Introduction
Over the previous years, higher education institutions were offering courses online for students as one of their academic plan’s components. Online courses are connecting a variety of tools such as social networking and curriculum available online through different e-learning platforms such as Moodle, Blackboard, etc. The active involvement of many students in these courses who participate individually according to their achieving goals, previous knowledge, and skills, is a major factor for success. (McAuley et al., 2010). Education is one of the most dominant means of providing social equality as well as an accomplishing experience of an individual’s life. The basic tools require computer screens connected to the Internet so lectures could be delivered, the course content is visible for the students to read, audio devices are required to deliver the course content as well as interactions and discussions via video output. Many academic institutions used Zoom as their platform to deliver all course from video and audio tools as well as Moodle for the whole course content and syllabus from power points, pdf files, assignments, and quizzes.
E-learning is defined as the learning tool delivered via computers for the intention to develop education (Mayer, 2003) that has expanded quickly over last years with the upgrades in technology and the integration of IT with educational curricula. (Smart and Cappel, 2006). E-learning is essential to develop good calibers, well educated, as well as the rapid need of society for long term learning that is delivered in an appropriate form. Learning online can take many forms from fully online, blended or web-assisted, no matter what the delivery technique of learning, however different tools are available at the students’ and faculty members’ hands. (Alshehri, 2017). In fact, online learning is a tool that is globally used for learning with standards and it is not required from the learner or the student to attend the class physically and is suitable for students who are interested in studying while they have other commitments. (El-Ebiary et al., 2016).
E-learning now is not a strategy to overcome just learning from distance, it is now a way to adapt to learning as per personal schedule especially after the pandemic of COVID-19 2020. In this study, students used Moodle that allowed them to interact with the lecturers using audio and video, submit assignments and quizzes, upload and/or download files, have feedback on their grades and have access to the content anywhere anytime. The development of such platforms or applications made the online learning a more interactive way for students to learn at their preference. In recent years, interventions using technologies based on the Internet have allowed electronic learning as a major element in education (Aşkar and Halici, 2004). Online learning can provide academic institutions with a reduced cost, and adaptable tool to grow globally (Casey, 2008).
Students enjoyed this way of online delivery and sometimes it seemed to be more effective than face to face teaching. While students have an online meeting with their instructors, this screen sharing approach may help the students feel less exposed when asking for help compared to a physical class within groups. The students may prefer a quicker way to ask for help such as posting their questions on the online chat. This way of asking questions may allow the student to feel more comfortable than raising up their hands in front of their colleagues in a computer lab. Similar recent studies have reported (Davison, 2020) that student interaction was motivated through the use of online chat functionality. (Dwivedi et al., 2020).
In light of the dramatic increase in the use of online courses, in higher education especially after the pandemic of COVID-19, the current study deals with identifying key determinants of student satisfaction in an online learning environment namely online tutorials flexibility, online tutorials quality and technology quality. It also investigates the impact of the student satisfaction on their commitment towards the institution and the learning process. Several studies tackled student satisfaction and its key determinants but one of the recent studies investigated factors that were relevant to the online learning environment students have experienced through the pandemic in Egypt. The study by Harsasi and Sutawijaya (2018) included four variables namely course structure, online tutorials flexibility, online tutorials quality and technology quality but online tutorials quality had less impact on student satisfaction. Therefore, the proposed model investigates three variables namely course structure, online tutorials flexibility and technology quality according to the pandemic period in Egypt.
This study sheds light on some of the determinants of students’ satisfaction and the impact of students’ satisfaction on their commitment online. This is to know which determinants are the most effective on the students’ satisfaction in the online learning process and to explore the influence of their online satisfaction on their commitment towards their institution. Technology becomes a crucial part in our life. E-learning, Internet and computers have been used extremely in the learning process during COVID-19 (Khan and Raad, 2020). This study may have a marked impact on online course satisfaction and commitment. Furthermore, this study may affect not only academic courses but have paved the way for academic institutions and professional training centers to measure the behavior of students after COVID-19. Instructors and researchers can use this study and the preliminary model for the benefit of their research and instruction specially to understand and improve the behavior of students through the online learning process.
Literature Review
There are a number of studies that have investigated student satisfaction and commitment in relation to e-learning. A study found that the less student satisfaction the more the failure of any e-learning implementation, and there are many determinants that affect student satisfaction in e-learning. Moreover, Hermans et al. (2009) suggested that student satisfaction has a vital role to endorse higher education successfully. These authors tested the relationships among different factors that influence student satisfaction in an online learning environments. (Alshehri, 2017)
A definition for satisfaction and commitment needs to be clarified. Student satisfaction is an indicator of whether learners or students are satisfied with their learning experience. (Li et al., 2016). In the academic context of higher education, student satisfaction plays an important role in universities’ success (Firdaus, 2006), and the concept of satisfaction has been extended to evaluations of higher education services. Elliott and Healy (2001) state that the concept of students’ satisfaction is a short-term behavior and a result of students’ experiences with their educational services provided by their institution.
Previous research has found that organizational commitment which is defined as a person’s commitment to the organization they work in is related to their career commitment (Womack, 2016) , which means that a person’s commitment to their organization is an indicator of their commitment to his or her career. The concept of organizational commitment is altered to state the commitment that the student feels towards his or her institution. (Womack, 2016)
Commitment can be viewed as future-oriented self-regulation where one sets the goals based on their identity and then behaves in a way to achieve the goals bounded by their identity. (Human-Vogel and Vogel, 2015).
A tool was developed to measure employee commitment based on a three-component model of affective, continuance and normative commitment. Affective commitment refers to the attachment and involvement to the organization, Continuance commitment refers to the costs assessed of leaving the organization and Normative commitment refers to the feeling of supporting the organization and remaining in it. (Wilson et al., 2016). The aim of a recent study was to explore factors that have an effect on student satisfaction in online learning environment based on previous research by (Sun et al., 2008) and (Eom et al., 2006).
The impact of course structure on student satisfaction
Course structure is viewed as an important variable that supports the success of online learning. According to Moore (1991), the course structure “expresses the rigidity or flexibility of the program’s educational objectives, teaching strategies, and evaluation methods” and the course structure describes “the extent to which an education program can accommodate or be responsive to each learner’s individual needs.”
Course structure has two parts which are course objectives and course infrastructure. Course objectives are concerned with the course curriculum that includes the topics to be learned, the assignments to be completed, the class participation online, group projects and so on. Those parts affect the student satisfaction level and their learning outcomes. According to Eom et al. (2006), it was stated that course structure will be correlated to student satisfaction and perceived learning outcomes when the course material is organized in a logical sequence and that a clarified course objectives will lead to high student satisfaction levels. (Eom et al., 2006)
Hypothesis 1: Course structure has a significant impact on student satisfaction.
The impact of online tutorials flexibility on student satisfaction
Satisfaction and participation of students are facilitated because of the flexibility in time, location and methods found in online learning courses. (Arbaugh, 2002) (Arbaugh, 2000) (Berger, 1999). Moreover, the exclusion of any traditional classroom environment enables more interaction that promotes cooperative learning. Students can communicate online anytime anywhere with no limits. In addition, the virtuality available online reduces the discomfort of face-to-face communication that is available in traditional classrooms. Students have the freedom to express their opinions and ask questions through discussion groups without restraint. “The definition of e-Learning course flexibility is learners’ perception of the efficient and effects of adopting e-Learning in their working, learning, and commuting hours”. (Sun et al., 2008)
Hypothesis 2: Online tutorials flexibility has a significant impact on student satisfaction.
The impact of technology quality on student satisfaction
Several researchers suggest that the technology quality and Internet quality affect satisfaction in e-Learning (Piccoli et al., 2001) (Webster, 1997). Students will be willing to adopt an e-learning software or tool with few barriers and their satisfaction will be improved. (Amoroso and Cheney, 1991). Online learning may involve learning and discussion using tools such as video conferencing. Therefore, quality of the technology and the Internet are important factors in e-Learning (Piccoli et al., 2001) (Webster, 1997). In addition, a research study conducted by Webster (1997) studied learning effects on the technology applied in a distance learning of 247 students and found that quality and reliability of technology influence learning effects. “The definition of technology quality is the learners’ perceived quality of IT applied in e-Learning (such as microphones, earphones, electronic blackboards, and so on)”. (Sun et al., 2008)
Hypothesis 3: Technology quality has a significant impact on student satisfaction.
The impact of student satisfaction on commitment
In an academic context, the knowledge about teachers’ attitudes needs more attention as the universities’ performance depends on their staff which must be satisfaction and commitment (Tsui and Cheng, 1999). In the same manner, students are other academic entities and their satisfaction and commitment require more attention. An academic employee will feel more satisfied, more committed to his or her academic institution and will develop the output of their universities (Sami et al., 2012). Some research studies support the contention that satisfaction predicts commitment and that satisfaction is positively related with affective and normative commitment, but not with continuance commitment. (Bashir and Ganai, 2019)
Hypothesis 4: Student satisfaction has a significant impact on student commitment.
Research Hypotheses
The main aim of this study is to explore the impact of each of those variables on student satisfaction in an online learning environment and the mediating effect of student satisfaction between independent variables and student commitment.
Based on the previous study, this research adopted three variables that have influence on student satisfaction online. These variables are course structure, online tutorial flexibility and technology quality. To achieve the research objectives, the hypotheses were developed as follows:
H1: Course structure has a significant impact on student satisfaction.
H2: Online tutorials flexibility has a significant impact on student satisfaction.
H3: Technology quality has a significant impact on student satisfaction.
H4: Student satisfaction has a significant impact on student commitment.
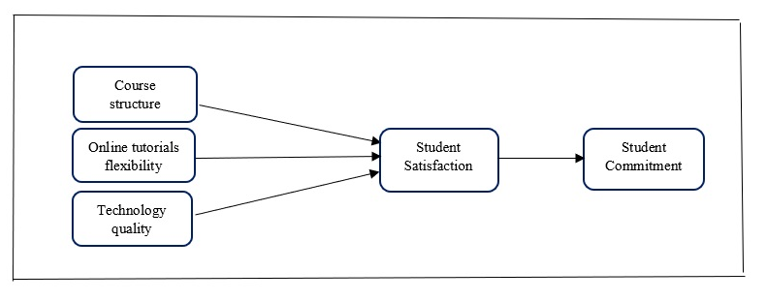
Figure 1: The proposed research model
Methodology
This research was based on the use of three independent variables (course structure, online tutorials flexibility and technology quality) and one mediating variable (student satisfaction). In addition, the dependent variable student commitment was used based on another previous study by Wilson et al. (2016).
The observed data were collected using a survey methodology. The target population were students from higher education institutions, and due to the pandemic of COVID-19, the sample size was 410 students inside one of the top accredited universities in Egypt. Participants of the study were students from different colleges inside the university. The students are those who pursued Second Semester 2020/2021 and who took the experiment of online courses through the pandemic of COVID-19.
A questionnaire was distributed to the students online through a link on SurveyMonkey. The responses to the questionnaire were 410 students from different colleges such as College of Management and Technology, College of International Transport & Logistics, College of Language & Communication, College of Computing & Information Technology and College of Engineering & Technology.
The survey instrument included the independent variables and the mediating variable were adopted from Harsasi and Sutawijaya (2018) while the dependent variable was adopted from Wilson et al. (2016). Each variable’s indicators are measured using 5 points Likert scale with the following scale:
1 = Strongly disagree
2 = Disagree
3 = Neither agree nor disagree
4 = Agree
5 = Strongly agree
Each variable has their own indicators as shown in Table 1.
Table 1: Indicators of each variable
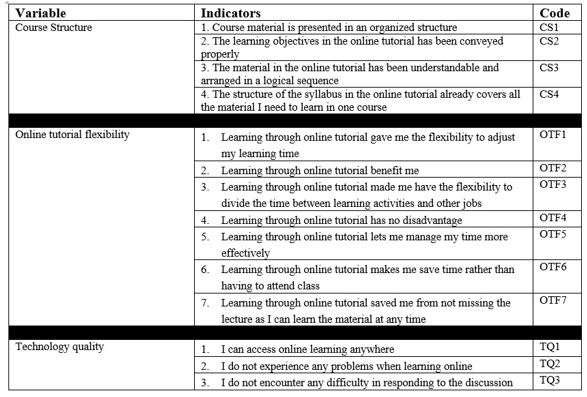
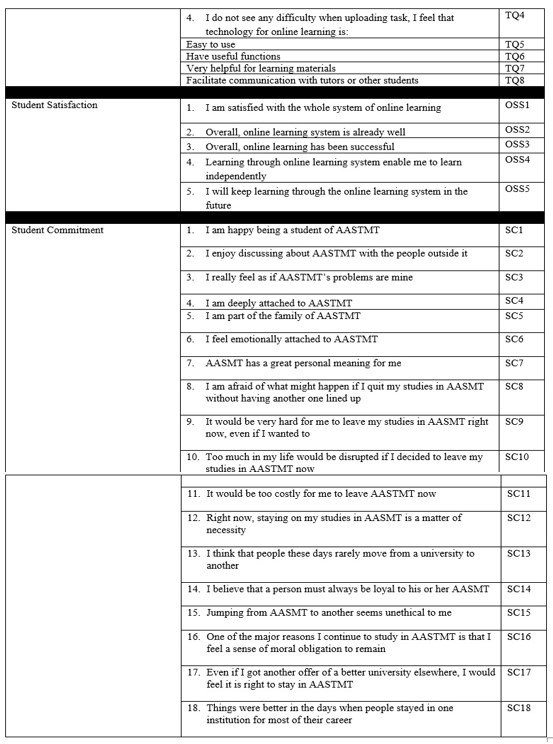
Results and Discussion
This section presents the data analysis part of the study. The analysis of this paper was done using the statistical package for social sciences (SPSS V26) for basic descriptive statistics, and (SmartPLS 3.2.7) for SEM-PLS modeling. It is divided into four sections respectively: Data preparation, measurement model for reliability and validity, several descriptive statistics and bivariate correlations are constructed and finally the structural model for hypothesis testing and mediation analysis.
Data preparation
This examination is important in any quantitative research and specifically when using SEM for data analysis (Hair Jr et al., 2017). The issue of missing data was inspected and found that some indicators have missing percent greater than 5%, so, according to Hair Jr et al. (2017) the best scenario is the case-wise deletion. Considering outliers, there are no outliers detected in our dataset.
The data distribution is not an issue, since the SEM-PLS is a non-parametric tool that does not assume normal data ((Hair Jr et al., 2017) (Garson, 2016). Structural equation modeling is an analysis approach that tests both the measurement model and path that helps to develop more realistic assumptions (Abdi, 2010) (Hair Jr et al., 2017). Hence, this study focuses on examining the prediction of the dependent variable, the mediation analysis, and the effect of independent variables, which make PLS method the most appropriate in this study.
Assessing the Measurement Model
The measurement models, which are also known as the outer models, describe the relationships between the constructs and their items. The assessment of the reflective measurement models in PLS-SEM requires evaluating the internal consistency reliability, convergent validity, and discriminant validity (Hair et al., 2011) (Nachtigall et al., 2003) (Xiong et al., 2015) (Garson, 2016). The internal consistency reliability examines whether all of the indicators associated with a construct are actually measuring it (Pallant and Manual, 2010). There are different ways to measure internal consistency. Cronbach’s alpha is a statistical measure that is the most used for this purpose. The accepted value of Cronbach’s alpha is 0.7; all values of Cronbach’s alpha in the table (2) were above 0.7.
Table 2: Results of the Measurement Model 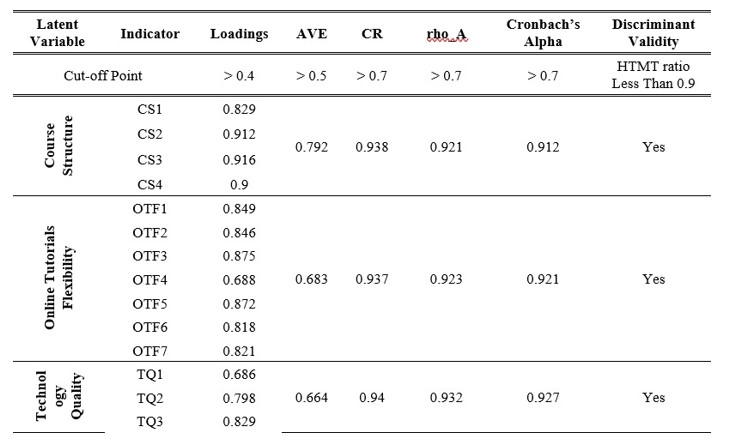
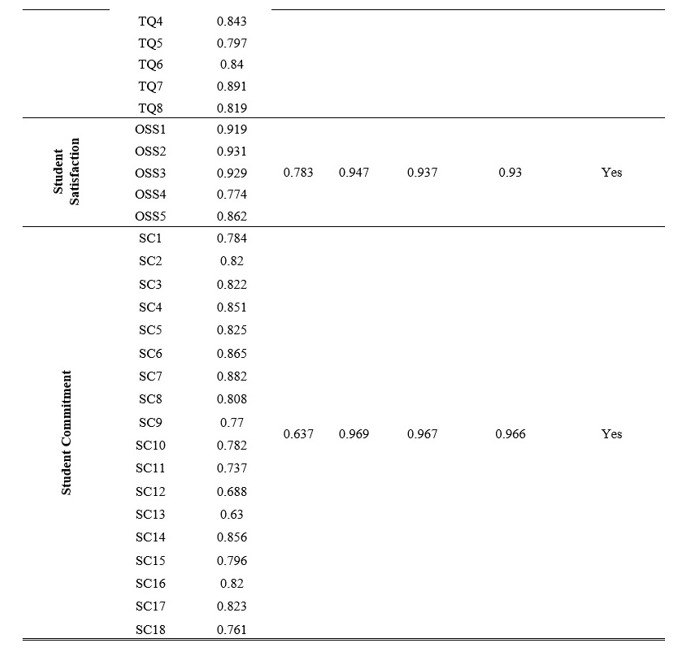
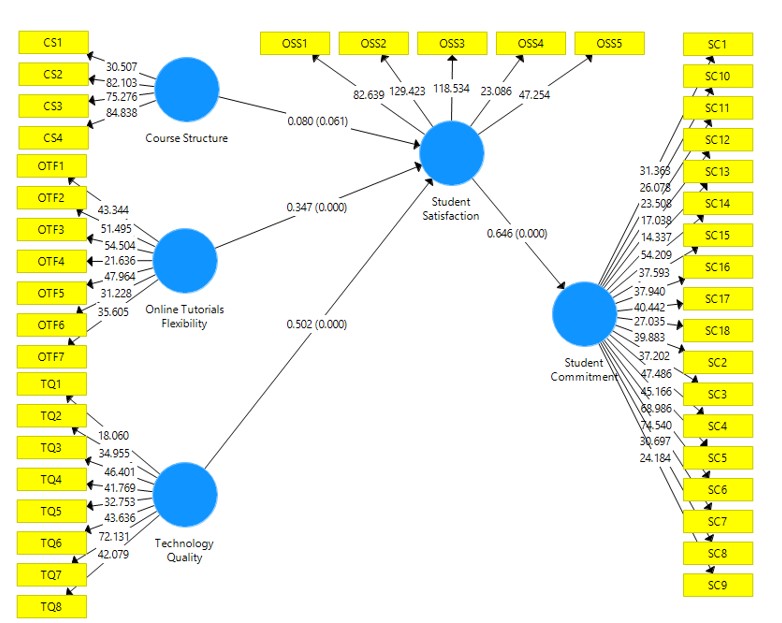
Figure 2: Path Coefficints with Corresponding P-values
The AVE is a standard measure used to establish convergent validity. All of the constructs in Table (2) have AVE scores higher than 0.50. After establishing the convergent validity, it is time to examine the discriminant validity. Discriminant validity examines how much a construct differs from other constructs.
Descriptive Statistics and Multiple Correlations
Table (3) shows the descriptive statistics of the variables. It can be shown that course structure has mean and standard deviation as with direct medium correlation to both online student satisfaction , and student commitment .
Table 3: Descriptive Statistics and Multiple Correlations 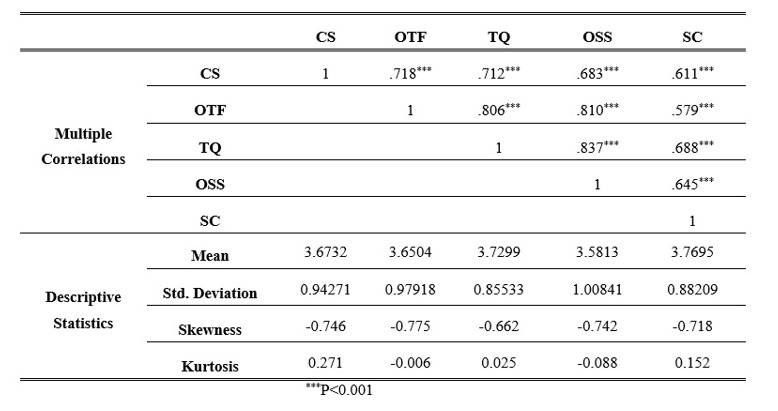
Online tutorial flexibility has mean and standard deviation as , with direct strong correlation to online student satisfaction , and direct medium correlation to student commitment . Technology quality has mean and standard deviation as , with direct strong correlation to online student satisfaction , and direct medium correlation to student commitment . Online student satisfaction with mean and standard deviation , and student commitment with mean and standard deviation correlate to each other with direct medium correlation as .
Assessing the Structural Model
Researchers provided guidelines for evaluating and reporting the structural model, including path coefficients, collinearity, coefficient of determination (R2), effect size (f²), predictive relevance (Q2), and goodness of fit criteria; table (4) summarises those criteria.
Table 4: Criteria of Structural Model Assessment 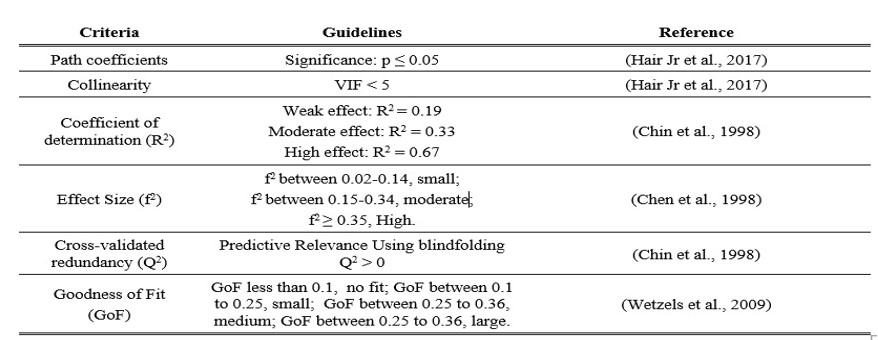
Path coefficients refer to the estimates of the relationships between the model’s constructs. When assessing the PLS path, studies should report path coefficients beside the significance level, t-value,
and p-value. According to Hair Jr et al. (2017) , every significant coefficient eventually depends on the standard error, which is usually obtained by using bootstrapping. Moreover, the strength of path coefficients can be measured through direct and indirect effects. We are interested in some direct and indirect effects to test the hypothesis underlying this research.
The hypothesis testing has been done to understand the signs, size, and statistical significance of the estimated path coefficients between the constructs. Higher path coefficients suggest stronger effects between the predictor and predicted variables. The significance of the supposed relationships has been established by measuring the significance of the p-values for each path with threshold equalling p ˂0.05. The p-values and inference of hypotheses, as well as the confidence level for each estimate, are shown in Table 4. The findings of this study reveal that all hypotheses are accepted except H1 and H5.
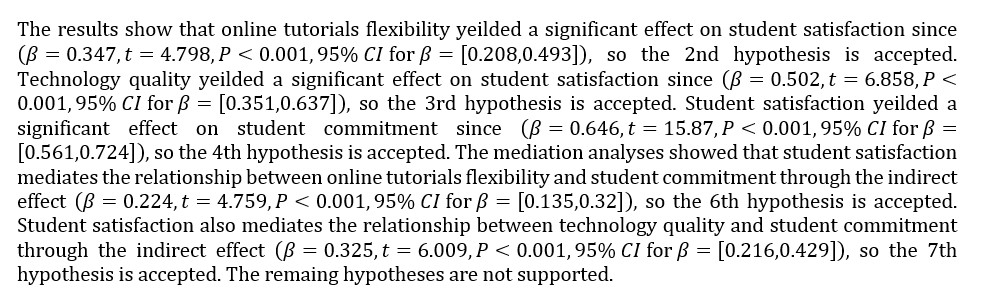
Table 5: Hypothesis Testing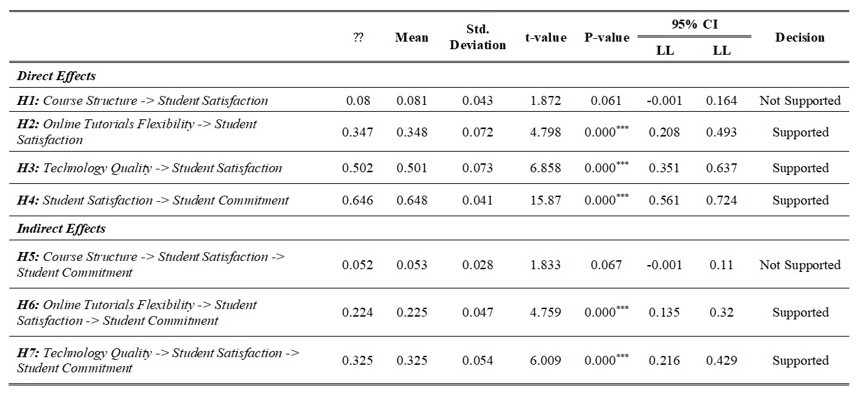
Table 6: Structural model assessment measure
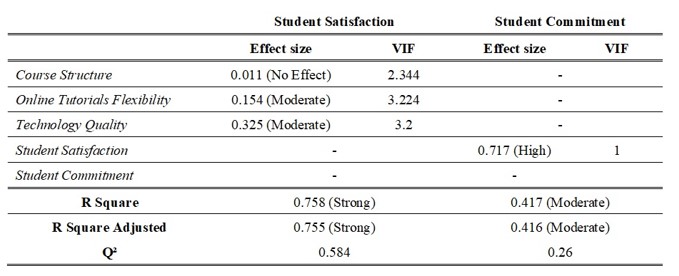
Coefficient of determination ( ) refers to the effect of independent variables on the latent dependent variables (Hair et al., 2011),which is one of the quality measures of the structural model (Hair et al., 2014). Researchers have used a different cut-off of value. For example, Hair et al. (2011) in marketing research described that values of 0.25, 0.50, or 0.75 are low, moderate, or high, respectively. In business research, Chin et al. (1998) suggested that with 0.19, 0.33, or 0.67 are low, moderate, or high, respectively. The results of R Square are reported in table (6), the R-Square of student satisfaction equals 76% which means that about 76% of the variations in student satisfaction are explained by the variations in the selected independent latent variables. Additionally, the R-Square of student commitment equals 42% which means that about 42% of the variations in student commitment are explained by the other latent variables.
The effect size is the measure of how much impact the endogenous construct will have if an exogenous construct was removed from the model. Table (4) shows the cut-off for the effect sizes. It can be noticed that course structure has no effect on student satisfaction, while both online tutorials flexibility and technology quality have moderate effect on student satisfaction. Finally, student satisfaction has high effect on student commitment.
value indicates the model’s out-of-sample predictive power. When a model is said to have a predictive power or predictive relevance, it means that it can accurately predict data not used in the model estimation. The value is calculated through running a blindfolding procedure. Before running this procedure, an omission distance (D) must be specified.
Researchers suggest specifying a D between 5 and 10 while being careful that the sample size divided by the selected D would not produce an integer. The omission distance indicates that while running the blindfolding procedure, every x data point of the items will be omitted and then predicted, with x being the specified D value (Hair et al., 2016). Based on the recommendation from the literature, an omission distance of 10 was selected to examine the predictive power of the model. Table (6) presents the values obtained from the analysis. The values of for both student satisfaction and student commitment are higher than 0, so it can be safely concluded that the model has a good predictive relevance.
Tenenhaus et al. (2005) proposed the Goodness of Fit (GoF) as a global fit indicator; it is the geometric mean of both the average and the average variance extracted of the endogenous variables. The GoF index can be calculated as follows:
.
The criteria of GoF for deciding whether GoF values are not acceptable, small, moderate, or high to be regarded as a globally appropriate PLS model, have been given in table (3). According to these criteria and the value of the GOF ( ), it can be safely concluded that the GoF model is large enough to consider valid global PLS model.
Conclusion
The present study used structure equation modeling to investigate the behavior of students’ commitment towards e-learning during the pandemic of COVID-19 and the key determinants of student satisfaction in online learning environment. Few studies have been developed to examine levels of satisfaction and commitment of students in the Egyptian higher education context through the digitization era.
Over the examination of a sample of 410 students enrolled in an Egyptian higher education institution, the study proved the validity of all the indicators of the variables in the proposed model. Moreover, all variables had a significant impact on student satisfaction except course structure; there was a positive correlation between student satisfaction and student commitment while course structure had an insignificant impact on student commitment. In addition, the majority of students’ responses were from College of Management and Technology while other colleges were of same responses and this can justify that even disciplines can differ from one another in the satisfaction and commitment level. Therefore, based on the previous literature review, all variables had significant influence on both student satisfaction and commitment except the variable named course structure.
Though the present study provides significant information for students and decision makers concerned with levels of student satisfaction and commitment in online learning environment, it was limited to be applied on a specific higher education institution inside Egypt. To address this limitation, the study should be expanded to other higher education institutions inside Egypt in different settings. It will be beneficial and useful for academics and decision makers to understand the behavior of students concerned with the commitment towards their institutions.
Future exploration and investigation might embrace testing the model on other higher education institutions to determine its validity in forecasting the satisfaction and commitment of students in an online learning environment. Some studies (Sun and Zhang, 2006) suggest that moderator variables such as age and gender add a great value in which they can be tested in the future (HASSAN et al.). Furthermore, more variables can be tested to explore their effect on both satisfaction and commitment.
(adsbygoogle = window.adsbygoogle || []).push({});
References
- ABDI, H. 2010. Partial least squares regression and projection on latent structure regression (PLS Regression). Wiley interdisciplinary reviews: computational statistics, 2, 97-106.
- ALSHEHRI, A. F. 2017. Student satisfaction and commitment towards a blended learning finance course: A new evidence from using the investment model. Research in International Business and Finance, 41, 423-433.
- AMOROSO, D. L. & CHENEY, P. H. 1991. Testing a Causal Model of End-User Application Effectiveness. Journal of Management Information Systems, 8, 63-89.
- ARBAUGH, J. B. 2000. Virtual Classroom Characteristics and Student Satisfaction with Internet-Based MBA Courses. Journal of Management Education, 24, 32-54.
- ARBAUGH, J. B. 2002. Managing the on-line classroom. The Journal of High Technology Management Research, 13, 203-223.
- AŞKAR, P. & HALICI, U. 2004. E-Learning as a Catalyst for Educational Innovation.
- BASHIR, B. & GANAI, A. 2019. Testing the effects of job satisfaction on organizational commitment. Journal of Management Development, ahead-of-print.
- BERGER, N. S. 1999. Pioneering Experiences in Distance Learning: Lessons Learned. Journal of Management Education, 23, 684-690.
- CASEY, D. 2008. A Journey to Legitimacy: The Historical Development of Distance Education through Technology. TechTrends: Linking Research and Practice to Improve Learning,
- CHIN, W. W., THATCHER, J. B., WRIGHT, R. T. & STEEL, D. 1998. Controlling for common method variance in PLS analysis: the measured latent marker variable approach. New perspectives in partial least squares and related methods.
- DAVISON, R. M. 2020. The Transformative Potential of Disruptions: A Viewpoint. International Journal of Information Management.
- DWIVEDI, Y. K., HUGHES, D. L., COOMBS, C., CONSTANTIOU, I., DUAN, Y., EDWARDS, J. S., GUPTA, B., LAL, B., MISRA, S., PRASHANT, P., RAMAN, R., RANA, N. P., SHARMA, S. K. & UPADHYAY, N. 2020. Impact of COVID-19 pandemic on information management research and practice: Transforming education, work and life. International Journal of Information Management.
- EL-EBIARY, Y., AL-SAMMARRAIE, N., MOAIAD, Y. & ALZUBI, M. 2016. The impact of Management Information System in educational organizations processes.
- ELLIOTT, K. & HEALY, M. 2001. CONTENTS Key Factors Influencing Student Satisfaction Related to Recruitment and Retention 1. Journal of Marketing for Higher Education, 10, 1–11.
- EOM, S., ASHILL, N. & WEN, H. J. 2006. The Determinants of Students’ Perceived Learning Outcomes and Satisfaction in University Online Education: An Empirical Investigation*. Decision Sciences Journal of Innovative Education, 4, 215-235.
- FIRDAUS 2006. Measuring Service Quality in Higher Education: Three instruments compared. International Journal of Research & Method in Education, 29, 71-89.
- GARSON, G. D. 2016. Partial least squares: Regression and structural equation models. Asheboro, NC: Statistical Associates Publishers.
- HAIR, J. F., BLACK, W. C., BABIN, B. J., ANDERSON, R. E. & TATHAM, R. L. 2014. Multivariate data analysis, Prentice hall Upper Saddle River, NJ.
- HAIR, J. F., RINGLE, C. M. & SARSTEDT, M. 2011. PLS-SEM: Indeed a silver bullet. Journal of Marketing theory and Practice, 19, 139-152.
- HAIR JR, J. F., HULT, G. T. M., RINGLE, C. & SARSTEDT, M. 2017. A primer on partial least squares structural equation modeling (PLS-SEM), Sage publications.
- HARSASI, M. & SUTAWIJAYA, A. 2018. Determinants of Student Satisfaction in Online Tutorial: A Study of A Distance Education Institution. Turkish Online Journal of Distance Education, 19, 89-99.
- HASSAN, A., ABD EL-AZIZ, R. & HAMZA, M. The Exclusion of People with Visual Disabilities from Digital Banking Services in the Digitalization Era.
- HERMANS, C., HAYTKO, D. & MOTT-STENERSON, B. 2009. Student Satisfaction in Web-enhanced Learning Environments. Journal of Instructional Pedagogies,
- HUMAN-VOGEL, S. & VOGEL, F. 2015. Academic commitment and self-efficacy as predictors of academic achievement in additional materials science.
- KHAN, M. & RAAD, B. 2020. THE ROLE OF E-LEARNING IN COVID-19 CRISIS. 3135.
- LI, N., MARSH, V., RIENTIES, B. & WHITELOCK, D. 2016. Online learning experiences of new versus continuing learners: a large-scale replication study. Assessment & Evaluation in Higher Education, 42, 1-16.
- MAYER, R. E. 2003. Elements of a Science of E-Learning. Journal of Educational Computing Research, 29, 297-313.
- MCAULEY, A. J., STEWART, B., SIEMENS, G. & CORMIER, D. The MOOC model for digital practice. 2010.
- MOORE, M. G. 1991. Editorial: Distance education theory. American Journal of Distance Education, 5, 1-6.
- NACHTIGALL, C., KROEHNE, U., FUNKE, F. & STEYER, R. 2003. Pros and cons of structural equation modeling. Methods Psychological Research Online, 8, 1-22.
- PALLANT, J. & MANUAL, S. S. 2010. A step by step guide to data analysis using SPSS. Berkshire UK: McGraw-Hill Education.
- PICCOLI, G., AHMAD, R. & IVES, B. 2001. Web-Based Virtual Learning Environments: A Research Framework and a Preliminary Assessment of Effectiveness in Basic IT Skills Training. MIS Quarterly, 25, 401-426.
- SAMI, P., SABRI, U., ILYAS, M. & AMJAD, Z. 2012. Organizational Culture and Its Impact on the Job Satisfaction of the University Teachers of Lahore.
- SMART, K. & CAPPEL, J. 2006. Students’ Perceptions of Online Learning: A Comparative Study. JITE, 5, 201-219.
- SUN, H. & ZHANG, P. 2006. The role of moderating factors in user technology acceptance. International journal of human-computer studies, 64, 53-78.
- SUN, P.-C., TSAI, R. J., FINGER, G., CHEN, Y.-Y. & YEH, D. 2008. What drives a successful e-Learning? An empirical investigation of the critical factors influencing learner satisfaction. Computers & Education, 50, 1183-1202.
- TENENHAUS, M., VINZI, V. E., CHATELIN, Y.-M. & LAURO, C. 2005. PLS path modeling. Computational statistics & data analysis, 48, 159-205.
- TSUI, K. T. & CHENG, Y. C. 1999. School Organizational Health and Teacher Commitment: A Contingency Study with Multi-level Analysis. Educational Research and Evaluation, 5, 249-268.
- WEBSTER, J., & HACKLEY, P. 1997. Teaching effectiveness in technology-mediated distance learning. Academy of Management Journal, 40, 1282–1309.
- WILSON, M., BAKKABULINDI, F. & SSEMPEBWA, J. 2016. Validity and Reliability of Allen and Meyer’s (1990) Measure of Employee Commitment in the Context of Academic Staff in Universities in Uganda. JOURNAL OF SOCIOLOGY AND EDUCATION IN AFRICA,
- WOMACK, A. J. 2016. Profiles of Academic Commitment. Doctor of Philosophy (PhD), University of Southern Mississippi.
- XIONG, B., SKITMORE, M. & XIA, B. 2015. A critical review of structural equation modeling applications in construction research. Automation in Construction, 49, 59-70.