Introduction
UAVs – unmanned aerial vehicles – are finding more and more possibilities for application in various areas of social life. The applicability of this technology is expanding rapidly. Initial analysis of research projects from around the world identified the use of UAVs in several diverse sectors of the economy. An example is research from Korea, where the authors examined the approach of potential customers to the application of the technology in the food delivery process (Hwang et al., 2021). In this research, specific traits of behaviour and attitudes influencing customers’ readiness to consider such uses of drones have been identified.
Another example is the use of drones in agriculture and forestry, which are described by Liang and Delahaye (2019). Drones provide advantages in comparison with other monitoring methods used so far. The authors point out the need for managerial decision-making to find a global optimal solution. It is therefore a matter of deciding between one drone and the creation of a fleet of several drones. In the case of creating a fleet, it is important to deal with the cooperation of individual drones, their mutual communication, and the procedure of processing the data generated by their application. Further uses of drones in agriculture, in the rice sowing process, are described by Worakuldumrongdej et al. (2019). Fanigliulo et al. investigated the application of UAVs in the analysis of tillage quality (2020). Even in this specific application, drones can offer various advantages over conventional methods. However, from the perspective of managerial decision-making, it is necessary to evaluate the effectiveness of this method’s deployment, based on the collection and evaluation of relevant data (Koman et al., 2018).
The use of drones can also be observed in the field of structural health monitoring. In a specific example, described by Sony et al., drones are used in combination with noncontact sensors to complement vibration data obtained from contact sensors (2019). In this way, the UAV contributes to the continuous analysis of the condition of buildings, which helps predict failures or major changes that can threaten the occupants or visitors of the building, and thus prevent incidents.
Finally, drones could also be applied in the field of urban vegetation mapping. In this case, it is the mapping of vegetation in a complex urban landscape. They are used to obtain ultra-high-resolution images captured at low altitudes (Feng et al., 2015). Again, it is an opportunity to obtain new data sources that can be used in the subsequent process of managerial decision-making targeted at a specific case/situation. Proper use of the data collected this way, for example in making predictions, can bring numerous benefits for the stakeholders.
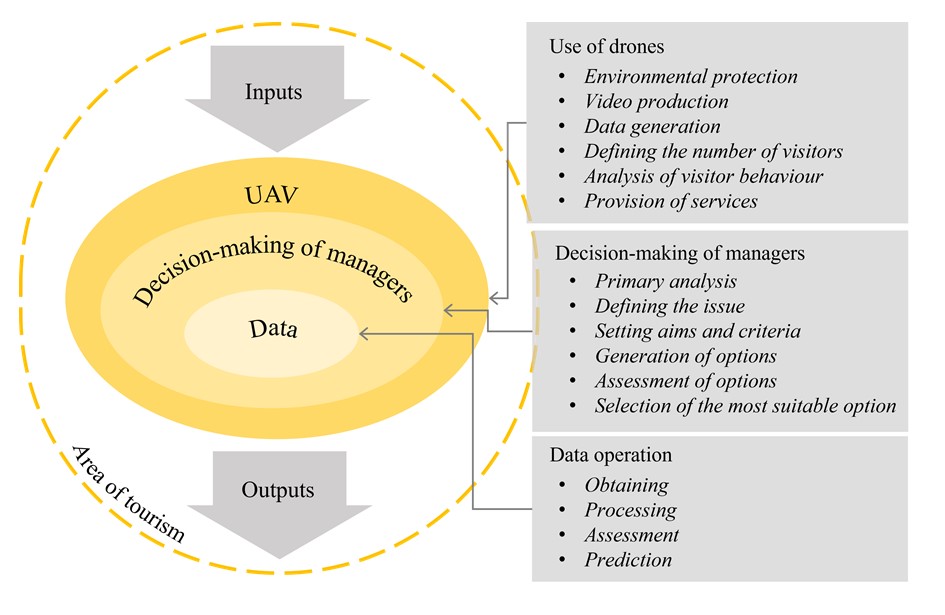
Fig 1. Decision-making of managers on the application of drones
supported by data and predictions (Authors’ own elaboration)
Based on the analysis of other authors’ opinions, it can be stated that the issue of drones’ application in tourism is a currently developing, and so far, little explored area.
Theoretical background
The analysis of the theoretical basis, in connection with the research goal, included an overview of current knowledge in the areas of managerial decision-making necessary for the optimal setting of individual business processes, predictive econometric models, and the use of the UAV technology in tourism.
Description of managerial decision-making
In any sector, the main part of the managers’ work is decision-making. This process plays a key role in the operation of a company. Managerial experts ask themselves many questions with various levels of complexity every day. For example, Barlett focuses on: “How do the decisions of specific individuals and groups affect the decision-making environment, or What variables influence a given choice, and how many stimuli influencing decisions need to be understood?” (2016, p. 3). He also gives a specific example from the field of agriculture in his work, based on which it can be stated that decision-making is a complex process. It is influenced by several factors and depends on the perceived needs and defined goals. Saafy states that decision-making can also be characterized as an analytical and hierarchical process consisting of several steps: defining the problem, determining the type of knowledge required; structuring specific goals; defining criteria and variants; creating a set of comparison matrices (2008, p. 85).
Decision-making is one of the criteria of professional competence in the field of management. When performing work tasks, the manager often engages in critical examination of problems, their solution, and final decision-making. The quality of top managers’ decisions is the factor that has the greatest impact on their success or failure. Based on the statements above, decision-making can be defined not only as the most key internal activity of a leader, but also as the core of management (Marquis, Huston, 2009, p. 1). Managerial decision-making is a complex of multilevel processes that are influenced by a plethora of factors (Hodgkinson, Sadler-Smith, 2018, p. 4). The strategic decisions of top management teams affect the performance of the entire company. Consensus among team members facilitates the implementation of these decisions and thus also affects the performance of the whole company. Therefore, the quality of decision-making, consensus, and affective acceptance are necessary for the sustainable performance (Amason, 1996, p. 123). The adoption of a decision without its actual implementation has no effect on the environment in which it was taken. Once a decision has been taken, responsibilities for carrying out specific activities must be assigned and exercised (Verma, 2009, p. 268).
Large-Scale Group Decision Making is often used to solve common decision-making problems in companies. It concerns the selection of the best option from a set of feasible variants, based on the preferences of many decision makers (Liu, Fan, Zhang, 2016, p. 2). However, different opinions among decision-makers can cause conflicts within the group (Giordano et al., 2007, p. 3). Some authors think that conflict within a group does not lead to an overall decision-making process but can lead to some inconsistency. However, negative consequences can be huge (Zhu, Chen, Zhao, 2014, p. 3). The method of Consensus Reaching Processes (Liu et al., 2018, p. 3) is used to mitigate or eliminate the conflict for a smoother implementation of the decision.
Econometric models created to predict future course of variables
The use of predictive modelling is currently very wide, not excluding the field of tourism. The authors Höpken et al. (2017) applied the statistical approach of linear regression to the predictions of tourism demand and the data mining technique of k-nearest neighbour. Höpken et al. compare two approaches, namely ARIMA (autoregressive integrated moving average) models, from the group of statistical prediction methods, and ANNs (artificial neural networks), based on machine learning (2020). Supriatna et al. compare the accuracy of Holt Winter’s approach to statistical modelling applied to tourism (2019).
The choice of the prediction method depends on the data sources, the length of the time series, and the frequency of monitoring these data. The choice of method is preceded by the analysis of properties, including the internal structure of a given time series and the examination of factors and their interrelationships. For short-term and long-term time series, distinguishing them according to the assumed seasonal component, approaches of regression models as well as exponential smoothing can be applied. Exponential smoothing methods, due to the principle of decreasing weights for older observations, allow a quick response to changes in data fluctuations. Additionally, their application does not require restrictions regarding the amount of data. According to Holt and Winter, the group of models enables work with seasonal and non-seasonal data (Chatfield, 1978).
The method of supporting vectors in the field of machine learning methods, originally developed for classification tasks, is now widely represented in prediction tasks in its regression form. The use of this approach, either alone or in combination with other approaches, such as genetic algorithms (Pai et al., 2006), opens new possibilities for predictive modelling not only in tourism decision-making problems.
Application of UAV technology in tourism
For the purposes of this article, the authors focused on the use of UAVs in the field of tourism. Therefore, an analysis of the research presented so far focused on the use of drones in this area was carried out. The result of the analysis is a comparison of opinions and their structuring into three separate, more narrowly focused areas. The first of such areas is the destination marketing and online content.
Marketing of tourist destinations creation of content, and online tourism
A specific opportunity of the UAV application in tourism is also the use of drones for organizing virtual online tours of outdoor tourist attractions (Ilkhanizadeh et al., 2020). Mathematical-statistical and predictive modelling techniques were used to determine the optimal number of drones to cover a given attraction. These must be applied in relation to the demand for such tours.
Mirk and Hlavacs also studied this type of UAV application in tourism. They state that drones find their use in shooting live videos from tourist attractions, which they send in real time to their customers – consumers (2014). In addition, video content generated by cameras attached to drones can then be used to further promote the tourist destination in combination with social networks. For example, if the videos are made available to the public on the YouTube network, it is possible to set up the collection of metadata in such a way that they can be subsequently analysed and used for making predictions (Stankov et al., 2019). Predictions are one of the functional tools for decision-making being performed by managers of tourist destinations.
It is now common practice to use data from the online environment to specify and adapt advertisements to the users – potential customers. The application of drones can also bring a new dimension to such a sphere by tracking visitors at crowded tourist places. Donaire et al. focused on this aspect in their research (2020). Drones were used to monitor the behaviour of visitors to archaeological sites and significant buildings (temples, churches). The UAV technology can thus be helpful in setting the capacity of visitors to individual monuments flexibly during the day. Online portals and travel agency sites can use the data in real time to help customers choose a suitable (non-crowded) attraction/landmark. The capacities of monuments can thus be used more optimally, and the customers’ satisfaction as well as the demand for these services will increase
Issues of security and protection
The second important area where the UAV finds its application in connection with tourism is the protection of nature and people. Drones can be used to monitor visitors in protected areas, as described by Ancin-Murguzur et al. (2020). The work of this team presents the study of visitors’ behaviour patterns, the effects of visitors on the vegetation or on the condition of sidewalks, and the garbage left in the protected natural areas. The benefit of such a solution is the rapid detection of a problem, in this case, the nature pollution. Subsequently, using drones, it is possible to determine the exact location, thus the elimination of the problem is more efficient and faster.
Other current research focused on the use of UAVs in nature protection includes the project conducted by Castro-Barrantes (2020). Data were collected in the Costa Rica as a part of a water conservation education project. This focused on finding new sustainable practices. Its specific goal was to define environmental zones and the maximum daily capacity of tourists in the given zones to ensure the long-term protection of a valuable water resource, creating a sustainable tourist model in a specific locality.
The aspect of people’s protection was addressed by Stokes et al., who describe the use of drones in coastal monitoring to increase shark bite prevention in Australia (2020). Data from the partial survey suggest that most respondents had no objections to such use of drones. In terms of importance, they prefer to protect their health over any invasion of their privacy. Human safety, which can be enhanced via the technology presented in tourism activities, has also been investigated by Lee et al. (2020). The authors describe the use of drones in examining the safety of hiking trails. In addition to safety and assessment of the suitability of the route for hiking, drones allow people to focus on the aesthetic qualities of the examined places. Thus, UAVs obtain data not only for safety analysis, but also for the aesthetic properties of the route. Potential tourists will not come across problematic places, their tours will be visually attractive, which will ensure their high satisfaction. Such an application of drones emphasises the generation of new data, which must be evaluated and based on which it is necessary to predict the future development of the relevant characteristics.
The last aspect, which was mentioned in the sub-area “security and safety”, can be logically linked to the first sub-area presented – “destination marketing, content creation and online tourism” and its positive impacts.
Application of new technologies
The last selected area, which falls under the use of drones in tourism with the potential for its further development, is the combination of this technology with various other results of scientific and technological advancement. A specific example of promoting tourism and making a tourist destination more attractive in Southern Ireland is the analysis of the possibilities of using drones in combination with 3D printing (Keogh et al., 2017). Another example of the combined power of drones and other new technologies is the creation of new, more efficient algorithms for processing video recordings generated by drones (Lu, Shahabi, 2017).
The presented sub-area has great potential for further development. Nowadays, technological progress is an indispensable part of any area of life, not excluding tourism and travel. Therefore, it is necessary to focus on the options of finding new ways to connect UAVs with new technologies that will gradually come to the mass market.
The presented findings can be supplemented by specific statistics. Looking at the future, various estimates of the development of the commercial drone segment were made, which are addressed in this article. The Statista portal also reports the expected development of commercial drone revenue in Europe between 2016 and 2025. For example, by 2022, the European commercial drone market is expected to generate revenues of more than US $1,248.32 million (Statista, 2021a). However, this projected development may be influenced by the current situation associated with the COVID-19 pandemic. Overall, the significant increase in sales in this segment can be expected.
Regarding the current situation in connection with the application of commercial drones, another statistical evaluation can be added (Table 1).
Table 1: Drone-based services by activity in 2020, (Statista, 2021b)
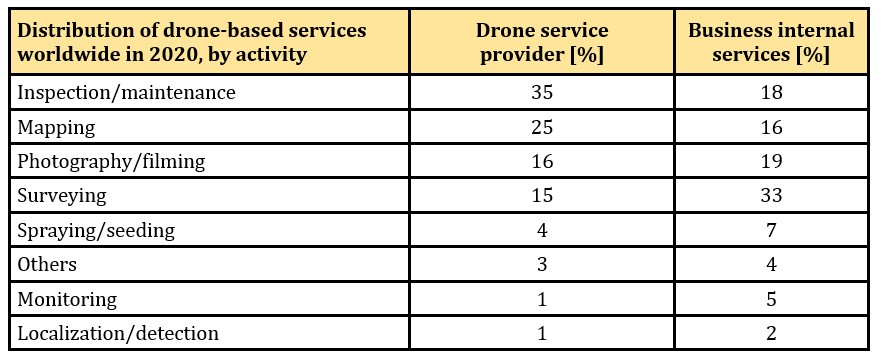
Currently, commercial drones are mostly used in two areas: ”surveying” and “inspection/ maintenance”. In “surveying”, companies provide these services (use of UAVs) mainly themselves, in contrast to the second area of “inspection/maintenance”, where they rely mainly on external drone service providers (Statista, 2021b). Based on these statistics, it can be stated that the use of drones is becoming more and more widespread across different areas. From the perspective of the research presented in this article, this is one of the important managerial decisions associated with the introduction of the UAV technology within the conditions of a particular entity operating in tourism.
Methodology
The aim of the article was to make recommendations for companies regarding the introduction of UAVs in tourism. By applying the specific points presented in the conclusion, managerial decision-making in a company will be streamlined via predictive models. To achieve this goal, the authors identified specific research questions at the beginning of the research: Does the UAV technology find application in the field of tourism? What is the role of predictive modelling using available data to increase customer satisfaction? What factors are important in the decision-making of tourism entities on the application of the UAV technology?
Description of data obtained
This article also focused on predictive modelling of short-term time series. The selected data describe the occupancy of chairlifts in selected mountain resorts. They can be divided into two groups, chairlifts in year-round operation and those in seasonal mode. The company providing the data is the operator of mountain resorts and tourist services. It focuses on Central and Eastern Europe. A specific indicator – the number of crossings is obtained from the turnstiles of individual cableways. The observed period is five years, and the studied variable represents the number of persons per week.
Methods Applied
Supporting methods and techniques were represented by the analysis of available relevant secondary sources, synthesis of partial findings, deduction allowing generalization of conclusions, and induction leading to the formulation of specific points of the procedure applicable to various tourism entities.
The aim of the experiments presented was to make predictions of the selected indicator using alternative approaches. Both approaches consider the length of individual time series and the internal structure of a given time series. For the final solution, the method of exponential smoothing from statistical methods and the method of supporting vectors (SVM) from the methods of machine learning were selected. The RStudio software working with the R programming language was used to perform mathematical calculations and make predictions.
The predictive modelling of selected time series was performed in several steps. The prediction itself was preceded by the appropriate preparation of the data set and the analysis of individual time series. The choice of statistical prediction method considers the assessment of data seasonality as well as stationarity. Stationarity was tested via KPSS (Kwiatkowski – Phillips – Schmidt – Shin test for stationarity) tests offered in RStudio functions. Due to the frequency of selected data, the presence of seasonality can be assumed. Graphical data analysis was included in the procedure. Based on the conclusions reached, the Holt and Winter approach was selected from the means of exponential smoothing, which enables the modelling of seasonal data. The software environment offers the HoltWinters() function with three balancing constants to include three components: level, trend, and season. The statistical modelling was compared with the machine learning approach. From several methods, the method of support vectors (support vector machines) was applied in the solution. The software environment offers the svm() function in the e1071 library for this access. Parameter optimization was performed via the tune() function, also offered by the open source product. Both in the exponential smoothing and in the machine learning method, the requirements of the methods were respected. The data set was divided into a training set and a test set. A 75:25 ratio was selected for the split.
The result of both approaches was the prediction of selected data. The quality of these predictions was assessed by the mean square error, or its square root (RMSE), as one of the criteria for evaluating the accuracy of prognostic models.
Results
As already mentioned earlier, experiments for time series obtained from the records of turnstiles of individual cableways were performed, both in year-round operation and in operation exclusively during the winter season. In this article, attention focused on Chairlift A, where the data and the results of predictive modelling were thoroughly described. Following the procedure described, the first step was a graphical data analysis showing weekly data over five years. It is a year-round operation, while the missing data represent the period when the chairlift did not run due to bad weather, maintenance, or reconstruction. The presence of the seasonal component results from the logical assumption of the tourist resort’s nature. In this case, it is a ski resort.
The expected increase in the occupancy of the chairlift in the winter in the following years compared to 2013 and 2014 was probably influenced, among other factors, using a loyalty card. In 2015, this increase was 56% compared to the previous period.
In the next modelling step, a KPSS was performed. Based on the value of the test statistics, the null hypothesis of process stationarity was rejected. According to the procedure described above, predictions of the data of this chairlift were created based on Holt Winter exponential smoothing and the method of supporting vectors.
Using the Holt and Winter method with the basic parameter settings, problems arose with negative values in the selected prediction horizon. Therefore, the attention was paid to this centre with year-round operation, specifically during the next quarter.
Using the method of support vectors, the basic parameters of the function were optimized via the tune() function. The modelling results were verified on the test data set. The predictions were obtained after the optimization of the parameters of the corresponding svm() function.
For the forecasting of the Chairlift A, weekly data were obtained from the turnstiles during the year-round operation. The indicated modelling procedure was applied at other centres, too.
The suitability of the procedures and the accuracy of the predictions were determined in all experiments based on the size of the RMSE criterion at two-time levels. One was a calculation on real data, which formed the basis for quantification. The second level was the forecast period. The support vector method was applied on the test set. During exponential smoothing, the real data of individual time series were divided into the period of quantification and verification to compare the chosen approaches. Based on the achieved results, it can be stated that despite the relatively high error rate, the SVM method is a better choice. In 86% of the experiments, the RMSE was lower with this method.
At the end of the modelling, it should be emphasized that the accuracy of the models as well as the following predictions is influenced by the choice of prediction method, as well as by factors that can be included in the models. The accuracy can also be affected by evaluation criteria, such as MAPE (mean absolute percent error). Despite the possibility of achieving higher accuracy when changing the input conditions of individual settings, two alternative approaches were successfully implemented, thus relevant predictions for selected resorts were created.
Conclusions and Discussion
This section describes recommendations for companies regarding the introduction of UAVs in tourism. The basic premise of the presented research was the possibility to achieve higher customer satisfaction of individual tourism services in the case of effective managerial decision-making. However, for such decision-making, it is essential that the entity has the relevant data available and that it can handle them appropriately. Working with the data obtained represents their analysis in the time and scope needed as well as the subsequent interpretation so that it is possible to prepare effective managerial decisions.
The UAV technology in connection with the researched industry represents new possibilities for collecting the data in real time. Specifically, it is a possibility to obtain new data on the utilization of the services provided and on the currently available staff, including their spatial distribution throughout the day. Consequently, econometric modelling and forecasting techniques represent a level of data analysis leading to interpretable information that can be used in the managerial decision-making process.
With the help of specific points presented below, managerial decision-making in companies will be streamlined via the application of predictive models:
- focusing on assessing the suitability of the use of UAV technology for a specific entity in the field of tourism;
- identification of use cases for drones in any of the processes being performed (data collection, provision of a new form of service, monitoring of customer safety, video recording);
- assessment of the costs of the envisaged solution related to the UAV technology;
- identification of other requirements related to the considered solution (human resources, existing technological infrastructure, and possibility of the UAV integration);
- assessment of the expected benefits arising from the implementation of the solution under consideration for the company and its various stakeholders;
- identification of risks associated with the solution and assessment of their probability and impact (legislative restrictions, negative attitude of stakeholders towards the given technology, non-acceptance of a new solution by current employees, etc.);
- proposal of corrective and preventive measures in connection with the identified risks and other activities needed to be performed before the implementation of the solution.
The companies in the tourism do not have to implement the UAV technology themselves. They can cooperate with other entities (Soviar et al., 2018). The utilization of drones and the growing interest in this technology is confirmed by the Statista portal (2021b). Organizations provide UAVs on their own or indirectly, and the possibilities for their use are constantly expanding across different areas. This justifies the topic of this article. One of the partial goals of the research was to identify the possibilities of applying UAVs in the field of tourism, as well as to identify the role of predictive modelling using available data in this area. Stankov et al. argue that predictions are one of the tools that should be used by destination managers in their decision-making (2019). The focus on customers when deciding on the implementation of UAVs is highlighted by many authors (Mirk, Hlavacs, 2014; Ancin-Murguzur et al., 2020; Donaire et al., 2020). They examine patterns of visitor behaviour or the tracking of visitors at crowded tourist sites.
An Australian research project by Stokes et al. also focused on the aspect of monitoring and analysing the views of stakeholders who can be exposed to drones. They remind the importance of respect towards attitudes of these people and demonstrate their findings on examples such as coastal monitoring for shark bite prevention (2020). Using prediction of behaviour based on data obtained via drones, the communication with stakeholders can be set in a way leading to the promotion of tourism.
Looking to the future of tourism, open thinking is important, leading to the connection of drones with evolving technologies. Examples where tourism was promoted this way include the increase in attractiveness of a tourist destination in Southern Ireland (Keogh et al., 2017) or the use of more efficient algorithms for processing drone-generated videos (Lu, Shahabi, 2017).
Acknowledgement
This publication was created with the support of Operational Program Integrated Infrastructure 2014 – 2020 of the project: Intelligent operating and processing systems for UAVs, code ITMS 313011V422, co-financed by the European Regional Development Fund.
References
- Amason, A. C. (1996). Distinguishing the Effects of Functional and Dysfunctional Conflict on Strategic Decision Making: Resolving a Paradox for Top Management Teams. In: Academy of Management J., 39, Issue 1, s. 123–148, ISSN: 0001-4273.
- Ancin-Murguzur, F J., Munoz, L., Monz, C. and Hausner, V H. (2020). Drones as a tool to monitor human impacts and vegetation changes in parks and protected areas. In: Remote sensing in ecology and conservation, Volume 6, Issue 1, Pages: 105-113, DOI: 10.1002/rse2.127.
- (2016). Agricultural Decision Making: Anthropological Contributions to Rural Development. London (UK): Academic Press. 395s. ISBN: 1-4832-6841-1.
- Castro-Barrantes, L. (2020). Tourism carrying capacity and environmental zoning of CEANA, oriented to visitors management and water resource protection in Cartago, Costa Rica. In: Revista forestal mesoamerica kuru-rfmk, Volume 17, Issue 41, Pages: 47-57, DOI: 10.18845/rfmk.v17i41.5283.
- Chatfield, C. (1978). The Holt-Winters Forecasting Procedure. In: Journal of the Royal Statistical Society. Series C (Applied Statistics), Volume 27, No. 3, pp. 264-279, DOI: https://doi.org/10.230
7/2347162.
- Donaire, J A., Gali, N. and Gulisova, B. (2020). Tracking visitors in crowded spaces using zenith images: Drones and time-lapse. In: Tourism management perspectives, Volume 35, ISSN: 2211-9736, DOI: 10.1016/j.tmp.2020.100680.
- Fanigliulo, R., Antonucci, F., Figorilli, S., Pochi, D., Pallottino, F., Fornaciari, L., Grilli, R. and Costa, C. (2020). Light Drone-Based Application to Assess Soil Tillage Quality Parameters. In: Sensors, Volume 20, Issue 3, eISSN: 1424-8220, DOI: 10.3390/s20030728.
- Feng, Q L., Liu, J T. and Gong, J H., (2015) UAV Remote Sensing for Urban Vegetation Mapping Using Random Forest and Texture Analysis. In: Remote Sens, Volume 7, Issue 1, Page: 1074-1094, DOI: 10.3390/rs70101074.
- Giordano, R., Passarella, G., Uricchio, V. and Vurro, M. (2007). Integrating conflict analysis and consensus reaching in a decision support system for water resource management, In: Journal of Environmental Management, 84, Issue 2, s. 213–228, ISSN: 0301-4797.
- Hodgkinson, G. P. and Sadler-Smith, E. (2018). The dynamics of intuition and analysis in managerial and organizational decision making. In: Academy of Management Perspectives. 32, Issue 4, s. 473–492, ISSN: 1558-9080.
- Höpken, W., Dominic, E., Fuchs, M., Kai, K. and Maria, L. (2017). Big Data as Input for Predicting Tourist Arrivals. In: Information and Communication Technologies in Tourism 2017, 187-199, DOI: 10.1007/978-3-319-51168-9_14.
- Höpken, W., Eberle, T., Fuchs, M. and Lexhagen, M. (2020). Improving Tourist Arrival Prediction: A Big Data and Artificial Neural Network Approach. In: Journal of Travel Research, Volume 60, DOI: https://doi.org/10.1177/0047287520921244.
- Hwang, J., Kim, J J. and Lee, K W. (2020). Investigating consumer innovativeness in the context of drone food delivery services: Its impact on attitude and behavioral intentions. In: Technological forecasting and social change, Volume 163, ISSN 0040-1625, DOI: https://doi.org/10.1016/j.techfore.2020.120433.
- Ilkhanizadeh, S., Golabi, M., Hesami, S. and Rjoub, H. (2020). The Potential Use of Drones for Tourism in Crises: A Facility Location Analysis Perspective. In: Journal of risk and financial management, Volume 13, Issue 10, DOI: 10.3390/jrfm13100246.
- Keogh, C., Hyland, V. and Nassar, R. (2017). 3D Rocks – Augmented reality Islands for Skellig Kerry region. In: Proceedings of the 2017 23rd international conference on virtual system and multimedia (VSMM), Dublin, IRELAND, Pages: 159-163. DOI: 10.1109/VSMM.2017.8346267.
- Koman, G., Kubina, M., Holubcik, M. and Soviar, J. (2018). Possibilities of Application a Big Data in the Company Innovation Process. In: Knowledge management in organizations, KMO 2018, Book Series Communications in Computer and Information Science, Volume 877, Page: 646-657, DOI: 10.1007/978-3-319-95204-8_54.
- Lee, S Y., Du, C J., Chen, Z H., Wu, H., Guan, K L., Liu, Y R., Cui, Y J., Li, W Y., Fan, Q. and Liao, W B. (2020). Assessing Safety and Suitability of Old Trails for Hiking Using Ground and Drone Surveys. In: ISPRS International journal of geo-information, Volume 9, Issue 4, DOI: 10.3390/ijgi9040221.
- Liang, M. and Delahaye, D. (2019). Drone Fleet Deployment Strategy for Large Scale Agriculture and Forestry Surveying. In: 2019 IEEE Intelligent Transportation Systems Conference (ITSC), Book IEEE International Conference on Intelligent Transportation Systems-ITSC, Auckland, New Zealand. Page: 4495-4500, DOI: 10.1109/ITSC.2019.8917235.
- Liu, B., Zhou, Q., Ding, R-X., Palomares, I. and Herrera, F. (2018). Large-scale group decision making model based on social network analysis: trust relationship-based conflict detection and elimination. In: European Journal of Operational Research. 275, Issue 2, s. 737–754, ISSN: 0377-2217.
- Liu, Y., Fan, Z. P. and Zhang, X. (2016). A method for large group decision-making based on evaluation information provided by participators from multiple groups, Information Fusion 29, s. 132–141, ISSN: 1566-2535.
- Lu, Y. and Shahabi, C. (2017). Efficient Indexing and Querying of Geo-tagged Aerial Videos. In: 25TH ACM Sigspatial international conference on advances in geographic information systems, Redondo Beach, CA, Pages: 1-10, DOI: 10.1145/3139958.3140046.
- Marquis, B. L. and Huston, C. J. (2009). Leadership Roles and Management Functions in Nursing: Theory and Application. China: Lippincott Williams & Wilkins, 654s, ISBN: 0-7817-7246-X.
- Mirk, D., Hlavacs, H. (2014) Using Drones for Virtual Tourism. Intelligent technologies for interactive entertainment, In: INTETAIN 2014, Book Lecture Notes of the Institute for Computer Sciences Social Informatics and Telecommunications Engineering, Chicago, IL, Volume 136, Page: 144-147, ISSN: 1867-8211, DOI: https://doi.org/10.1007/978-3-319-08189-2_21.
- Pai, P. F., Hong, Wei-Chiang, Chang, P. T. and Chen, C. T. (2006). The Application of Support Vector Machines to Forecast Tourist Arrivals in Barbados: An Empirical Study. In: International Journal of Management. Volume pp.375-385.
- Saafy, T. L. (2008). Decision making with the analytic hierarchy process. In: J. Services Sciences, Vol. 1, No. 1, 2008, s. 83–98, ISSN: 1753-1446 (Print).
- Sony, S., Laventure, S. and Sadhu, A. (2019). A literature review of next-generation smart sensing technology in structural health monitoring. In: Structural Control Health Monitoring, Volume 26, Issue 3, [Online]. [Retrieved September 20, 2021], https://doi.org/10.1002/stc.2321.
- Soviar, J., Holubcik, M. and Vodak, J. (2018). Regional Cooperation Ecosystem: Case of the Zilina Self-Government Region (Slovak Republic). In: Volume 10, Issue 7. DOI: 10.3390/
su10072219.
- Stankov, U., Kennell, J., Morrison, A M. and Vujičić, M D. (2019). The view from above: the relevance of shared aerial drone videos for destination marketing. In: Journal of Travel & Tourism Marketing, Volume 36, Issue 7: Future of Tourism Marketing, Pages: 808-822, DOI: https://doi.org/10.1080/105
48402019.1575787.
- (2021). Distribution of drone-based services worldwide in 2020, by activity. [Online]. [Retrieved September 12, 2021], https://www.statista.com/statistics/1200562/drone-service-activity-worldwide/, (b).
- (2021). Projected commercial drone revenue in Europe from 2016 to 2025. [Online]. [Retrieved September 10, 2021], https://www.statista.com/statistics/607794/commercial-drone-market-revenue-in-europe-projection/, (a).
- Stokes, D., Apps, K., Butcher, P A., Weiler, B., Luke, H. and Colefax, A P. (2020). Beach-user perceptions and attitudes towards drone surveillance as a shark-bite mitigation tool, In: Marine policy, Volume: 120, DOI: 10.1016/j.marpol.2020.104127.
- Supriatna, A., Lesmana, E., Aridin, L., Sukono and Napitupulu, H. (2019). Comparison between multiplicative Holt Winter and decomposition method in predicting the number of incoming international tourists to Indonesia. In: IOP Conference Series: Materials Science and Engineering, IOP Conf. Ser.: Volume 567, DOI: 10.1088/1757-899X/567/1/012047.
- Verma, D. (2009). DECISION MAKING STYLE: Social and Creative Dimensions. New Delhi: Global India Publications, 306s, ISBN: 9-3802-2830-9.
- Worakuldumrongdej, P., Maneewarn, T. and Ruangwiset, A. (2019). Rice Seed Sowing Drone for Agriculture. In: 19TH International conference on control, automation and systems (ICCAS 2019), Book SeriesInternational Conference on Control Automation and Systems, South Korea, Page: 980-985, DOI: 10.23919/ICCAS47443.2019.8971461.
- Zhu, Y., Chen, P-Y. and Zhao, W. (2014). Injured workers in china: injustice, conflict and social unrest, In: International Labour Review, 153, Issue 4, s. 635–647, ISSN: 1564-913X.