Introduction
Climate change is among the biggest threats facing humanity nowadays. Its potential impact on global food security is of great concern for policymakers, stakeholders, and researchers alike. The Intergovernmental Panel on Climate Change (IPCC) has estimated that the world’s average surface temperature will rise from 1.5°C to 4.5°C by the end of the century, the exact value depending on the level of greenhouse gas emissions. This increase in temperature combined with variations in precipitation patterns, will have significant influences on agriculture, impacting everything from crop yields to pest infestations.
Agriculture is an important sector of the global economy, as it makes available employment to billions of people and it supplies food for the entire world’s population. United Nations Food and Agriculture Organization (FAO) estimates that the global food production will need to increase by 70% until 2050 to meet the demands of our growing population. Yet, climate change is likely to reduce crop yields in many regions of the world, posing a big challenge to global food security.
Romania has a very fertile soil in central and eastern regions, as well as a favorable climate. These two factors combined with many more make Romania a significant producer of wheat, corn, sunflower seeds, and other crops. However, in the last decade, Romania’s agricultural sector has suffered substantial changes. The country has shifted towards market-oriented agricultural policies. The sale of state-owned farmland, increased investment in modern agricultural technologies, and an emphasis on exports have all influenced the growth of this sector. Nevertheless, the sector also faces numerous challenges, for example: low productivity, a significant deficiency of investment in infrastructure, and the effects of climate change. Despite the above-mentioned challenges, Romania’s agricultural sector represents a significant contributor to the country’s economy, as it comprises almost one-fifth of the country’s workforce.
Nowcasting is a statistical technique that uses real-time data to predict present or near-future values of a variable. Nowcasting can be used to deliver timely and precise information on the potential influence of climate change on cereal yields, which could enable both policymakers and stakeholders to develop adaptation approaches that can ease the effects of climate change on food production.
Regression analysis is a statistical method used to model the relationship between two or more variables. In the framework of nowcasting the effect of climate change on agricultural yields, regression analysis can be used to create models that predict cereal yields in relationship with historical data on temperature, precipitation, and other factors. These models can be used to nowcast the possible effect of climate change on cereal yields under different circumstances.
The scope of this research paper is to explore how regression analysis can be used to nowcast the impact of climate change on cereal yields in Romania. We will investigate historical data on temperature, precipitation, cereal yields and other variables to develop regression models that can foresee future yields based on climate projections.
The paper is designed as the following: chapter 2 provides a useful and insightful literature review on the effect of climate change on agricultural yields and how regression analysis was used for nowcasting. In chapter 3, we describe the data sources and methods used in this research paper. In Chapter 4, we present the results of our regression analysis, and also debate the implications for policymakers and stakeholders. Lastly, in chapter 5, we conclude our paper and make some recommendations for future research.
Literature Review
The most recent number of people suffering from famine as of 2022 is 828 million, yet the Food and Agricultural Organization (FAO) states that this number is not truthful, and a closer number to the reality figure would be 2.3 billion of people suffering from, not extreme but still, dangerous level of food security. Since climate change is expected to have an increasing significant impact on global food security, many studies have explored the relationship between climate change and agricultural yields using a variation of approaches.
The multiple regression models enhance regression analysis as described by (Titan, Ghita, & Trandas, 2003), (Constantin, 2006) and (Lefter, 2004). Also, the multiple regression model can provide a more accurate output than the unifactorial regression model (Goschin & Vatui, 2002). Regression analysis is extensively used to model the relationship between climate variables and agricultural yields. Quite a few studies have used it to determine the influence of climate change.
For example, (Lobell & Gourdji, 2012) used regression analysis to study the influence of temperature and precipitation on global crop yields, and they found that an increase in temperature resulted in a corresponding decrease in crop yields, while precipitation had a positive impact. The study is particularly intriguing as it emphasized the benefits of adaptation strategies: enhanced irrigation systems, or the usage of heat-tolerant crops.
On NASA official site, we can find the abstract of another study by (Rosenzweig, et al., 2013) assessing the influence of climate change by using seven global gridded crop models (GGCMs) for a corresponding set of simulations of global crop yields taking into consideration evolving climate conditions. The paper results indicate a strong negative influence of climate change on maize, particularly at higher levels of warming and at low latitudes where most developing countries are concentrated.
Recently, a study by (Pineda, et al., 2019) used regression analysis to explore the projected changes in the climate of five conifer species in Brazil. The study was based on normal climatic variables from 1961 to 1990, and resulted in 3 scenarios centered on years 2030, 2060 and 2090. The study found that habitat loss could total up to 46–77% and conifer species at the highest elevations would suffer the greatest losses.
A new similar research paper addresses the same topic; (Kumar, Sahu, Kumar, & Ansari, 2021) examined the influence of climate change on cereal production in a range of lower-middle-income countries throughout 1971-2016. The study findings are interesting: there is a negative relationship between temperature and cereal production, yet there is a positive relationship between other climate variables, i.e., rainfall and CO2 and cereal yield.
Another paper researching the crop production in relationship with climate change, energy consumption, CO2 emissions, and eco-environmental factors, concluded that environmental and economic factors in Turkey are critical for cereal yield. (Köprücü & Acaroğlu, 2023) used a time-series autoregressive distributed lag model and spectral causality relationships with data between 1960 and 2019. There are some interesting findings: energy consumption has a positive influence on cereal yield, climate change has varying effects, while CO2 emissions has a negative influence.
A bibliometric research paper provides insights related to the effects of climate change on quality characteristics of cereals. (Akın , Eyduran, Rakszegi, Yıldırım, & Roch, 2023) constructed a thought-provoking paper which highlights that although this subject has thrived in recent years with noteworthy spikes in 2018 and 2020, the literature on the field is still fragmented. Taking everything into consideration, the literature suggests that both regression analysis and nowcasting are valuable tools for forecasting the influences of climate change on agricultural yields. This approach can provide valuable insights that would help policymakers and stakeholders in developing effective and efficient strategies to mitigate climate change impact.
Methods
This chapter presents the methodology used in the current research paper. The research purpose is to apply regression analysis to predict the influence of climate change on cereal yields in Romania. The following sections provide a detailed description of the data sources, variables, model development, and statistical techniques employed in the study.
The research utilizes a comprehensive dataset comprising historical data on average temperature, average precipitation, fertilizer consumption (% of fertilizer production), agriculture, forestry, and fishing, value added (% of GDP), employment in agriculture (% of total employment), agricultural irrigated land (% of total agricultural land) and the cereal yield between 2001 and 2021. The data were collected from (World Bank, 2023) (INS, 2023).
The dependent variable in the study is cereal yield, measured as kilograms per hectare of harvested land, includes wheat, rice, maize, barley, oats, rye, millet, sorghum, buckwheat, and mixed grains.
As independent variables, we have included: mean temperature (Celsius), precipitation(mm), fertilizer consumption (% of fertilizer production), agriculture, forestry, and fishing, value added (% of GDP), employment in agriculture (% of total employment), and agricultural irrigated land (% of total agricultural land). Variables were standardized for better transparency and clarity in our regression model. Since some variables have one or two missing values mainly for years 2020 and 2021, exponential smoothing was used to estimate them. Exponential smoothing is a method that considers the weighted average of preceding values to forecast future values. In our case, we used the Holt’s linear method, which combines both the level and trend components. The missing values estimated were the following: for fertilizer consumption (% of fertilizer production)- year 2021, employment in agriculture (% of total employment) – years 2019, 2020 and 2021 and agricultural irrigated land (% of total agricultural land)- year 2021.
Regression analysis is employed to establish the influence of the independent variables upon cereal yields. We have chosen to conduct a time series analysis considering the nature of the data and research objectives. The goodness-of-fit will be assessed using statistical indicators such as the R-squared, adjusted R-squared, and p-values. In addition, diagnostic tests, including residual analysis, normality tests, and heteroscedasticity tests, will be accomplished to guarantee the validity of the model.
In conclusion, the research paper utilizes regression analysis as the primary methodology to nowcast the impact of climate change on cereal yields. The study employs a comprehensive dataset, develops regression models, applies suitable statistical techniques, evaluates model performance, and conducts sensitivity analysis to ensure the validity and reliability of the findings.
Results
The multiple R value, in this case, is 0.81. This value indicates a strong positive correlation between the variables. The R-squared value is 0.66, which indicates the share of variance in the dependent variable that can be explained by the independent variables. In our case, approximately 66.03% of the variability in the dependent variable is influenced by the 6 chosen independent variables. The significance F in our case is 0.009, which is less than the conventional significance level of 0.05. Consequently, we reject the null hypothesis and conclude that the multiple regression model is statistically significant.
Table 1. Regression analysis summary output
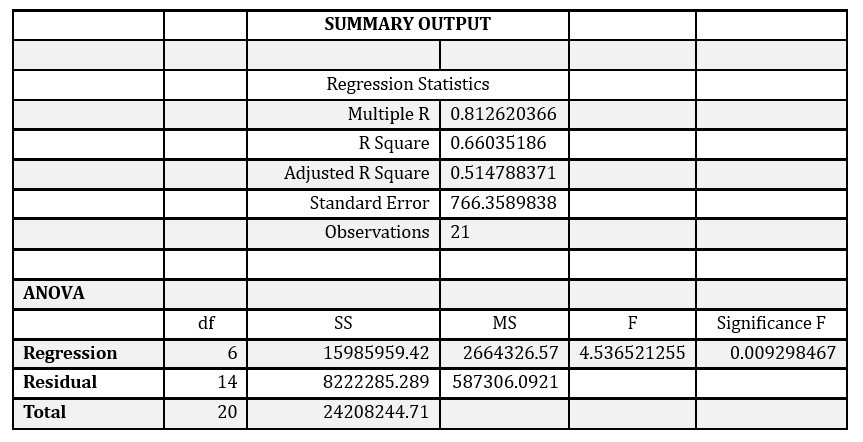
The multiple regression analysis is the following:
Y = 7269.072013+ 112.010804* X1 -0.64120504* X2 + 9.240572547 * X3 + 296.6105551* X4 – -232.2038196* X5 – 281.6867222 * X6 + ε
Where:
- Y represents the dependent variable- Cereal yield (kg per hectare)
- X1 represents the average temperature (Celsius).
- X2 represents precipitation(mm).
- X3 represents fertilizer consumption (% of fertilizer production)
- X4 represents agriculture, forestry, and fishing, value added (% of GDP)
- X5 represents employment in agriculture (% of total employment)
- X6 represents agricultural irrigated land (% of total agricultural land)
In order to test the validity of multiple regression model, a global test must be used, so we formulate the following null and alternative hypotheses:
H0 = β1 = β2 = β3 = β4 = β5 = β6 =0
H1 = not all β coefficients are equal to 0
To test the null hypothesis, we perform F test, and we have a value of 4.536521255. The critical value of F, at a significance level of 0.05 is 2.85. In our case, calculated F value is greater than the critical F value; the observed relationship is statistically significant. This indicates that we have evidence to reject the null hypothesis, which assumes that there is no relationship between the independent variables and the dependent variable.
From the above information, we can draw the following conclusion: the independent variables selected for the regression model are significant for the cereal yield in the analyzed period of 2002- 2021. Diagnostic tests, including residual analysis, normality tests, and heteroscedasticity tests, were performed and we have evidence that the model is valid and statistically significant.
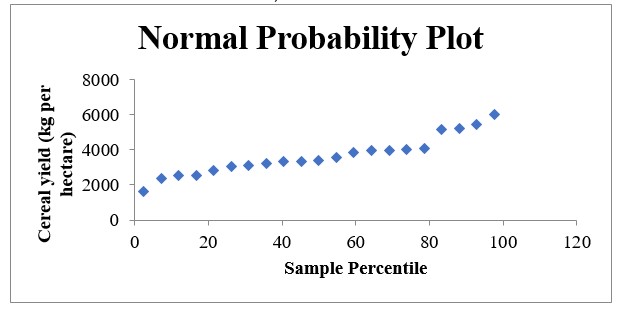
Figure 1. Normal Probability Plot
This model provides us with lots of useful information about the effect of climate change on Romanian agriculture. In order to have a more accurate perspective on the influences of climate change on agriculture in Romania, it is important to consider other variables in addition to climate variables. Despite the fact that climate change unquestionably plays a significant role in the agricultural outcomes, the relationship between climate and other factors can provide a more comprehensive context. One important influence to study is agricultural land use and management practices. The practices used in land management can have a considerable impact on crop yields and total agricultural productivity. Variables such as: crop rotation, irrigation systems, fertilizer application, and pest management methods can either improve or hinder the adaptive ability of the agricultural system to climate change.
Additionally, socio-economic influences should be taken into account too, like: resources availability, technological progresses, funding, infrastructure, and policy agendas. Socio-economic factors can impact the capacity of both farmers and agricultural societies to adapt to changing macroclimate conditions. For example, farmers with limited education might face difficulties in applying strategies to adapt or invest in resilient practices.
Moreover, the addition of market dynamics is vital in constructing a better model for Romania. Agricultural yields and the success of farming activities are influenced by the market trends, like: demand-supply equilibrium, price variations, and trade policies. A better understanding of how climate change interacts with market trends can provide new and insightful information into the economic feasibility of different agricultural practices, and possible adaptation policies.
Another important influence to consider is represented by the ecosystem context, such as: pollination, natural pest control, and soil fertility. Climate change can either have a direct or indirect impact on these ecological procedures, which in turn influence agricultural yields. Consequently, investigating the relationship between climate change, ecosystem practices, and agricultural productivity can offer valuable insights.
In conclusion, in order to gain a more accurate perspective on the influences of climate change on agriculture in Romania, it is critical to consider variables beyond climate alone. Including factors such as land management, socio-economic settings, market trends, and ecological framework into the analysis can provide a complete understanding of the complex relations and challenges in the agricultural sector. A more cohesive approach is crucial for developing effective and efficient strategies and policies that would address the multidimensional influences of climate change on agriculture in Romania.
Conclusions
In the current research paper, we have used regression analysis to nowcast the influence of climate change on Romanian cereal yields. Through our study, we have confirmed that climate variables play a role in shaping agricultural yields. The regression model has allowed us to calculate the relationship between climate variables and cereal productivity, offering us better understandings into the possible influences of climate change.
Our research has emphasized the importance of considering multiple factors in addition to climate variables in the case of Romania. By including variables such as land management, socio-economic environment, market trends, and ecological context, a more comprehensive understanding of the complex relations in the agricultural sector could be provided. This tactic is critical for developing effective strategies for adapting and procedures to mitigate the effects of climate change on agriculture.
It is important to acknowledge the limitations of our research. While regression models offer valuable insights, it is important to recognize that it cannot capture all the complexities and uncertainties related with climate change. We encourage future research to continue exploring and incorporating additional factors, such as technological progresses, policy agendas, and social dynamics.
In conclusion, our research paper has contributed to the growing body of knowledge on the impact of climate change on agricultural yields. This study, along with further research papers, can help the development of operative adaptation strategies and policies to ensure the resilience and sustainability of agricultural systems in the face of climate change.
References
- Akın , M., Eyduran, S. P., Rakszegi, M., Yıldırım, K., & Roch, J. M. (2023). Chapter 16 – Statistical modeling applications to mitigate the effects of climate change on quality traits of cereals: A bibliometric approach. Conventional and Molecular Breeding, 381-396.
- Constantin, C. (2006). Marketing information system: analiza si prelucrarea marketing date: application in SPSS. Brasov: Infomarket Publishing House.
- Goschin, Z., & Vatui, M. (2002). Statistica. Bucharest: ASE Publishing House.
- (2023). INS. Retrieved from Tempo online: http://statistici.insse.ro:8077/tempo-online/
- Köprücü, Y., & Acaroğlu, H. (2023). How cereal yield is influenced by eco-environmental factors? ARDL and spectral causality analysis for Turkey. Cleaner Environmental Systems.
- Kumar, P., Sahu, N. C., Kumar, S., & Ansari, M. A. (2021). Impact of Climate Change on Cereal Production: Evidence from Lower-Middle-Income Countries. Research Square.
- Lefter, C. (2004). Cercetare de marketing. Brasov: Infomarket Publishing House.
- Lobell, D. B., & Gourdji, S. M. (2012). The Influence of Climate Change on Global Crop Productivity. Plant physiology.
- Pineda, E. G., Romero, C. S., Rodríguez, J. O., García, A. B., Sánchez, X. M., Cisneros, R. L., . . . Rehfeldt, G. E. (2019). Suitable climatic habitat changes for Mexican conifers along altitudinal gradients under climatic change scenarios. Ecological Applications.
- Rosenzweig, C., Elliott, J., Deryng, D., Ruane, A. C., Müller, C., Arneth, A., . . . Jones, J. (2013). Assessing agricultural risks of climate change in the 21st century in a global gridded crop model intercomparison. Proceedings of the National Academy of Sciences.
- Titan, E., Ghita, S., & Trandas, S. (2003). Statistica Aplicata. Bucharest: Meteor Press.
- World Bank. (2023). World Bank. Retrieved from Climate Change Knowledge Portal: https://climateknowledgeportal.worldbank.org/download-data