Introduction
Nowadays, countries around the world are facing an unprecedented situation with a health pandemic turning into an economic and social crisis. At the end of January 2020, the World Health Organization declared a global health emergency. Since this announcement, most national governments started to adopt measures to reduce the spread of the virus, which has also included travel restrictions that have made it impossible to carry out certain activities as well as the supply of raw materials. In this context, sectors such as tourism, airlines, and manufacturing have suffered the biggest decline since the financial crisis from 2008.
The lockdown phenomenon, the consumer behavioural changes, as well as reduced demand and disruption of the supply chain have contributed to the challenges that industrial production is facing, generating losses that will not be easily recovered. Although the national authorities have taken steps to recover the industrial sector, the outlook for both industry and the economy as a whole remains exposed to uncertainty.
The motivation for choosing this theme consists in the concern that the disruption in manufacturing caused by COVID-19 has generated severe social (inceasing unemployment and poverty, deepening inequalities) and budgetary (deficit and debt hikes) issues. There is also a major concern about how to respond to the industrial production challenges to mitigate the overall effects on the economy, mainly through the job losses channel. The main objective of this article is to assess the impact of the COVID crisis on industrial production in the European Union (EU), but also on the confidence in this sector.
Regarding the structure of this article, first we analysed the economic literature related to the theme studied. Second, we described the methods we used to assess the impact of COVID-19 shock on the industrial sector. In the third section, we have provided the main results of the study and the specific interpretations. Finally, we have drawn the study conclusions and we addressed some issues related to the appropriate way forward.
Literature Review
The industrial production sector is one of the most important sectors in the economy. At the same time, the industrial sector may be severely affected by internal and external shocks resulting from fluctuations in the business cycle (Behun et al., 2018). Thus, the decline in economic activity caused by lockdown has generated substantial decreases in global industrial production. Furthermore, at European Union level, some industrial companies experienced a dramatic fall as a result of measures imposed by the authorities to limit the spread of COVID-19.
According to the International Labour Organisation (2020), the manufacturing sector has been identified as one of the worst affected by the measures taken to contain the spread of the virus. The organization also estimated that about 54% of all employers in the world work in vulnerable industrial sectors, which has a major impact on employment.
Among the economists, the pandemic is considered as one of the most severe supply chain disruptions in history (Ivanov and Dolgui, 2020), the direct impact of the measures taken being dramatic and unprecedented (Wuest et al., 2020). Moreover, the Euro Area has been severely affected, the business activity collapsing to a record low in March 2020, while US industrial production registered the biggest monthly decline since the end of the World War II (Badkar and Greeley, 2020).
Regarding the way of spreading the negative effects in the economy, Cai (2020) identifies two stages through which the COVID-19 shock affected industrial production in 2020. The first stage, related to the period February-March, is represented by the forced interruption of production in China, which reduced the supply of raw materials in EU Member States (as a consequence of the delay / reduction of Chinese exports to EU). In the second stage, industrial production was severely affected due to restrictive measures, lockdowns, and border closures.
Compared to the previous economic crises, the pandemic one is different because production and economic activities have been partially or totally interrupted in several geographical areas; actions such as lockdown, closing borders, imposing travel restrictions prevented the development of certain activities involving travel, the shocks manifesting themselves simultaneously, both on the demand side and on the supply side. During the economic crisis of 2008, the main challenges were related to financing, with many companies reducing the working hours and workers’ wages. However, compared to the current crisis, during the economic crisis of 2008 the companies did not have to close.
With a view to the importance of economic sentiment, Tonkiss (2009) stated that the confidence in the economy promotes economic efficiency by reducing the transaction costs of economic exchange, based on the assumption that the behaviour of other individuals will be in accordance with the common rules of economic conduct. Therefore, good management of public confidence becomes essential, because economic uncertainty is also positively linked with recession risk. Reducing uncertainty in the economy can be also seen as an action to stimulate economic efficiency (Tonkiss, 2009).
The relationship between the trust of economic agents and the real economy is a topic of great interest for policy makers. In this context, confidence, insofar as it affects consumer economic choices, savings, investment, etc., has an influence on both the current state of the economy and the prospects for economic growth, but also confidence indices depend on the evolution of certain economic and financial indicators. (Castellanos-García et al. 2014).
According to the ECB (2013), the link between confidence indicators and economic activity is not simple, but may be more relevant in times of crisis; a significant deterioration in confidence may have some predictive power over future economic development leading to a decline in production. In addition, Stiglitz (2008) considers that the main cause that supported the economic crisis of 2008 was the decline of trust.
As a potential response to the current crisis, the transition to the green economy may be a good way forward since air pollution and a low quality environment may be harmful for human health conditions, but also for companies (including these operating in the industrial sector). This conclusion has been drawn by several authors (Colli, 2020; Gupta et al., 2020; Frontera et al., 2020; Coccia, 2020a; Coccia, 2020b) proving that air pollution and unsustainable environment led to an increase in the number of COVID-19 infections and intensified the severity of the cases registered in the polluted areas.
Methodology
In this section we have described the methods used to estimate the impact of the COVID-19 crisis (an event captured by a binary variable which depends on the restrictions applied in the context of the coronavirus pandemic) on the industrial production (Model A), respectively on the confidence in the industrial sector (Model B).
In the analysis, we used panel data with monthly frequency for the period January 2018 – November 2020, to capture the pre-crisis (COVID) period and the COVID crisis. The statistical data series were collected from the Eurostat platform for all 27 Member States (the United Kingdom was excluded from the analysis in the context of Brexit).
According to the previous mention, the shock generated by the SARS-CoV-2 virus has been identified by a dummy variable taking into account the occurrence of the shock in each Member State, starting with the application of severe restrictions. In this context, we used the value 0 to capture pre-COVID crisis period (when the economy operated under normal conditions), while the value 1 has been used to capture the exceptional conditions generated by the COVID crisis (specifically – the period of application of restrictions to limit the spread of the virus). Although in many cases the restrictions were lifted, the value 1 was kept until the end of the analysed period to catch the persistence of the low confidence in the economy, which is specific to crisis conditions. The information on restrictions were collected using the available data on the national government websites, European Commission, and those available on other official international news sources (e.g., Financial Times, Reuters). Therefore, we set the starting point of COVID-19 shock to March 2020 for all Member States, excepting the case of Italy (where the COVID-19 shock has been manifested since February 2020) and Sweden (which started to apply restrictions later, in May 2020).
Regarding the estimated models, we used the Estimated Generalized Least Squares method (EGLS) weighted with the Cross-section SUR option, since the number of cross-section observations is greater than the number of cross-sections (Cross-section SUR has also the advantage of limitating the issues related to heteroskedasticity and those related to cross-section dependence). In the case of Model A, we used a model with fixed effects, consistent with the result of the Redundant Fixed Effects Likelihood Ratio test. However, in the case of Model B, it was not possible to apply the Cross-section SUR option (to facilitate the ex-ante removal of heteroskedasticity and cross-section dependence), simultaneously with the application of the fixed effects model, due to the use of unbalanced panel data in this model. In this context, a compromise has been needed between these two calculation techniques. Therefore, we chose a model without effects, using the Cross-section SUR option, since the presence of heteroskedasticity and cross-section dependence could raise additional issues in panel estimation techniques.
For Model A (918 observations), we have applied the estimation method indicated above on the following equation:

, where: indprodcal represents the percentage change of production in industry (calendar adjusted data) compared to the same period of the previous year; coviddummy is the COVID-19 restriction dummy variable; and d(hicpindustry) represents the first difference of the annual percentage change of the industrial goods prices.
On the other hand, the Model B (882 observations) has been structured as follows:

, where: is the confidence in the industrial sector (index); represents the indicator used in Model A lagged by 1 year; is the first difference of the consumer confidence lagged by one year; while and have been described above.
Subsequently, we have performed the necessary tests to verify the assumptions needed to confirm the accuracy of the models, as follows:
- the significance of the parameters;
- the absence of multicollinearity;
- the absence of heteroskedasticity – Breusch-Pagan-Godfrey test;
- the absence of autocorrelation of residuals – Breusch-Pagan test;
- the absence of cross-section dependence – Breusch-Pagan LM, Pesaran scaled LM, and Pesaran CD tests;
- the normal distribution of residuals – Jarque-Bera test.
In the analysis, we have also examined other relevant indicators in the context of COVID-19, such as total COVID cases / deaths and mortality rate (the share of deaths in total cases), which will be presented in the next section.
Results and interpretations
In this section, we have analysed the impact of the COVID-19 crisis on industrial production and on confidence in the industrial sector in EU-27 countries. However, first, we examined the evolution of COVID-19 cases and deaths (per million inhabitants). As can be seen in Figure 1, the most affected Member States in terms of the incidence of COVID-19 cases at the end of 2020 were Luxembourg (74148 cases per 1 million inhabitants), Czechia (67108 cases per 1 million inhabitants) and Slovenia (58757 cases per 1 million inhabitants).
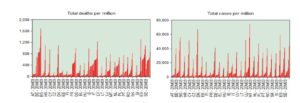
Figure 1: Total deaths / cases per million population
Source: Authors’ own calculations in Eviews 9.0 using Our World in Data statistics
However, the situation is quite sensitive to the ability of Member States to test the population. In this context, it can be mentioned that Luxembourg is a state with a small population, and testing a larger percentage of the population is much easier to perform (Luxembourg ranks third in the world from the perspective of testing capacity – 3194940 tests performed per 1 million inhabitants on 17 February 2021, according to Worldometers – indicating that this Member State tests about 3 times a person). On the other hand, the incidence of cases is more appropriate to the reality in Czechia and Slovenia, which ranks 35th and 59th from the perspective of the tests performed per million inhabitants.
Following the examination of the number of deaths per million inhabitants, it can be seen that the most affected Member States in the EU, at the end of 2020, were Belgium (1685 deaths per 1 million inhabitants), Slovenia (1297 deaths per 1 million inhabitants) and Italy (1277 deaths per 1 million inhabitants), which are also ranked in the top seven countries globally from the point of view of this indicator, according to the data provided by Worldometers. This confirms the challenges that these countries have faced, especially those that have resulted from hospitals overwhelming.
On the other hand, the mortality rate calculated as a percentage of the total number of deaths in the total number of cases (Annex 1) demonstrates that, in 2020, the period April-June was the most difficult one, with several Member States accounting a COVID-19 mortality rate higher than 11%. In this regard, we may look to the epidemiological developments in Belgium, which also registered the highest mortality rate among EU Member States (16.2% – May 2021), followed by Italy (14.4% – June 2021), Hungary (14.1% – June 2021) and Spain (11.5% – April 2021). Starting with March, Member States began to impose severe restrictions, and the effects on the new COVID-19 cases / deaths became visible after May-June, when the mortality rate entered on a downward path. The cause of this reduction was mainly determined by the decrease in the number of new COVID-19 cases, respectively by the reduction of the hospital overwhelming, which also generated a marginal increase in the capacity of medical staff to save patients with severe forms of COVID-19.
Although the intervention of national governments was necessary to limit the increase in the number of new infections and, implicitly, the mortality rate, this generated negative effects in the economy, industry being one of the sectors strongly affected by the COVID crisis. In April (following the effects of the restrictions that have been in place since March), 14 Member States experienced a decrease in the industrial production compared to the same month of the previous year of more than 20%, a contraction that can also be seen in Annex 2. According to Annex 2, the largest decreases in industrial production were recorded in April, in Italy (-43%), Slovakia (-42%), Romania (-39%), and Hungary (-37%), while the industrial sectors of Latvia, Malta, Finland and the Netherlands were less vulnerable to the COVID-19 shock. Annex 3 highlights the high correlation between COVID-19 shock and declining confidence in industry, this being one of the reasons why we chose to examine both the impact of COVID shock on industrial production and the one on the confidence in this sector.
Model A refers to the impact of the crisis generated by the SARS-CoV-2 virus on industrial production (Figure 2). The results show that a prolonging of the COVID-19 shock for another month led to a decrease in the percentage change of industrial production by 8.156 percentage points. The appearance and the wide spread of the SARS-CoV-2 virus led to the temporary closure of several economic sectors, including the industrial sector, which led to significant losses for the economy.
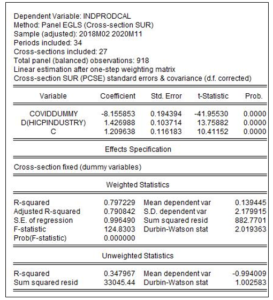
Figure 2: The impact of COVID-19 shock on industrial production (Model A)
Source: Authors’ own calculations in Eviews 9.0 using Eurostat data
Another conclusion is that the increase by one deviation point in the first difference of the annual percentage change of industrial goods prices led to an increase in the dynamics of industrial production by 1.427 percentage points. The increase in the prices of industrial products is considered by companies a source of profit, these being incentivised to invest and produce at a faster pace, compared to the increase in the industrial goods prices. This argument also demonstrates the theory proving that industrial products have an elastic price responsiveness of supply.
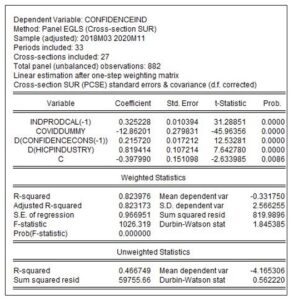
Figure 3: The impact of COVID-19 shock on confidence in industry (Model B)
Source:Authors’ own calculations in Eviews 9.0 using Eurostat data
In the second model (Model B), we calculated the impact of the crisis generated by COVID-19 on confidence in the industrial sector (Figure 3). The results show that the increase by one percentage point in the percentage change of industrial production led to an increase in the industrial confidence index by 0.325 deviation points, the effect manifesting after one month lag. The growth of industrial production is generally based on new investments and supported by involvement in research and development, which generates greater confidence in this sector.
The results also show that the COVID-19 shock led to a decrease in the industrial confidence index by 12.862 deviation points each month (on average). The extension of the containment measures implemented in the context of the COVID-19 pandemic facilitated the emergence / maintenance of negative expectations from the economy / industry. On the other hand, the increase by one deviation point of the first difference in the consumer confidence index lagged by one month led to an increase of the industrial confidence index by 0.216 deviation points. The current crisis that EU Member States are facing has fueled economic uncertainty in households, and individuals have tried to save more and reduce their consumption, which has reduced the demand for industrial products and limited the growth of industrial production.
At the same time, according to the results, the increase in the first difference of the annual percentage change of industrial goods prices (lagged by one month) by one deviation point led to the increase of the industrial confidence index of 0.819 percentage points. The increase in the industrial goods prices boosts the confidence of economic agents in this sector, since high prices make producers increase their supply to obtain higher profits.
According to the results, all estimators (in both models) are statistically significant at 5%, and the high values of R-squared confirm the appropriate choice of the regressors (79.72% – Model A; 82.39% – Model B). In addition, the probability of the Fisher test is 0% for both models, which confirms their statistical validity.
To confirm the homoskedastic feature of both models (Table 1), we used the Breusch-Pagan-Godfrey (BPG) test. In this context, in the case of Model A, we found a probability associated with the BPG test of 53.98%; and 6.63% for Model B, both results confirming the absence of heteroskedasticity. In order to verify the autocorrelation of the residuals, we used the Breusch-Pagan (BP) test, which provided a probability of 6.40% for Model A and 1.72% for Model B. The results of this test show that at the level of Model A there is no autocorrelation between residuals, even if it has been found in the case of Model B. However, in Panel technique, autocorrelation is not a significant issue and may be ignored if the model meets the other criteria used in the robustness checking process.
Table 1: Tested hypotheses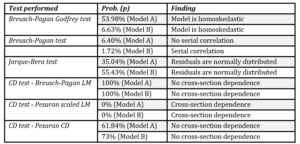
Source: Authors’ own calculations in Eviews 9.0 using Eurostat data
Subsequently, we used the Jarque-Bera test to check the normality of the residuals, the probability associated with it being 35.04% for Model A and 55.43% for Model B. Given that their values are higher than 5%, the hypothesis that the residuals are normally distributed has been accepted.
The hypothesis of no cross-section dependence has been confirmed following the use of Pesaran CD, Pesaran scaled LM, and Breusch-Pagan LM tests. According to Table 1, most of the probabilities associated to these tests (which are higher than 5%) confirmed the absence of cross-section dependence. In addition, the absence of multicollinearity has been also confirmed, since the correlation coefficients between the exogenous variables are lower than R-squared, in the case of both models. Therefore, following the tests performed, we found that the impact coefficients are robust in both models, with the only mention that, in Model B, there are some limitations caused by the occurrence of the autocorrelation between residuals.
Conclusions
The COVID-19 crisis started suddenly, while European Union Member States were not prepared for a pandemic evolution. The rapid spread of the outbreak raised the need for urgent, coordinated, and unitary action at EU level. In this context, EU Member States applied several containment measures, which also affected economic developments and individual expectations, including industrial production and confidence in industry.
Following the lockdowns, industrial production dropped sharply and highlighted the challenges related to low economic resilience in most of the EU countries, while national governments directly supported the sectors severely affected by the containment measures to maintain the survival chances of the economic operators. Our estimation showed that the COVID-19 shock led to the contraction of the EU industrial production with 8.156 percentage points (on monthly average). According to the data, the largest falls of industrial production were found in the case of Italy, Slovakia, Romania, and Hungary, while Latvia, Malta, Finland, and the Netherlands experienced moderated shocks.
In the second econometric model performed, we found that the COVID-19 shock reduces dramatically the confidence in the industrial sector. According to our results, the shock caused a shrink of the EU industrial confidence index by 12.862 deviation points. We have also found a positive relationship between the confidence in the industrial sector and the consumer confidence, since any drop in the demand will limit the producers supply.
The way forward is still uncertain and many questions remain without answer. European Union should focus on prevention by promoting the strengthening of resilience at the country level. A higher resilience will help EU countries to better cope with other possible crisis events. In this context, European Commission started to focus on the digital and green transition, but also keep the objective related to social inclusion, which are important drivers of resilience. Member States should also invest in health and education systems and should create and make use effectively of long-term strategies. Fiscal and budgetary planning could also increase the resilience, but this depends on the extent to which the national governments promote anti-cyclical policies by creating fiscal buffers in good economic times and using them in bad economic times.
Acknowledgment
This paper was co-financed by The Bucharest University of Economic Studies during the PhD program.
(adsbygoogle = window.adsbygoogle || []).push({});
References
- Behun, L., Smetana, M. and Capoca, K. (2018), ‘Comparison of detection abilities between fluxgate and GMR magnetometer în inverse ECT of deep lying cracks’, Proceedings of the 2018 ELEKTRO, 21-23 May2018, Mikulov, Czech Republic, 1-4.
- Badkar, M. and Greeley, B. (2020), ”New data shows vast scale of US economic breakdown,”Financial Times, [Online], [Retrieved December 4, 2020], https://www.ft.com/content/6361247d-a1df-4a50-b0aa-59395801b3f3
- Cai, M. and Luo, J. (2020), ‘Influence of COVID-19 on manufacturing industry and corresponding countermeasures from supply Chain perspective’, Journal of Shanghai Jiaotong University (Science), 25(4), 409 – 416.
- Cantore, N., Hartwich, F., Laplane, A., Larsen, J., Lavopa, A. and Rodousakis N. (2020), ”Coronavirus: the economic impact,” United Nations Industrial Development Organization”, [Online], [Retrieved December 4, 2020], https://www.unido.org/stories/coronavirus-economic-impact-21-october-2020
- Castellanos-García, P., Pérez-del-Río, I. and Sánchez-Santos, J. M. (2014), ‘The role of confidence in the evolution of the Spanish economy: empirical evidence from an ARDL model’, European Journal of Government and Economics, 3(2), 148-161.
- Coccia M. (2020a), ‘Factors determining the diffusion of COVID-19 and suggested strategy to prevent future accelerated viral infectivity similar to COVID’, Science of the Total Environment, 729, 138474.
- Coccia, M. (2020b), ‘How (un)sustainable environments are related to the diffusion of COVID-19: the relation between coronavirus disease 2019, air pollution, wind resource and energy’, Sustainability, 12(22), 9709.
- Colli, F. (2020), ‘The end of ‘business as usual’?: COVID-19 and the European Green Deal’, Institut Royal des Relations Internationales (Egmont Institute) / Koninklijk Instituut voor Internationale Relaties, 60, 5.
- ECB (2013), ”Confidence indicator and economic developments”, in ECB Monthly Bulletin, January 2013, [Online], [Retrieved November 22, 2020],
- https://www.ecb.europa.eu/pub/pdf/other/art1_mb201301en_pp45-58en.pdf
- Eurostat (2021), ‘Eurostat database’, available at: https://ec.europa.eu/eurostat
- Frontera, A., Martin, C., Vlachos, K. and Sgubin, G. (2020), ‘Regional air pollution persistence links to COVID-19 infection zoning’, Journal of Infection, 81(2), 318–356.
- Gupta, A., Bherwani, H., Gautam, S., Anjum, S., Musugu, K., Kumar, N., Anshul, A. and Kumar, R. (2020), ‘Air pollution aggravating COVID-19 lethality? Exploration in Asian cities using statistical models’, Environment, Development and Sustainability, 22(5), 1-10.
- Gutu, L. M., Străchinaru, A. I., Ilie,V. and Străchinaru, A. V, (2015), ‘The macroeconomic variables’ impact on industrial production in the context of financial crisis’, Procedia Economics and Finance, 32, 1258-1267.
- International Labour Organisation Monitor, (2020), ”COVID-19 and the world of work (2nd edition),” [Online], [Retrieved December 19, 2020], https://www.ilo.org/global/about-the-ilo/WCMS_740877/lang–it/index.htm
- Ivanov, D. and Dolgui, A. (2020), ‘Viability of intertwined supply networks: extending the supply chain resilience angles towards survivability. A position paper motivated by COVID-19 outbreak’, International Journal of Production Research, 58(10), 2904-2915.
- Lopez-Gomez C., Corsini L., Leal-Ayala D. and Fokeer S. (2020), ”COVID-19 critical supplies: The manufacturing repurposing challenge”, United Nations Industrial Development Organization, [Online], [Retrieved March 4, 2021], https://www.unido.org/news/covid-19-critical-supplies-manufacturing-repurposing-challenge
- Malgarini, M. (2011), ‘Industrial production and confidence after the crisis: what’s going on?’, University Library of Munich,
- Rapaccini, M., Saccani, N., Kowalkowski, C., Paiola, M. and Adrodegari, F. (2020), ‘Navigating disruptive crises through service-led growth: The impact of COVID-19 on Italian manufacturing firms’, Industrial Marketing Management, 88, 225-237.
- Romih, D. and Kavkler, A. (2020), ‘The impact of economic policy uncertainty on industrial production in Germany’, Economic and Social Development (Book of Proceedings), 339-342.
- Roser, M., Ritchie, H, Ortiz-Ospina, E. and Hasell, J. (2020), ”Coronavirus Pandemic (COVID-19)”, [Online], [Retrieved January 24, 2021], https://ourworldindata.org/coronavirus
- Stiglitz, J., (2008), ”The fruit of hypocrisy”, The Guardian, [Online], [Retrieved January 13, 2021],
- https://www.theguardian.com/commentisfree/2008/sep/16/economics.wallstreet
- The Economist (2020), ”COVID-19: The impact on industry Report”, The Economist Intelligence Unit report.
- Tonkiss, F. (2009), ‘Trust, confidence and economic crisis’, Intereconomics: Review of European Economic Policy, 44, (4), 196-202.
- World Health Organization (2020), ‘WHO Director-General`s statement on IHR emergency committee on novel coronavirus (2019-nCoV)’, [Online], [Retrieved January 14, 2021] available: https://www.who.int/director-general/speeches/detail/who-director-general-s-statement-on-ihr-emergency-committee-on-novel-coronavirus-(2019-ncov)
- Worldometers (2021), ‘COVID-19 coronavirus pandemic’,
- https://www.worldometers.info/coronavirus/
- Wuest, T., Kusiak, A., Dai, T., Tayur, S., (2020), Impact of COVID-19 on manufacturing and supply networks – the case for AI-inspired digital transformation, Electronic Journal, 47.
Annexe
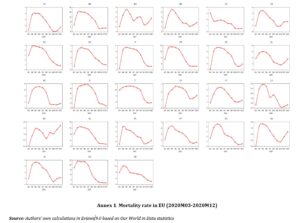
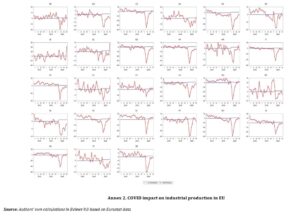