Introduction
For decades, the theory of Big Data Analytics has aided all sectors in identifying the best strategies and innovations. This description has evolved over time, as do the methods used in large data processing. The analytics offer a practical means for the user to stay agile. Big data refers to methods for manipulating, continuously collecting information from, or otherwise dealing with data sets that are too large or complex for traditional data analysis program applications to handle. Data collection, data management, data processing, searching, posting, downloading, visualizing, querying, updating, anonymity, and source data are all big data topics (Cavanillas et al., 2016). The higher education institutions (HEIs) keep a lot of information on students and teachers. This information is vital as it helps educational organizations to improve their organizational effectiveness. Variables such as the actions of students, the results of tests and the progress of each student, as well as their educational needs, would be focused on changing educational needs.
Most of the institutions of higher education collect more data than ever before. Nevertheless, this research found evidence that most of these data were used to meet credentialing or reporting requirements rather than to address strategic questions, and many of the data collected are not used at all. Analytics in HEIs are mainly used in the fields of enrolment management, student progress, and institutional finance and budgeting.
Big data application at HEIs in Malaysia is still in its early stage. The public universities have a large amount of data that could be mined using big data applications. According to Tarmidi et al. (2018), a survey of readiness for big data among accounting and finance students in public universities revealed that the awareness and readiness for big data is still at infant stage. In addition, Hanapiyah et al. (2018) stated that there are difficulties in accessing the required data from the database due to low quality and inaccurate data organization. However, at the Malaysian Ministry of Higher Education (MOHE) level, the first phase of Big Data centres has been launched across four Malaysian public universities and one polytechnic, consulted by the Prestariang Group (Hanapiyah et al., 2018). Hence, it could be presumed that BDM research projects and its development initiative in Malaysian universities are at its introductory stage. Hence, this research focuses on studying the key precedents for public universities in adopting BDM for their strategic advantages, via qualitative approach. As such, BDM applications and implementation could be enhanced and empowered to complement the Industry 4WRD policy paper that had been launched by the Malaysian Government in 2018. The Industrial Revolution 4.0 (IR 4.0) sparked a new wave of educational reform. In recent years, educational scholars have recognized the enormous impact of a variety of ICT technological advancements on education. They both believe that innovation will affect Education 4.0, and that students will need to be trained to create new ideas and potential solutions.
Research Problem
Data measurement is increasing rapidly across different sectors worldwide. With this development, there is a direct need to develop a system that enables assimilation and data review for better decision-making. It could be noted that there are various procedures for developing data covering issues such as consolidation, isolation, naming, searching and access to unstructured and structured data (Patel et al., 2012). The main challenge is to manage and analyze the large measure of data in order to recognize key trends in decision making.
The significant point is that previous work through big data concentrated mainly on technological features such as machine learning or software algorithms and system development (Haddad et al., 2018). However, not much research is found on how factors affect big data adoption or the challenges encountered during implementation for higher education institutions (Santos et al., 2018). Nowadays, many academic institutions are heading to cloud architectures and with users ‘ increased use of digital devices in these ecosystems, many data are collected in these institutions than ever before, creating significant opportunities to use big data to analyze and compare information that improves decision making.
In order to enhance the quality of learning outcomes, it is important to evaluate effectively large volumes of data produced by educational systems to promote suitable responses to new challenges (Murumba & Micheni, 2017b). Big data poses many technical problems that include constant upgrading of resources and expertise, meaning higher education institutions need to have sufficient budgets to overcome these concerns. Legitimate concerns about privacy do exist, particularly regarding data from online sources. This combined with the digital divide in many nations poses barriers to using Big Data’s power for the benefit of educational system users.
Research Question
- What are the supportive factors that may influence the adoption of new integrated big data competence model in Malaysia Higher Institutions?
- What are the challenging constraints that may affect the adoption of new integrated big data competence model in Malaysia Higher Education Institutions?
Research objective
- To explore the supportive factors that are able to influence the adoption of new integrated big data competence model.
- To investigate the challenging constraints that may affect the adoption of new integrated big data competence model.
Research scope
This research focuses on the institution’s top management group because it is focused on a targeted strategic management group of respondents which can be demographically linked to academicians. ATLAS.TI is the instrument used to analyze the data after they have been collected from the interviewees. The research’s final results address the most important determinants of big data adoption in Malaysian Higher Education Institutions. It is important to review existing BDM literature which provides a golden opportunity to serve as the basis for this new research.
Significance of the Study
Many facets of education have changed as a result of big data movement. Opportunity to track educational programs is one of the most important improvements that big data has brought to education. Educational institution will soon undergo a major transition that will be somewhat different from what it is now. Data scientists use three fundamental features to interpret it: volume, velocity, and variety (3Vs). Volume refers to the sum of data, velocity to the speed at which data travel and are stored, and variety to the data types and attributes. The improvement in learning success will be focused on the education system, which will revolutionize the way it develops students from higher education, who will select a career and be guided to acquire skills in any course. Educational tools can be developed based on data and analytics to address the demands of both students and teachers. In the classroom, for example, the advancement of facial recognition and voice-based learning would change the learning method and speed.
Literature Review
Data
Data are accumulating at an alarming rate, and they can be found almost anywhere. Data are described as numerical information or features that are collected through observation. Furthermore, data are a set of values for qualitative or quantitative variables gathered from data that include one or more people or objects, while a datum is a single value for a single variable. Data can be measured, processed, recorded, and analyzed, and they can be presented in a variety of ways, including photographs, graphs, and other methodologies.
Big Data
Three characteristics can assist in breaking the term down. Volume, velocity, and variety are the “3Vs,” and they’re key to learning how to analyse large data and how big data differs from traditional data. Big Data is characterised by the “3Vs” (volume, velocity, and variety), but there are now “5Vs” (volume, veracity, velocity, value, and variety) that are often referred to as Big Data characteristics.
Big Data Management
Big data is relevant both economically and scientifically. BDM focuses on big data modelling skills and strategies for gathering, organizing, storing, and enhancing related data. When developing a big data management approach, it’s critical to consider problems like big data convergence, deception, consistency, and management (Kaur & Sood, 2017). Big data management efficiency is a time-consuming activity that often experiences occasional delays or poor results. One of the most important facets of Big Data Management is to keep up with emerging technologies (Almeida, 2017).
Big Data Management in the Education Sector
Big data enables organizations to more effectively leverage internal data to explore new domains for growth opportunities and to improve the knowledge sector (Martin & Thawabieh, 2017). New innovations in managing data, information and knowledge lead to higher organizational performance, thorough new service being offered to customers (Tasmin & Woods, 2007). According to Ruddle (2009), a specific need for today’s global community’s educational content is widely accessible worldwide. Academic institutions have long existed in an information-rich environment, producing and gathering vast volumes of data on a regular basis. For organisations, data are an important resource for guiding daily operations as well as long-term institutional and organisational decisions.
Technology Organization Environment (TOE)
The Technology Organization Environment (TOE) is a theoretical concept introduced by Thornatzky and Fleischer in 1990 that defined the behavioral traits of technology. Environmental conditions are a critical predictor of technology acceptance for institutions’ organizational readiness (Chong & Olesen, 2017). The adoption of technological innovation can be identified by a 3-stage technique for initiation, adoption and implementation. The initiation phase involves the classification and evaluation of technological innovation that meets the needs of the organization. The phase of adoption needs to make a decision determination to adopt the innovation based on the distinctive conditions of adoption (Lippert & Ph, 2006). The implementation process aids in the introduction of innovation into development activities. The TOE paradigm supports an important analytical framework for higher education institutions that takes into account both internal and external considerations in order to examine the use of technological development (Wahab & Olugu, 2019). In addition, this research concentrates on the development of the following conceptual framework, which incorporates the TOE framework. In the analytical context, the variables from the TOE framework were used as independent variables, and the real use of BDM was used as a dependent variable.
Factors adopted Big Data in HEIs Malaysia and Conceptual Framework
Technology Awareness, Technology Readiness, Reliability Technology, Top Management Support, and Technology Infrastructure are all independent variables in this research framework context. This context has affected the dependent variable, which is the intention to implement BDM from the TOE framework, as shown in Figure 1
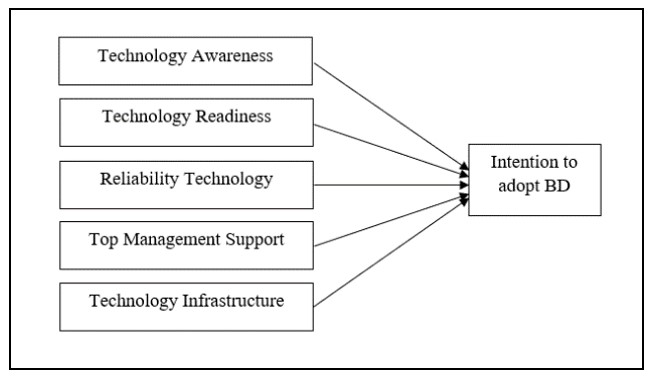
Fig. 1: Big Data Qualitative Research Framework
Intention to adopt Big Data
In this study, the intention to use refers to the extent to which higher education institutions are committed to incorporating BDM into their systems. Technology Awareness, Technology Readiness, Technology Reliability, Top Management Support, and Technology Infrastructure are five factors that influence the decision to implement BDM. According to previous research, the use of BDM technologies would aid the system in improving performance and achieving the desired result while reducing the effort.
Technology Awareness
It is essential to provide a basic understanding of technology and awareness in a higher education institution. The aim is to maximise its capacity in the sense of an organisation (Verma, 2017). Technology knowledge is a consideration that is based on big data deployment processes that are ideal for effective decision-making. Big data is important in assessing the level of big data knowledge and expertise will help the organisation develop successful big data management strategies (Izhar & Shoid, 2016)
Technology Readiness
The technological readiness principle is used to assess a company’s ability to respond to new technology. Technology readiness, or technology competence, is a term used to describe the ability to use technology (Huda et al., 2017). Recognizing and implementing different aspects of big data technical applications as a core component of the organization’s milestones has been critical in gaining a better understanding (Izhar & Shoid, 2016). The organisation must decide how easy the big data approach is too incorporated and how well it integrates with existing processes, as well as the possible advantages of this emerging technology (Sam & Chatwin, 2019).
Reliability Technology
Big Data reliability review is critical. Through evaluation and analysis, technology reliability improves product reliability. It is also capable of preventing the production of a defective component. Big Data collects a large amount of data and analyses it in order to achieve outstanding results quickly. Engineers of Reliability Technology would only be able to do detailed reliability studies if the data are searchable and clearly explained throughout the system (Williamson, 2018).
Top Management Support
For educational institute, top management support for technology implementation is especially important. To increase executive support and consent for the use of Big Data in higher education institutions, top management participation is needed (Sun et al., 2018). Aside from that, senior management interest in the implementation and use of big data is critical for both promoting and sponsoring a new technology and assisting in the removal of future roadblocks. According to the findings of a previous survey, top management guidance has a favourable impact on big data adoption (Chong & Olesen, 2017). Top management interest in the introduction and use of Big Data is critical for embracing a new technology as well as overcoming transition barriers.
Technology Infrastructure
The institution’s tangible capabilities are defined by its technology infrastructure. For example, physical assets are required for organizations to implement technological innovations. Technology Infrastructure is in charge of advancing advanced new colleges while also reforming higher education and establishing a secret marketing framework in higher education. Increasing access to higher education Technology infrastructure needs private-sector consulting firms, app engineers, cloud storage companies, data analytics programmers, and a slew of other technological experts (Williamson, 2018). Higher Education’s technology infrastructure can handle the complexity of big data.
Research Methodology
The data for this research were collected from primary sources that were collected by the researchers themselves, from first-hand sources which are based on Google Meet online interviews. In the first stage, the respondents are identified based on their expertise in BDM research and their respective literature articles. The first respondent was identified (interviewee A) and then snow-balling technique was applied. Interviewee A was politely asked to recommend a BDM expert from other Malaysian public and private universities, and so on. At initial stage, the interviewee was asked to rank from 1 to 5 on five factors of BDM adoption by his/her university, via Google Form survey. Subsequently, a schedule was arranged for a Google Meet session to be held, which was executed in an open-ended interview session. There were 4 interviewee respondents being engaged in this qualitative study, which involved 4 lecturers from 2 private and 2 public universities in Malaysia.
The methodological tool for analyzing the data used is ATLAS.TI version 9. This software is one of the latest methods for computer-assisted qualitative data analysis. Subsequently, developing a coding scheme is the next step. These initial themes could be installed to begin creating a coding scheme. There is a list of all the themes, ‘scripts’, to be implemented into the data. The next step is to transcribe-out the interview texts or coding the data. This next step is to start implementing these codes to the entire data set either by writing codes on the transcript margins or notes or labeling the quotation by using ATLAS software.
Results
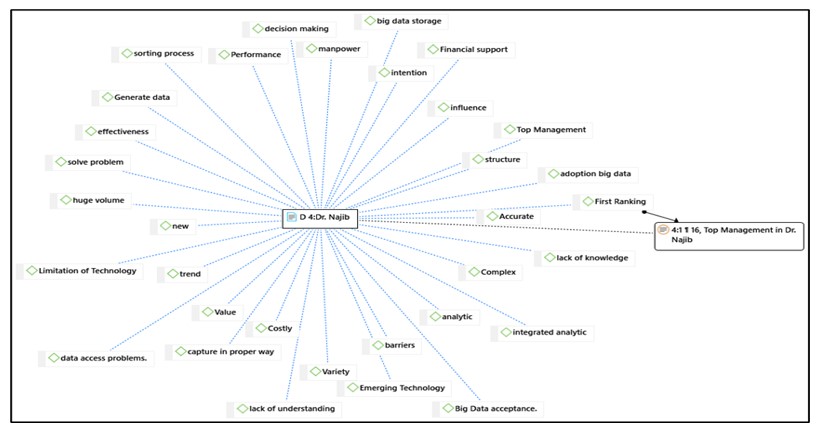
Fig 2. Interviewee A based on Word Frequency Coding
Figure 2 shows Interviewee A Coding. Interviewee A is a professor from the faculty of computer science and information technology, Universiti Tun Hussein Onn Malaysia. ATLAS.TI version 9 has coded out the major keyword themes from the interview transcript. The farther the distance of a word from the central position signifies that such word has been more frequently used, in the interview session. Figure 1 has shown that the five major key themes’ coding are namely, 1) limitation of technology, 2) data access problems, 3) lack of understanding, 4) big data acceptance and lastly 5) big data storage. This BDM expert has also firstly ranked the factor of “Top Management Support” as the most important factor in the adoption of BDM at his university.
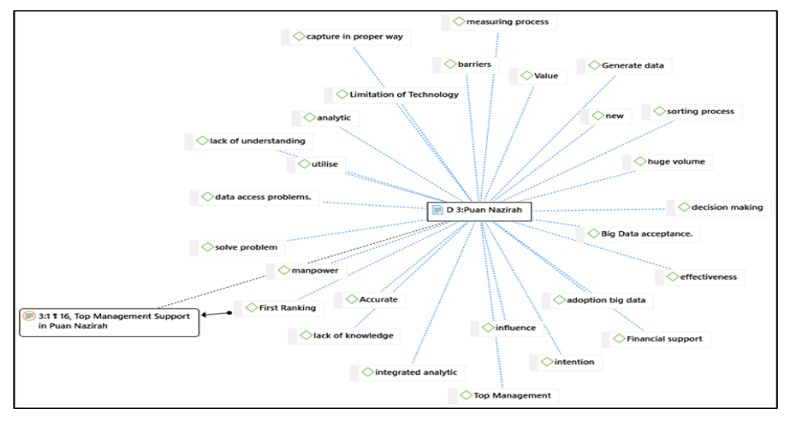
Fig 3. Interviewee B based on Word Frequency Coding
Figure 3 shows Interviewee B Coding. Interviewee B is a senior lecturer from the faculty of creative multimedia, Multimedia University (MMU), Cyberjaya. ATLAS.TI version 9 has coded out the keyword themes from the interview transcript. Figure 2 has shown the major five keyword themes’ coding namely, 1) lack of understanding, 2) data access problems, 3) top management, 4) financial support and lastly 5) measuring process. This big data expert has also firstly ranked the factor of “Top Management Support” as the most important precedence in the adoption of BDM approach at her university.
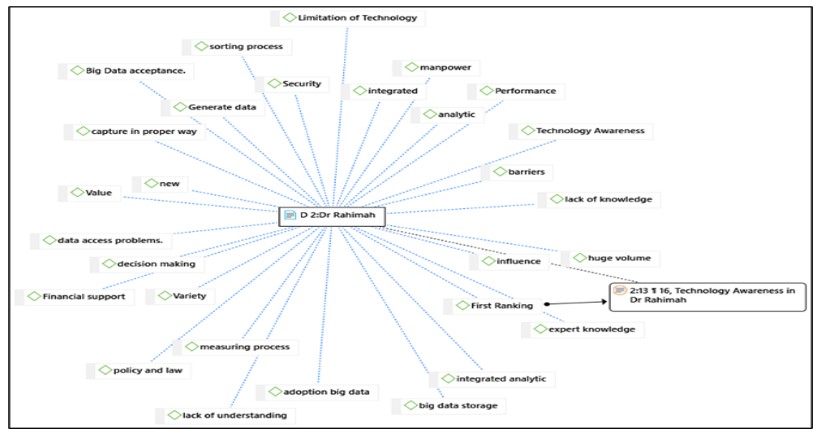
Fig 4. Interviewee C based on Word Frequency Coding
Figure 4 shows Interviewee C Coding. Interviewee C is a senior lecturer from the Malaysian Institute of Industrial Technology, the Kuala Lumpur University (UniKL). Software ATLAS.TI version 9 has coded out the keyword themes’ coding, from the interview transcript. Figure 3 has shown the major five keyword themes’ coding namely, 1) Financial support, 2) Lack of understanding, 3) Data access problems, 4) Expert knowledge and lastly 5) Limitation of technology. This UniKL BDM expert has firstly ranked the factor of “Technology Awareness” as the most important precedence in the adoption of BDM approach at her university.
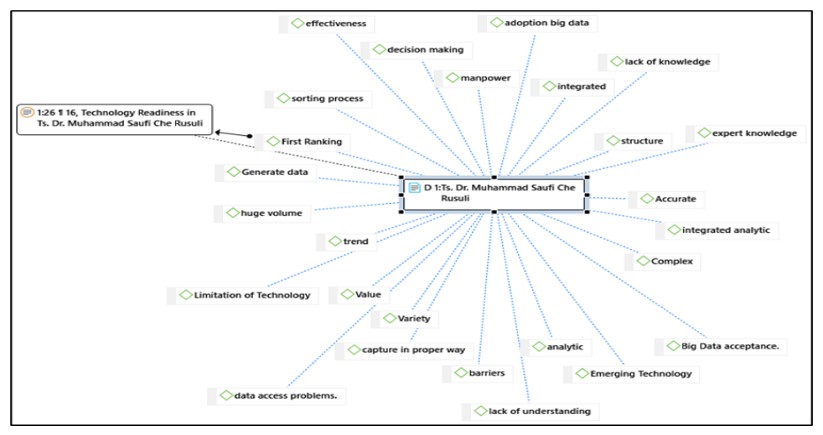
Fig 5. Interviewee D based on Word Frequency Coding
Figure 5 shows Interviewee D Coding. The interviewee is the senior lecturer from the faculty of business and entrepreneurship management, UMK Kampus Kota (Universiti Malaysia Kelantan). ATLAS.TI version 9 has coded out the keywords from the interview transcript. Figure 4 has shown the major five keyword themes’ coding namely, 1) Data access problems, 2) Limitation of technology, 3) Lack of understanding, 4) Expert knowledge and lastly 5) Effectiveness. This BDM expert has firstly ranked the factor of “Technology Readiness” as the most important precedence in the adoption of BDM approach at his university.
Summative Findings
The overall perspective of the qualitative findings is consolidated in Table 1, as shown in the 5-factors ranking column and with their respective 5 major BDM-enabling keyword themes.
Table 1: Summative findings of TOE factors’ ranking and 5 major BDM keyword themes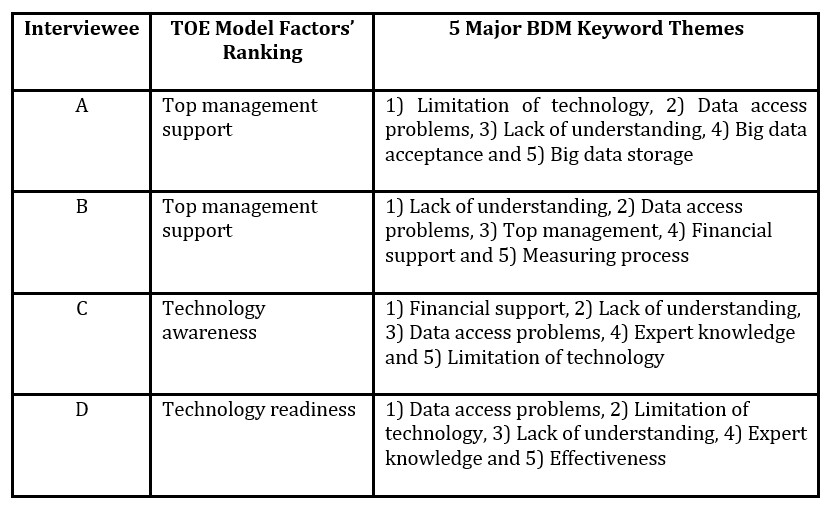
Discussions
Generally, it is widely accepted that BDM approach in Malaysian HEIs is at the introductory stage. Hence, it is essential to develop good general understanding and its approach for successful implementation. Based on the TOE model, this research has managed to evince that the most influential factor is top management support. Two out of four academic interviewees ranked the factor of top management support as the first. In Malaysian HEIs management setting, it is customary and highly expected that any new initiative or change management project must be with permission granted from the top management group, or in other words top-down approach. In addition, this research has also managed to coin-out the challenging problems that may arise out of any intention to adopt big data into Malaysian HEIs, namely and primarily are data access problems, lack of understanding and limitation of technology.
The supportive factors that may influence the adoption of new integrated big data competence model in Malaysia Higher Institutions
The evinced supportive factors that may influence the adoption of BDM in Malaysian HEIs are top management support, technology awareness and technology readiness. It is regarded as widely acceptable that in many organizations, new project of change must be blessed by the top management cluster, for mostly of its authority to allocate resources, including financial means. According to Hanapiyah et al. (2018), when the University of Arizona, USA, decided to engage big data analytics to create higher values out of its existing data for its strategic management and future university direction, the top management allocated resources and created big data center for this purpose. This signifies the importance of top management support as a motivating factor that aids toward making new initiative that well will help get the organization moving and working. Technology awareness and readiness must always be the precedent of any new technology introduction to organization and even society. For example, introduction of digital wallet in Malaysia such as ‘Boost’ and ‘Touch N Go’ has been made aware and preceded with digital money incentive scheme by the government and widespread promotion in media, advertisement and appointed influencers.
The challenging constraints that may affect the adoption of new integrated big data competence model in Malaysia Higher Education Institutions
One of the major challenges, and also becoming constraint in adopting BDM at the HEIs in Malaysia, is data access problems posed by the data owners, such as the students’ academic management center, strategic planning office, Alumni center and information technology center. Each specific strategic business unit, such as the academic management center, has to maintain its status quo for the students’ information data, in the name of confidentiality and student data secrecy. In most situations, it is reluctant to open their database to external party usage (big data analysts). Another challenging constraint is lack of understanding for what the purpose of the data to be analyzed by the big data analysts. For one thing is the rendered trust placed upon the data users, hence assuming that the derived data would be used inappropriately and unethically. The last major challenges for HEIs in adopting BDM is the notion of limitation of technology. It is widely believed that even engaging the latest big data management technology, such as Hadoop and R-programming, it would not solve all the needs, wants and wishes for using the derived data. There are still limitations of what BDM technologies could offer due to data quality, accuracy and legacy data which exist in many varieties of formats and platforms.
Conclusion
The aim of this research is to present a comprehensive overview of the precedents of big data adoption for higher education institutions in Malaysia. This research contains two research questions which are formulated to focus on the adoption of Big Data in HEIs. The intention to adopt BDM is formed by five factors which are technology awareness, technology readiness, technology reliability, top management support and technology infrastructure. Top management support is highlighted as the major factor to influence the intention to adopt BDM in Malaysian HEIs. Alas, it has been noted that the implementation of BDM approach at Malaysian universities is at its infancy stage. Thus, it is strongly suggested that the Ministry of Higher Education, Malaysia, initiates a game-changing initiative to introduce the BDM approach to few selected key universities, such as the research-based universities, to embrace the big data analytic approach in their strategic management and future university direction. University top management support will move forward if it is the direction and instruction from the ministry itself. Only then the university top management could be empowered to launch their respective programme and scheme to initiate respective BDM project/initiative within their university management scope.
The keyword theme coding analysis derived from the done four interview sessions is showing that the major constraint is data access problems. This syndrome is due to maintaining status quo of departmental data owners. Departmental heads insist to keep and maintain their data ownership and sense of pride in keeping their data. As suggested earlier, these managers won’t budge their mentality to share the needed data; if it is not the direction from the university top management to open-up their departmental data. As it has been suggested above that if the Ministry of Higher Education has issued the direction for embracing BDM approach (top-down instruction), for creating higher values to better chart university direction; only then, these university managers would open-up their departmental data. By this it means that top management support from the ministry and the university levels would address the challenge of data access problems at the data manager/owner level. Hence, when this data access issue has been addressed well, the BDM approach would be much well accepted and it could progress forward, beyond its current introductory stage.
Acknowledgement
This research was supported by the Ministry of Higher Education of Malaysia (MOHE), through Fundamental Research Grant Scheme (FRGS/1/2019/SS03/UTHM/02/1) and partially sponsored by Universiti Tun Hussein Onn Malaysia (UTHM).
(adsbygoogle = window.adsbygoogle || []).push({});
References
- Al-Barashdi, H., & Al-Karousi, R. (2019). Big Data in academic libraries: literature review and future research directions. Journal of Information Studies & Technology (JIS&T), 2018(2).
- Almeida, F. L. F. (2017). Benefits, Challenges and Tools of Big Data Management. Journal of Systems Integration, 8(4), 12–20.
- Angelini, M., Catarci, T., Mecella, M., & Santucci, G. (2018). A Comprehensive Guide Through the Italian Database Research Over the Last 25 Years. Springer, 31(April), 3–25.
- Bamiah, M. A., Brohi, S. N., & Rad, B. B. (2018). Big data technology in education: Advantages, implementations, and challenges. Journal of Engineering Science and Technology, 13(Special Issue on ICCSIT 2018), 229–241.
- Banik, A., & Bandyopadhyay, S. K. (2018). Big Data-A Review on Analysing 3Vs Available online www.jsaer.com Journal of Scientific and Engineering Research , 2016 , 3 ( 1 ): Big Data- A Review on Analysing 3Vs. January 2016.
- Bello-Orgaz, G., Jung, J. J., & Camacho, D. (2016). Social big data: Recent achievements and new challenges. Information Fusion, 28, 45–59.
- Brancheau, J. C., & Wetherbe, J. C. (1990). The adoption of spreadsheet software: Testing innovation diffusion theory in the context of end-user computing. Information Systems Research, 1(2), 115–143.
- Cavanillas, J. M., Curry, E., & Wahlster, W. (2016). New Horizons for a Data-Driven Economy: A Roadmap for Usage and Exploitation of Big Data in Europe. In New Horizons for a Data-Driven Economy: A Roadmap for Usage and Exploitation of Big Data in Europe (Issue January 2015).
- Chong, D., & Shi, H. (2015). Big data analytics: a literature review. Journal of Management Analytics, 2(3), 175–201.
- Chong, J. L. L., & Olesen, K. (2017). A technology-organization-environment perspective on eco-effectiveness: A meta-analysis. Australasian Journal of Information Systems, 21, 1–26.
- Chopra, A. (2017). Analytics in Higher Education System. International Journal for Research in Applied Science and Engineering Technology, V(XI), 1730–1734.
- Chris Dede, B., Ho, A., & Mitros, P. (2016). Big Data Analysis in Higher Education: Promises and Pitfalls.
- Daniel, B. (2015). Big Data and analytics in higher education: Opportunities and challenges. British Journal of Educational Technology, 46(5), 904–920.
- Daniel, B. K. (2016). Big data and learning analytics in higher education: Current theory and practice. Big Data and Learning Analytics in Higher Education: Current Theory and Practice, 1–272.
- Daniel, B. K. (2019). Big Data and data science: A critical review of issues for educational research. British Journal of Educational Technology, 50(1), 101–113.
- Experteninterviews, L.-. (2019). Handbuch Methoden der empirischen Sozialforschung. In Handbuch Methoden der empirischen Sozialforschung. Springer Fachmedien Wiesbaden.
- Gabriel, Y. (2007). Qualitative Research in Organizations and Management : An Article information : An International Journal, 10(4), 332–336.
- Gong, H., & Li, X. (2015). SCIENTIA SINICA Informationis, 45(1), 1–44.
- Haddad, A., Ameen, A., & Mukred, M. (2018). The Impact of Intention of Use on the Success of Big Data Adoption Via Organization Readiness Factor. International Journal of Management and Human Science (IJMHS), 2(1), 43–51.
- Hanapiyah, Z. M., Wan Hanafi, W. N., & Daud, S. (2018). Issues, Challenges and Opportunities of Big Data Management in Higher Education Institutions in Malaysia. Indian Journal of Science and Technology, 11(4), 1–6.
- Hsiao, K., & Bomhold, C. R. (2013). Library Hi Tech News Article information : Library Hi Tech, 32(3), 409–422.
- Hsinchun, C., Roger, H. L. C., & Veda, C. S. (2018). Business Intelligence and Analytics: From Big Data To Big Impact. MIS Quarterly, 36(4), 1293–1327.
- Huda, M., Maseleno, A., Shahrill, M., Jasmi, K. A., Mustari, I., & Basiron, B. (2017). Exploring adaptive teaching competencies in big data era. International Journal of Emerging Technologies in Learning, 12(3), 68–83.
- Hurley, M. A., & Harris, R. (1997). Facilitating corporate knowledge: building the data warehouse. Information Management & Computer Security, 5(5), 170–174.
- Hwang, Y. (2019). Adoption of Big Data in Higher Education for Better Institutional Effectiveness. American Journal of Creative Education, 2(1), 31–44.
- Izhar, T. A. T., & Shoid, M. S. M. (2016). A Research Framework on Big Data awareness and Success Factors toward the Implication of Knowledge Management: Critical Review and Theoretical Extension. International Journal of Academic Research in Business and Social Sciences, 6(4).
- Kaur, N., & Sood, S. K. (2017). Efficient Resource Management System Based on 4Vs of Big Data Streams. Big Data Research, 9(February), 98–106.
- Kitchin, R., & McArdle, G. (2016). What makes Big Data, Big Data? Exploring the ontological characteristics of 26 datasets. Big Data and Society, 3(1), 1–10.
- Krén, E., Szabó, P., Pál, L., Tarnóczi, T., Kádár, G., & Hargitai, C. (1968). X-ray and susceptibility study of the first-order magnetic transformation in Mn3Pt. Journal of Applied Physics, 39(2), 469–470.
- Limited, I. (2018). Effective Data Governance. Perspective – Infosys Journal.
- Lippert, S. K., & Ph, D. (2006). Technological , Organizational , and Environmental Antecedents to Web Services Adoption. 6(1).
- Lorenzo, S. (2013). The Use of Big Data in Electrical. September 2014. http://repositorio.unican.es/xmlui/bitstream/handle/10902/4525/TFM – Sheila Lorenzo FernándezS.pdf?sequence=1
- Majid, U. (2018). Research Fundamentals: Study Design, Population, and Sample Size. Undergraduate Research in Natural and Clinical Science and Technology (URNCST) Journal, 2(1), 1–7.
- Martin, A. L., & Thawabieh, F. A. (2017). The Role of Big Data Management and Analytics in Higher Education. Business, Management and Economics Research, 3(7), 85–91.
- Mikalef, P., Giannakos, M. N., Pappas, I. O., & Krogstie, J. (2018). The human side of big data: Understanding the skills of the data scientist in education and industry. IEEE Global Engineering Education Conference, EDUCON, 2018–April(January), 503–512.
- Moghadam, R. S., & Colomo-Palacios, R. (2018). Information security governance in big data environments: A systematic mapping. Procedia Computer Science, 138, 401–408.
- Murumba, J., & Micheni, E. (2017a). Big Data Analytics in Higher Education: A Review. The International Journal of Engineering and Science, 06(06), 14–21.
- Murumba, J., & Micheni, E. (2017b). Big Data Analytics in Higher Education: A Review. The International Journal of Engineering and Science, 06(06), 14–21.
- Patel, A. B., Birla, M., & Nair, U. (2012). Addressing big data problem using Hadoop and Map Reduce. 3rd Nirma University International Conference on Engineering, NUiCONE 2012, 6–8.
- Patgiri, R., & Ahmed, A. (2017). Big Data: The V’s of the Game Changer Paradigm. Proceedings – 18th IEEE International Conference on High Performance Computing and Communications, 14th IEEE International Conference on Smart City and 2nd IEEE International Conference on Data Science and Systems, HPCC/SmartCity/DSS 2016, December, 17–24.
- Rodríguez-Mazahua, L., Rodríguez-Enríquez, C. A., Sánchez-Cervantes, J. L., Cervantes, J., García-Alcaraz, J. L., & Alor-Hernández, G. (2016). A general perspective of Big Data: applications, tools, challenges and trends. Journal of Supercomputing, 72(8), 3073–3113. https://doi.org/10.1007/s11227-015-1501-1
- Ruiz-Palmero, J., Colomo-Magaña, E., Ríos-Ariza, J. M., & Gómez-García, M. (2020). Big data in education: Perception of training advisors on its use in the educational system. Social Sciences, 9(4). https://doi.org/10.3390/SOCSCI9040053
- Sam, K. M., & Chatwin, C. R. (2019). Understanding Adoption of Big Data Analytics in China: From Organizational Users Perspective. IEEE International Conference on Industrial Engineering and Engineering Management, 2019–Decem, 507–510. https://doi.org/10.1109/IEEM.2018.8607652
- Sanders, J. (2016). Defining terms: Data, information and knowledge. Proceedings of 2016 SAI Computing Conference, SAI 2016, 223–228. https://doi.org/10.1109/SAI.2016.7555986
- Santos, A. F. C., Teles, Í. P., Siqueira, O. M. P., & de Oliveira, A. A. (2018). Big data: A systematic review. Advances in Intelligent Systems and Computing, 558, 501–506. https://doi.org/10.1007/978-3-319-54978-1_64
- Shacklock, X. (2016). From Bricks to clicks. The Potential of Data and Analytics in Higher Education. http://www.policyconnect.org.uk/hec/sites/site_hec/files/report/419/fieldreportdownload/frombrickstoclicks-hecreportforweb.pdf
- Shahroom, A. A., & Hussin, N. (2018). Industrial Revolution 4.0 and Education. International Journal of Academic Research in Business and Social Sciences, 8(9), 314–319.
- Shekhar, H., & Sharma, M. (n.d.). A Framework for Big Data Analytics as a Scalable Systems.
- Sun, S., Cegielski, C. G., Jia, L., & Hall, D. J. (2018). Understanding the Factors Affecting the Organizational Adoption of Big Data. Journal of Computer Information Systems, 58(3), 193–203.
- Tarmidi, M., Ahmad, N. & Roni, R.A. (2018). Readiness for Big Data among Students in Public Universities. Global Business and Management Research: An International Journal Vol. 10, No. 3 (2018, Special Issue).
- Tasmin, R. & Woods, P. (2007). Relationship between corporate knowledge management and the firm’s innovation capability. International Journal of Services Technology and Management, 8(1), 62-79.
- Umezuruike, C., & Ngugi, H. N. (2020). Imminent Challenges of Adoption of Big Data in Educational Systems in Sub-Saharan Africa Nations. International Journal of Recent Technology and Engineering, 8(5), 4544–4550.
- Verma, S. (2017). The Adoption of Big Data Services By Manufacturing Firms: an Empirical Investigation in India. Journal of Information Systems and Technology Management, 14(1), 39–68.
- Wahab, S. N., & Olugu, E. (2019). Big Data Analytics Adoption in Malaysia Warehousing Industry Khalid S . Soliman International Business Information Management Association ( IBIMA ). December.
- Wang, Y. (2016). Big Opportunities and Big Concerns of Big Data in Education. TechTrends, 60(4), 381–384.
- What is Data | University of Minnesota Libraries · University of Minnesota Libraries. (n.d.). Retrieved May 23, 2020, from https://www.lib.umn.edu/datamanagement/whatdata
- Williamson, B. (2018). The hidden architecture of higher education: building a big data infrastructure for the ‘smarter university.’ International Journal of Educational Technology in Higher Education, 15(1), 1–26.
- Wilson, R. (2016). Deductive and Inductive Reasoning The two major types of reasoning. Www.Mscc.Edu. http://www.mscc.edu/documents/writingcenter/Deductive-and-Inductive-Reasoning.pdf