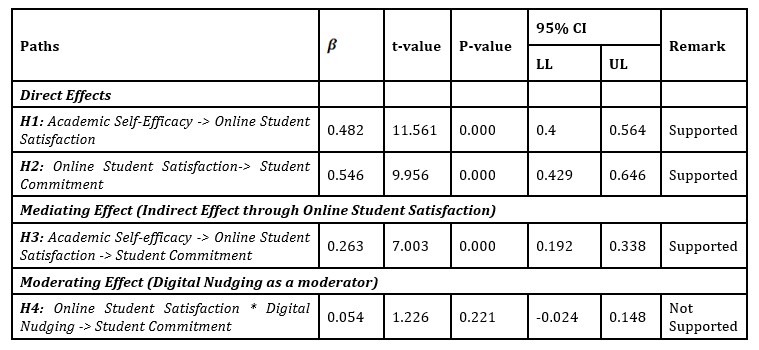
***P<0.001
Table 8: Assessment of structural model
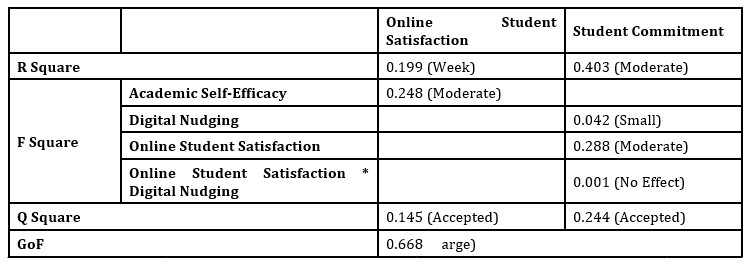
Moderating Effect of Gender
Since the moderator variable was not continuous, the moderating effect was determined based on group comparisons by multi-group analysis (MGA). Moreover, dichotomization was a common technique used when the moderating variables were
divided into two value categories. Based on Table 8, respondents’s gender did not moderate their academic self-efficiency and online satisfaction towards their commitment. However, it moderates the moderating effect of digital nudging on the relationship from online student satisfaction to student commitment.
Table 9: Moderating effect of gender
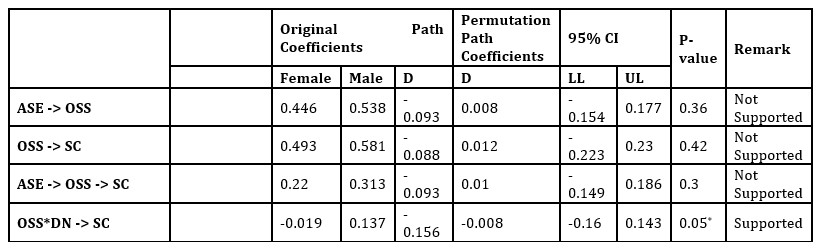
D = Difference, CI = Confidence Intervals, LL = Lower Limit, UL = Upper Limit
*P ≤ 0.05
Discussion of findings
As indicated in the results section above, this study found that academic self-efficacy has a positive correlation with students’ online commitment. This result indicated that whenever students show high levels of self-efficacy towards the tasks or assignments given to them, they will be more committed to attain their goals or tasks, more committed to their institution and more committed not to leave their academic institution. This is aligned with what Bandura (1977) stated about self-efficacy and how it influences the behavior of people to be committed toward a certain action or task. In addition, it also agrees with the study by Zeb and Nawaz (2016) who stated that self-efficacy is positively correlated to the faculty members’ organizational commitment in Pakistan universities. Another study by Saremi and Rezeghi (2015) revealed the same results as well as the study by (Almutairi, 2020).
Concerning the relationship between self-efficacy and satisfaction, the findings revealed that there is a positive relationship between the two constructs. A study by Shen et al. (2013) investigated the relation between online self-efficacy and student satisfaction and the results show that all variables of online self-efficacy have a positive effect on student satisfaction. In addition, Gunawardena et al. (2010) investigated some factors that expect satisfaction of learners and found that online self-efficacy is a strong influencer of the satisfaction of students. A study by Prifti (2020) suggested that course satisfaction can be developed by applying tools that boost self-efficacy of students in a blended learning environment.
Some demographic characteristics such as age and gender have impact on individuals’ attitudes and behaviors at their work. The age and gender of participants are to be studied in educational settings (Hall, 2014). Females and males are different at work and at life generally (Colley and Comber, 2003). Males in general have agentic behavior that let them behave in a competitive-oriented manner while females generally have communal behavior that let them behave in a nurturing and socially-oriented manner (Karakuş, 2018). A study by Chukwusa (2020) revealed that there was a significant difference between male and female staff on job satisfaction. It also revealed no significant impact of gender on the organizational commitment.
Nudging is a tool that affects the decision or behavior of students. For example, Castleman and Page (2015) sent text message reminders to secondary school students intending to go to college and to their parents. The reminders were about upcoming deadlines and tasks required for enrolment in their college as well as information available for them in case they need any help. The findings reveal that reminders can increase some colleges enrollment but only in regions where students have little access for help to complete the enrolment process. Moreover, a study by Castleman and Meyer (2016) sent reminders of tasks, access to advising and encouragement to students and the results revealed no effect of nudging. Other studies (Kizilcec et al., 2014, Unkovic et al., 2016) indicated the same results as well. The insignificant moderating impact of nudging on both student satisfaction and commitment in an online learning environment, in this study, may be due to the nature of the academy’s students in specific or Egyptian nature in general that needs more investigation in further research.
There are theoretical and practical implications in this study. Theoretically, when students’ self-efficacy is high, this means more committed students to their tasks, institution and obligation to stay at their university. Moreover, the high levels of self-efficacy that the students have will result in more satisfied students with the whole online learning system.
Practically, self-efficacy as a theory is a major factor that have strong impact on the behavior of students in the higher education context. When an academic institution has students with high self-efficacy levels, it is developing more satisfied and thus more committed students who are available to learn and develop for the sake of their institution and for the society. The key implication for this study is that it sheds the light on other academic institutions or higher education universities to explore the impact of nudging on students’ behavior when applying the online learning. This will help to investigate whether applying nudges is sufficient to change or alter the behavior of students in a positive or negative way.
Conclusion
The present study used structure equation modeling to investigate the impact of academic self-efficacy of students towards their satisfaction and commitment in an online learning environment during the pandemic of COVID-19 and the moderating effect of both digital nudging and gender. Few studies have been developed to examine levels of academic self-efficacy of students in the Egyptian higher education context through the pandemic as well as examining the moderating effect of digital nudging on both students’ commitment and satisfaction in an online learning environment.
Over the examination of a sample of 410 students enrolled in an Egyptian higher education institution, the study proved the validity of all the indicators of the variables in the proposed model. Moreover, academic self-efficacy had a significant effect on student satisfaction as well as student commitment; there was a positive relation between student satisfaction and student commitment; digital nudging had an insignificant impact on student satisfaction as well as student commitment. In addition, the majority of students’ responses were from College of Management and Technology while other colleges were of the same responses, and this can justify that even disciplines can differ from one another in the satisfaction and commitment level. Moreover, most responses were Males; females were 196 students while males were 208. Therefore, based on the previous literature review, all variables had significant influence on both student satisfaction and commitment except the variable named digital nudging.
Though the present study provides significant information for students and decision makers concerned with levels of student satisfaction and commitment in online learning environment, it was limited to be applied on a specific higher education institution inside Egypt, applying it in normal environment without the existence of any crisis and other moderating variables can be tested and explored in such study. To address this limitation, the study should be expanded to other higher education institutions inside Egypt in different settings. It will be beneficial and useful for academics and decision makers to understand the behavior of students concerned with the commitment towards their institutions.
Future exploration and investigation might embrace testing the model on other higher education institutions to identify its validity in forecasting the satisfaction and commitment of students in an online learning environment with respect to their academic self-efficacy and gender differences. Furthermore, more variables can be tested to explore their effect on both satisfaction and commitment as well as COVID-19 might have an influence on the results that this study has reached.
References
- <1472.pdf>.
- ABDI, H. 2010. Partial least squares regression and projection on latent structure regression (PLS Regression). Wiley interdisciplinary reviews: computational statistics, 2, 97-106.
- ADEWALE, A. S., GHAVIFEKR, S. & DAUD, M. A. K. B. M. 2017. Impact of leadership self-efficacy on organizational citizenship behaviour: A qualitative analysis on academic leaders’ perceptions. International Research Journal of Education and Sciences, 1, 41-47.
- ALLEN, N. & MEYER, J. 1990. The measurement and antecedents of affective, continuance and normative commitment to the organization. . Journal of Occupational Psychology, 63, 1-18.
- ALLEN, N. & MEYER, J. 1991. A three-component conceptualization of organizational commitment. Human resource management review, 1, 61-89.
- ALLEN, N. & MEYER, J. 1996. Affective, continuance, and normative commitment to the organization: An examination of construct validity. Journal of vocational behavior, 49, 252-276.
- ALMUTAIRI, Y. M. N. 2020. Leadership Self-Efficacy and Organizational Commitment of Faculty Members: Higher Education. Administrative Sciences, 10,
- ALSHEHRI, A. F. 2017. Student satisfaction and commitment towards a blended learning finance course: A new evidence from using the investment model. Research in International Business and Finance, 41, 423-433.
- ANARI, N. N. 2012. Teachers: emotional intelligence, job satisfaction, and organizational commitment. Journal of workplace Learning.
- BANDURA, A. 1977. Self-efficacy: toward a unifying theory of behavioral change. Psychological review, 84,
- BANDURA, A. 2002. Social Cognitive Theory in Cultural Context. Journal of Applied Psychology: An International Review, 51, 269–290.
- BANDURA, A. GUIDE FOR CONSTRUCTING SELF-EFFICACY SCALES. 2006.
- BASHIR, B. & GANAI, A. 2019. Testing the effects of job satisfaction on organizational commitment. Journal of Management Development, ahead-of-print.
- CASTLEMAN, B. L. & MEYER, K. E. 2016. Can text message nudges improve academic outcomes in college? Evidence from a West Virginia initiative. The Review of Higher Education, 43, 1125-1165.
- CASTLEMAN, B. L. & PAGE, L. C. 2015. Summer nudging: Can personalized text messages and peer mentor outreach increase college going among low-income high school graduates? Journal of Economic Behavior & Organization, 115, 144-160.
- CHUKWUSA, J. 2020. Gender Difference in Organizational Commitment, Job Satisfaction and Job Involvement: Evidence from University Library Staff. International Information & Library Review, 52, 193-201.
- COLLEY, A. & COMBER, C. 2003. School subject preferences: age and gender differences revisited. Educational studies, 29, 59-67.
- DAVISON, R. M. 2020. The Transformative Potential of Disruptions: A Viewpoint. International Journal of Information Management.
- DEMIR, S. 2020. The role of self-efficacy in job satisfaction, organizational commitment, motivation and job involvement. Eurasian Journal of Educational Research, 20, 205-224.
- DEWITZ, S. J. & WALSH, W. B. 2002. Self-efficacy and college student satisfaction. Journal of career Assessment, 10, 315-326.
- DWIVEDI, Y. K., HUGHES, D. L., COOMBS, C., CONSTANTIOU, I., DUAN, Y., EDWARDS, J. S., GUPTA, B., LAL, B., MISRA, S., PRASHANT, P., RAMAN, R., RANA, N. P., SHARMA, S. K. & UPADHYAY, N. 2020. Impact of COVID-19 pandemic on information management research and practice: Transforming education, work and life. International Journal of Information Management.
- EL-EBIARY, Y., AL-SAMMARRAIE, N., MOAIAD, Y. & ALZUBI, M. 2016. The impact of Management Information System in educational organizations processes.
- FORNELL, C. & LARKER, D. 1981. Evaluating Structural Equation Models With Unobservable Variable Sand Measurement Error. Journal of Marketing Research, 18, 39-50.
- GRACE, D. & KHALSA, S. 2003. Re-recruiting faculty and staff: The antidote to today’s high attrition. Independent school, 62, 20-27.
- GRAHAM, A., TOON, I., WYNN-WILLIAMS, K. & BEATSON, N. 2017. Using ‘nudges’ to encourage student engagement: An exploratory study from the UK and New Zealand. The International Journal of Management Education, 15, 36-46.
- GUNAWARDENA, C. N., LINDER-VANBERSCHOT, J. A., LAPOINTE, D. K. & RAO, L. 2010. Predictors of learner satisfaction and transfer of learning in a corporate online education program. The Amer. Jrnl. of Distance Education, 24, 207-226.
- HAIR, J., HULT, G. T., RINGLE, C. & SARSTEDT, M. 2017. A primer on partial least squares structural equation modeling (PLS-SEM), Sage publications.
- HAIR, J., PAGE, M. & BRUNSVELD, N. 2016. Essentials of business research methods, Routledge.
- HAIR, J., RINGLE, C. & SARSTEDT, M. 2013. Partial least squares structural equation modeling: Rigorous applications, better results and higher acceptance. Long range planning, 46, 1-12.
- HAIR, J., RISHER, J., SARSTEDT, M. & RINGLE, C. 2019. When to use and how to report the results of PLS-SEM. European Business Review.
- HALL, N. T. 2014. Parental Involvement at the High School Level: Parents’ Perspective.
- HARSASI, M. & SUTAWIJAYA, A. 2018. Determinants of Student Satisfaction in Online Tutorial: A Study of A Distance Education Institution. Turkish Online Journal of Distance Education, 19, 89-99.
- HEFFERON, K., & BONIWELL, I 2011. Positive psychology: Theory, research and applications, New York: Mc Graw-Hill Open International Publishing Ltd.
- HENSELER, J., RINGLE, C. M. & SARSTEDT, M. 2015. A new criterion for assessing discriminant validity in variance-based structural equation modeling. Journal of the academy of marketing science, 43, 115-135.
- HENSELER, J., RINGLE, C. M. & SARSTEDT, M. 2016. A new criterion for assessing discriminant validity in variance-based structural equation modeling. Journal of the academy of marketing science, 43, 115-135.
- KARAKUŞ, M. 2018. The moderating effect of gender on the relationships between age, ethical leadership, and organizational commitment. Journal of Ethnic and Cultural Studies, 5, 74-84.
- KIZILCEC, R. F., SALTARELLI, A. J., REICH, J. & COHEN, G. L. 2014. Closing global achievement gaps in MOOCs. Science, 355, 251-252.
- LEE, R. & MILLER, T. Assessing the performance of the Lee-Carter approach to modeling and forecasting mortality. Annual Meeting of the Population Association of America, Los Angeles, 2001.
- LILIN & SHIQIAN, W. 2018. Self-efficacy, organizational commitment, and employee engagement in small and medium-sized enterprises. International Journal of Business Marketing and Management (IJBMM), 3, 35-39.
- LITTLE, R. J. & RUBIN, D. B. 2019. Statistical analysis with missing data, John Wiley & Sons.
- LUSZCZYNSKA, A., SCHOLZ, U. & SCHWARZER, R. 2005. The general self-efficacy scale: multicultural validation studies. The Journal of psychology, 139, 439-457.
- MAYER, R. E. 2003. Elements of a Science of E-Learning. Journal of Educational Computing Research, 29, 297-313.
- PAJARES, F. & SCHUNK, D. H. 2001. Self-beliefs and school success: Self-efficacy, self-concept, and school achievement. Perception, 11, 239-266.
- PALLANT, J. & MANUAL, S. S. 2010. A step by step guide to data analysis using SPSS. Berkshire UK: McGraw-Hill Education.
- PRIFTI, R. 2020. Self–efficacy and student satisfaction in the context of blended learning courses. Open Learning: The Journal of Open, Distance and e-Learning, 1-15.
- ROBBINS, S. P., COULTER, M. K. & DECENZO, D. A. 2017. Fundamentals of management.
- ROTH, P. L. 1994. Missing data: A conceptual review for applied psychologists. Personnel psychology, 47, 537-560.
- SABRI, P. S. U., ILYAS, M. & AMJAD, Z. 2011. Organizational culture and its impact on the job satisfaction of the University teachers of Lahore. International Journal of Business and Social Science,
- SACHITRA, V. & BANDARA, U. 2017. Measuring the Academic Self-Efficacy of Undergraduates: The Role of Gender and Academic Year Experience.
- SAREMI, H. & REZEGHI, A. A. 2015. A study on the relationship between self-efficacy beliefs and organizational commitment with job satisfaction in office employees in Esfarayen city, Iran. International Journal of Life Sciences, 9, 15-23.
- SCHERMERHORN, J. R., HUNT, J. G., OSBORN, R. N. & UHL-BIEN, M. 2011. Organizational behaviour, Langara College.
- SCHWARZER, R. & JERUSALEM, M. 1995. Generalized self-efficacy scale. Measures in health psychology: A user’s portfolio. Causal and control beliefs, 1, 35-37.
- SHEN, D., CHO, M.-H., TSAI, C.-L. & MARRA, R. 2013. Unpacking online learning experiences: Online learning self-efficacy and learning satisfaction. The Internet and Higher Education, 19, 10-17.
- SIMONSEN, I.-E. & RUNDMO, T. 2020. The role of school identification and self-efficacy in school satisfaction among Norwegian high-school students. Social Psychology of Education, 23, 1565-1586.
- SMART, K. & CAPPEL, J. 2006. Students’ Perceptions of Online Learning: A Comparative Study. JITE, 5, 201-219.
- TSUI, K. T. & CHENG, Y. C. 1999. School organizational health and teacher commitment: A contingency study with multi-level analysis. Educational Research and Evaluation, 5, 249-268.
- UNKOVIC, C., SEN, M. & QUINN, K. M. 2016. Does encouragement matter in improving gender imbalances in technical fields? Evidence from a randomized controlled trial. PloS one, 11,
- VANDERPUTTEN, J. & WIMSATT, L. Cross-national faculty departure: Development of a new model. annual meeting of the Association for the Study of Higher Education, San Antonio, TX, 1999.
- WILSON, M., BAKKABULINDI, F. & SSEMPEBWA, J. 2016. Validity and Reliability of Allen and Meyer’s (1990) Measure of Employee Commitment in the Context of Academic Staff in Universities in Uganda. JOURNAL OF SOCIOLOGY AND EDUCATION IN AFRICA,
- ZEB, M. & NAWAZ, A. 2016. Impacts of Self-Efficacy on Organizational Commitment of Academicians A Case of Gomal University. Information and Knowledge Management, 6, 36-42.
- ZEB, M. S. & NAWAZ., A. 2016. Impacts of self-efficacy on organizational commitment of academicians: A case of Gomal University, Dera Ismail Khan, Khyber Pakhtunkhwa, Pakistan. Information and Knowledge Management, 6, 36–42.