Introduction
There are popular ojek-hailing applications in Indonesia, including Gojek the pioneer, followed by Grab Bike and Uber. Ojek can be considered as an authentic public transport of Indonesia. Ojek is a local name for a motorbike taxi. Using a motorbike, an ojek driver will deliver a client from one place to another with an agreed price beforehand. This individual public transport-type has existed for decades in almost all parts of Indonesia. Furthermore, the establishment of an online application for the ojek hailing has caused a change of consumer behaviour. Previously, clients should visit a shelter where ojek drivers wait and negotiate the price with the driver. For regular trips, prices are fixed. For unusual destinations, a negotiation is needed. Sometime, if the drivers dealt with strangers, they gave a very high price. Practically the traditional way for hailing a taxi is uncomfortable and inefficient (He and Shen 2015). The current applications, as we know, are different. They reduce price and the average wait time for clients (Soota 2016).
The ojek-hailing application is considered as a form of sharing economy. The concept of sharing economy or collaborative consumption refers to “the peer to-peer-based activity of obtaining, giving, or sharing the access to goods and services, coordinated through community-based online services” (Hamari, Sjöklint et al. 2015). There are four categories of sharing economy according to Schor (2014), including “recirculation of goods, increased utilization of durable assets, exchange of services, and sharing of productive assets”. Some authors have predicted that there would be a clash between sharing economy-based service providers and the industry as the existing industry faced a decrease of profitability (Zervas, Proserpio et al. 2014). It also occurred in Indonesia, where conventional ojek drivers protested and demanded the government to protect them (Wijaya 2016).
This study aims to test the theory of technology acceptance model (TAM) that is used to predict the intention of a person to use the ojek-hailing app. There is a study relating to a taxi-hailing-app in the service marketing field conducted by Liu (2014). However, there is a paucity of research focussing on an ojek-hailing app. Besides, TAM has been employed by many researchers in various settings of technology application, such as gameplay, tablet PC, mobile money, and e-learning (Park and del Pobil 2013; Mbengo 2014; Suhud and Hidayat 2015; Wang and Sun 2016).
Literature Review
Theoretical Background
In life, people will face a certain technology and this technology might change in certain periods of time. Some will adapt, then adopt sooner or later, and some others, for a myriad of reasons, will avoid. As mentioned earlier, TAM has been used by researchers to predict a person’s intention to adopt a certain technology both in an original model or modified model. In the original version, Davis, Bagozzi et al. (1989) included the variables: external variables, PEOU, PU, attitude towards usage, intention to use, and actual system usage. In more details, here are the links: Actual system usage is influenced by intention to use; intention is influenced by PU and attitude towards usage; attitude is influenced by PEOU and PU; PU is influenced by PEOU and external variables; and PEOU is influenced by external variables. Davis, Bagozzi et al. (1989) mentioned that PEOU is similar to self-efficacy while PU is defined by these scholars as “the degree to which a person believes that using a particular system would enhance his or her job performance”.
Theoretical Framework
TAM has been employed by prior studies in various settings of technology apps. Fleischer and Wåhlin (2016) chose Generation Y as the subject of their study. According to these researchers, the attitude of Generation Y towards usage of a taxi-hailing app influenced intention to use and further, the attitude towards the usage was influenced by PEOU and usefulness. While Fleischer and Wåhlin (2016) collected data in Jönköping, Sweden, Liu (2014) collected data in Shanghai, China. Her study also focussed on the use of a taxi-hailing app. She found that PEOU influenced PU and attitude, PU influenced behavioural intention and attitude, and attitude influenced behavioural intention.
The use of e-learning in Zimbabwe was explored by Mbengo (2014). This researcher recorded that PEOU significantly affected intention and perceived of usefulness, and perceived of usefulness significantly affected attitude. Another study on e-learning was presented by Elkaseh, Wong et al. (2016). Their study focussed on students and teachers’ behavioural intention to adopt e-learning using social networking media. They carried out findings that suggested that attitude towards usage was influenced by PEOU and PU and attitude was directly influenced by intention to use this technology. Furthermore, Park (2009) concluded that attitude could affect the intention to use an e-learning system while attitude was effected by PEOU and PU. In addition, PEOU affected PU. While Park (2009) took place in Korea, Al-Alak and Alnawas (2011) took place in Jordan. These researchers demonstrated a significant impact of PEOU and PU on behavioural intention to adopt e-learning. Another study that took place in Korea was done by Kim (2006). That study focused on e-learning community for preparatory teachers and carried out findings: PEOU and PU influenced attitude and attitude influenced intention.
Online shoppers’ intention behaviour was investigated by Cho and Sagynov (2015). According to these researchers, PEOU had a significant effect on PU and intention to use while PU also had a significant effect on intention to use. Taking place in Malaysia, Ramayah and Ignatius (2005) surveyed staff of a public institution to understand their intention to shop online. They showed that intention to shop online was influenced by PEOU while PEOU influenced PU.
Another study that was conducted in Malaysia is reported by Lim and Ting (2012) who surveyed a mall visitors. These researchers stated that PEOU influenced PU and attitude while attitude influenced intention. Other findings they carried out were that PU influenced attitude. Renny, Guritno et al. (2013) studied the intention of consumers to shop online. One of the findings showed that PU was an important key to predict attitude.
Furthermore, Cho and Sagynov (2015) involved students and employees, and recorded that PEOU and perceived of usefulness impacted intention to shop online. Furthermore, Juniwati (2014) involved unexperienced university students in her study. She found that attitude towards technology usage was influenced by PEOU and usefulness, and behavioural intention was influenced by attitude.
Suki and Suki (2011) found that PEOU and PU had a significant impact on intention to use a 3G mobile service. A website behavioural revisit intention has intrigued Hallegatte and Nantel (2006). As reported by these researchers, perceived ease predicted PU and behavioural intention. In addition, PU significantly predicted behavioural intention. Internet users, consisted of students and working professionals, were approached conveniently by Rizwan, Umair et al. (2014). They showed the significant impact of PEOU on attitude and PU. Taking place in Thailand, Rotchanakitumnuai (2005) selected securities investors to test their intention to use online securities trading. As they reported, attitude towards usage and PU were significantly correlated with intention to use. Kanchanatanee, Suwanno et al. (2014) investigated intention of small and medium sized business owners in Thailand to use e-marketing. They included various variables, namely internal and external factors, ease of use, relative advantage, compatibility, and attitude towards behaviour to predict intention. They noted that attitude was the most influential factor to impact behavioural intention.
Another two findings included a significant impact of PEOU on attitude and perceived of usefulness. Furthermore, a study on e-government was conducted by Hamid, Razak et al. (2016). Employees from a public institution were included to test whether TAM could predict their intention to use e-government app. This study pointed out that PEOU and PU could predict intention to use.
This study is addressed to examine the theoretical framework as illustrated below:
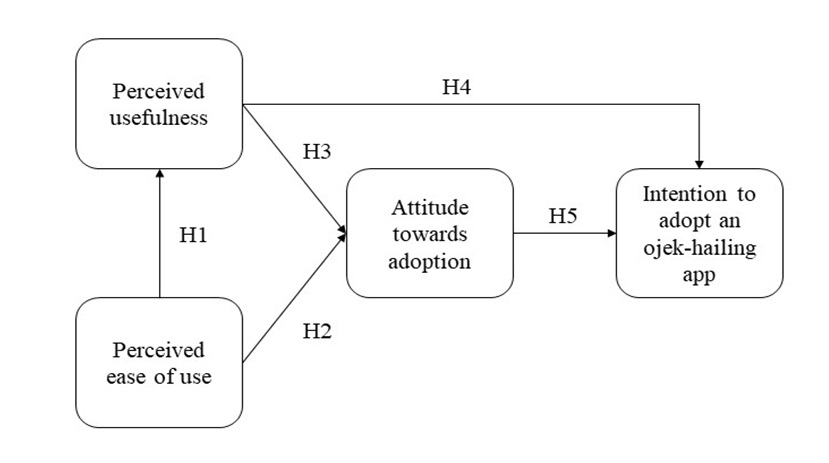
Fig. 1: The theoretical framework
Furthermore, based on the framework above, the following six hypotheses will be tested:
H1: PEOU will influence a person’s PU
H2: PEOU will influence a person’s attitude towards using an ojek online application
H3: PU will influence a person’s attitude towards using an ojek online application
H4: PU will influence a person’s intention to use an ojek online application
H5: Attitude towards using an ojek online application will influence a person’s intention to use an ojek online application
Methodology
Participants
In total, 200 participants involved in a self-administered survey consisting of 130 females (65%) and 70 males (35%). Predominantly, respondents were aged between 20-30 years old (176 – 88%) and were students (150 – 75%) followed by employees (37 – 18.5%). All respondents claimed that they had an experience downloading one or more ojek-hailing online apps. They mentioned that the apps they downloaded were Gojek (182 – 91%), Grabbike (153 – 76.5%), Bluejek (5 – 2.5%), Ladyjek (3 – 1.5%), and Uber (22 – 11%). Besides, all respondents indicated that they also had had an experience using the apps and the service of the ojek. Another note that can be presented here is the frequency of usage. Respondents showed they used the service as once in a day (16 – 8%), twice in a day (4 – 2%), once in 2-3 days (8 – 4%), 2 – 3 times in a week (29 – 14.5%), once in a week (8 – 4%), and uncertain (135 – 67.5%).
Instrument Development
The instrument contains indicators to measure attitude, PU, perceived ease to use, and usage intention adapted from Liu (2014), Peng, Wang et al. (2014), and Zarrad and Derabi (2012).
Data Analysis
Data were collected using exploratory and confirmatory factor analyses. For exploratory factor analysis, indicators with loadings of 0.4 or greater are selected. This condition is required as the number of the sample for this study of 200 (Hair Jr., Black et al. 2006). To guarantee its reliability, the authors choose only dimensions with a score of 0.7 and greater to be used for confirmatory factor analysis (Hair Jr., Black et al. 2006). Based on the reliability calculation, all dimensions are considered reliable. The next action is conducting confirmatory factor analysis to measure the proposed research framework. A fitted framework would be considered if the model will have a probability of 0.05 (Schermelleh-Engel, Moosbrugger et al. 2003), CMIN/DF of ≤ 2 (Tabachnick and Fidell 2007), CFI of ≥ 0.97 (Hu and Bentler 1995), and RMSEA of ≤ 0.05 (Hu and Bentler 1999).
Results
Exploratory Factor Analysis
Exploratory factor analysis produces eight dimensions. Attitude consists of two dimensions: ‘consistency’ (five indicators with α= 0.888) and ‘direction’ (three indicators with α= 0.808). PU consists of two dimensions: ‘improves job performance’ (four indicators with α= 0.864) and ‘enhance effectiveness’ (eight indicators with α= 0.912). PEOU retains ten indicators with α= 0.900. Behavioural intention forms three dimensions: ‘pay more’ (four indicators with α= 0.875), ‘external response’ (two indicators with α= 0.725), and ‘loyalty’ (two indicators with α= 0.773).
Table 1: Result of exploratory factor analysis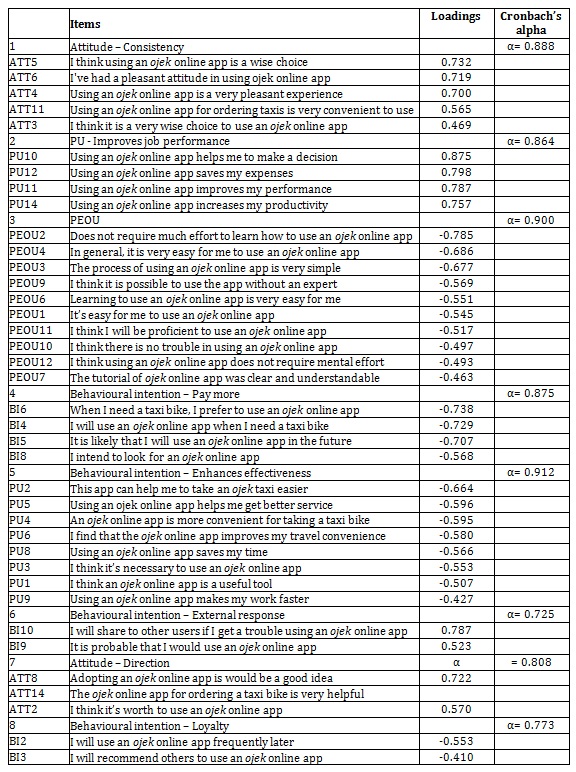
Hypotheses Testing
The table below presents a fitted model resulted by structural equation model. This model has a probability score of 0.062, CMIN/DF score of 1.339, CFI score of 0.985, and RMSEA score of 0.041. These scores are significant with the score stated by Schermelleh-Engel, Moosbrugger et al. (2003), Tabachnick and Fidell (2007), Hu and Bentler (1995), and Hu and Bentler (1999) respectively.
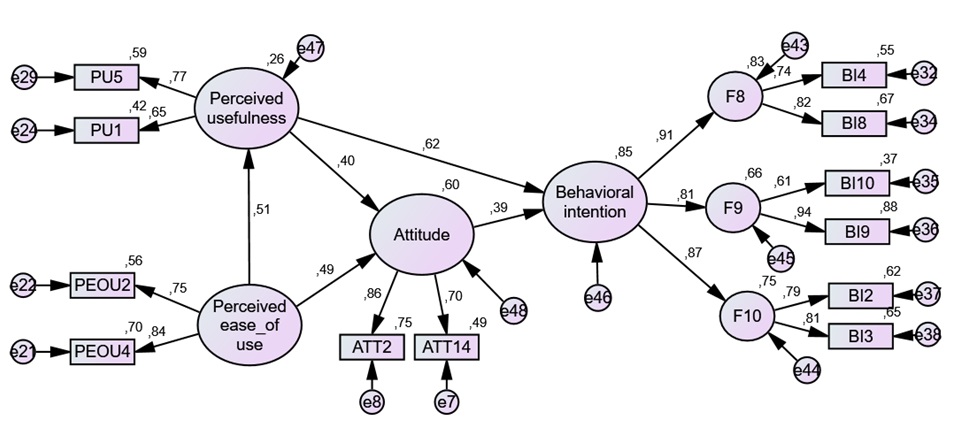
Fig. 2: Structural model of hypotheses testing
As seen in the table below, all hypotheses inspected are significant with a C.R. score greater than 1.96 as requested (Byrne 2001; Holmes-Smith 2010).
Table 2: Summary of the hypotheses testing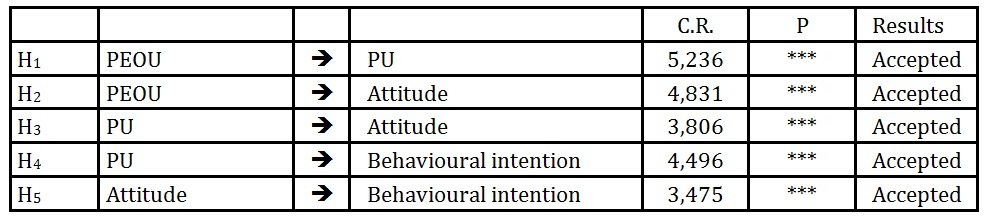
Discussion
This study tested TAM, and the results of this study showed that TAM could be used to test the consumer behaviour of an application-based taxi. All hypotheses tested showed significance. The first hypothesis predicted the impact of PEOU on PU. H1 was accepted with a C.R. score of 5.005. This finding was significant with the theory documented by Lim and Ting (2012), Liu (2014), and Rizwan, Umair et al. (2014). Further, the second hypothesis predicted the impact of PEOU on attitude. In this study, this path owned a C.R. score of 4.870 which indicates significance. This finding supported prior studies reported by Fleischer and Wåhlin (2016), Juniwati (2014), and Kim (2006), Lim and Ting (2012), Liu (2014), and Park (2009).
Prior studies (Kim 2006; Park 2009; Lim and Ting 2012; Renny, Guritno et al. 2013; Juniwati 2014; Liu 2014; Fleischer and Wåhlin 2016) hypothesised that PU would significantly impact attitude towards usage. This third hypothesis was accepted with a C.R. score of 3.894. According to prior studies (Al-Alak and Alnawas 2011; Liu 2014; Hamid, Razak et al. 2016), PU would impact intention to use. This path in this study became the fourth hypothesis. Based on the calculation, this path had a C.R. score of 4.516. Therefore, H4 was accepted. The last hypothesis was attitude toward usage would impact intention to use an ojek-hailing online app. This path produced a C.R. score of 2.530 and therefore, H5 was accepted. This finding supported existing studies (Kim 2006; Park 2009; Lim and Ting 2012; Juniwati 2014; Liu 2014; Fleischer and Wåhlin 2016).
Conclusion
This study examined the intention of young consumers to use an ojek-hailing online app by applying TAM. To conclude, TAM can predict ojek-hailing online apps usage intention. PU and attitude were significantly influenced by PEOU. Attitude towards ojek-hailing online apps and behavioural intention were significantly influenced by PU and attitude towards ojek-hailing online apps.
This current study employed convenience sampling method. Therefore, results of the study cannot generalise all subscribers of the taxi-hail apps. This can be considered as one of the limitations. Another limitation of this study is the absence of other predictor variables, for example, compatibility, perceived ease, trust, subjective norm, and innovativeness (Cho and Cheung 2003; Rose and Fogarty 2006; Bigne-Alcaniz, Ruiz-Mafé et al. 2008; Nasri, Lanouar et al. 2013; Kim and Woo 2016). Therefore, future study should pay attention to this area. It is also recommended to distinguish participants with pre-paid and post-paid Internet subscribing. Similar studies may also be conducted within different areas to explore any cultural differences in results. Concerning the research model being tested, this study does not provide any additional variables other than those variables that are part of the TAM model. This fact can be a weakness of this research. Therefore, by adding another variable as a predictor, it will contribute differently to expand the literature of sharing economy.
This study carries out a message for practitioners in the taxi-hail app-relating industry. Respondents perceived that the taxi-hailing online applications are still uneasy to use. Indeed, as the apps are Internet-based, they can be used only if the users have an Internet connection. In Indonesia, mobile users have two options to obtain an Internet connection: pre-paid and post-paid. For those who chose pre-paid scheme, they are required to have credits on their accounts. The app providers might pay attention on this part.
(adsbygoogle = window.adsbygoogle || []).push({});
References
- Al-Alak, B. A. and I. A. M. Alnawas (2011). “Measuring the acceptance and adoption of e-learning by academic staff.” Knowledge Management & E-Learning: An International Journal 3(2): 201-221.
- Bigne-Alcaniz, E., C. Ruiz-Mafé, et al. (2008). “Influence of online shopping information dependency and innovativeness on internet shopping adoption.” Online Information Review 32(5): 648-667.
- Byrne, B. M. (2001). “Structural equation modeling with AMOS, EQS, and LISREL: Comparative approaches to testing for the factorial validity of a measuring instrument.” International Journal of Testing 1(1): 55-86.
- Cho, V. and I. Cheung (2003). “A study of on-line legal service adoption in Hong Kong.” Department of management. The Hong Kong Polytechnic University. Retrieved Jan 20: 2009.
- Cho, Y. C. and E. Sagynov (2015). “Exploring factors that affect usefulness, ease of use, trust, and purchase intention in the online environment.” International Journal of Management & Information Systems (Online) 19(1): 21.
- Davis, F. D., R. P. Bagozzi, et al. (1989). “User acceptance of computer technology: A comparison of two theoretical models.” Management science 35(8): 982-1003.
- Elkaseh, A. M., K. W. Wong, et al. (2016). “Perceived ease of use and perceived usefulness of social media for e-learning in libyan higher education: A structural equation modeling analysis.” International Journal of Information and Education Technology 6(3): 192-199.
- Fleischer, A. and C. Wåhlin (2016). Want to take a ride with me? The intention of generation Y to use Uber. Business Administration. Jönköping, Jönköping University. Master.
- Hair Jr., J. F., W. C. Black, et al. (2006). Multivariate data analysis New Jersey, Prentice-Hall, Inc.
- Hallegatte, D. and J. Nantel (2006). “The intertwined effect of perceived usefulness, perceived ease of use and trust in a website on the intention to return.” The E-Business Review 6: 1-5.
- Hamari, J., M. Sjöklint, et al. (2015). “The sharing economy: Why people participate in collaborative consumption.” Journal of the Association for Information Science and Technology.
- Hamid, A. A., F. Z. A. Razak, et al. (2016). “The effects of perceived usefulness and perceived ease of use on continuance intention to use e-government.” Procedia Economics and Finance 35: 644-649.
- He, F. and Z.-J. M. Shen (2015). “Modeling taxi services with smartphone-based e-hailing applications.” Transportation Research Part C: Emerging Technologies 58: 93-106.
- Holmes-Smith, P. (2010). Structural equation modeling: From the fundamentals to advanced topics. Melbourne, SREAMS (School Research Evaluation and Measurement Services)
- Hu, L.-t. and P. M. Bentler (1995). Evaluating model fit. Structural equation modeling. Concepts, issues, and applications. R. H. Hoyle. London, Sage: 76-99.
- Hu, L.-t. and P. M. Bentler (1999). “Cutoff criteria for fit indexes in covariance structure analysis: Conventional criteria versus new alternatives.” Structural Equation Modeling: A Multidisciplinary Journal 6(1): 1-55.
- Juniwati (2014). “Influence of perceived usefulness, ease of use, risk on attitude and intention to shop online.” European Journal of Business and Management 6(27): 218-229.
- Kanchanatanee, K., N. Suwanno, et al. (2014). “Effects of attitude toward using, perceived usefulness, perceived ease of use and perceived compatibility on intention to use E-marketing.” Journal of Management Research 6(3): 1-13.
- Kim, S.-W. (2006). “The effect of perceived usefulness and perceived ease of use on learner flow in e-Learning community.” Journal of the Korea Society of Computer and Information 11(6): 87-97.
- Kim, Y. G. and E. Woo (2016). “Consumer acceptance of a quick response (QR) code for the food traceability system: Application of an extended technology acceptance model (TAM).” Food Research International 85: 266-272.
- Lim, W. M. and D. H. Ting (2012). “E-shopping: An analysis of the technology acceptance model.” Modern Applied Science 6(4): 51-62.
- Liu, Z. Y. (2014). An analysis of technology acceptance model – Exploring user acceptance and intension of taxi-hailing app in Shanghai. Department of Computer Science and Engineering. Göteborg, University of Gothenburg. Bachelor
- Mbengo, P. (2014). “E-learning adoption by lecturers in selected Zimbabwe state universities: An application of technology acceptance model.” Journal of Business Administration and Education 6(1): 15.
- Nasri, W., C. Lanouar, et al. (2013). “Expanding the Technology Acceptance Model to Examine Internet Banking Adoption in Tunisia Country.” International Journal of Innovation in the Digital Economy (IJIDE) 4(4): 61-81.
- Park, E. and A. P. del Pobil (2013). “Technology acceptance model for the use of tablet PCs.” Wireless Personal Communications 73(4): 1561-1572.
- Park, S. Y. (2009). “An analysis of the technology acceptance model in understanding university students’ behavioral intention to use e-learning.” Educational technology & society 12(3): 150-162.
- Peng, L., H. Wang, et al. (2014). Exploring factors affecting the user adoption of call-taxi app. 25th Australasian Conference on Information Systems. Auckland, ACIS.
- Ramayah, T. and J. Ignatius (2005). “Impact of perceived usefulness, perceived ease of use and perceived enjoyment on intention to shop online.” ICFAI Journal of Systems Management (IJSM) 3(3): 36-51.
- Renny, S. Guritno, et al. (2013). “Perceived usefulness, ease of use, and attitude towards online shopping usefulness towards online airlines ticket purchase.” Procedia-Social and Behavioral Sciences 81: 212-216.
- Rizwan, M., S. M. Umair, et al. (2014). “Determinants of customer intentions for online shopping: A Study from Pakistan.” Journal of Sociological Research 5(1): 248-272.
- Rose, J. and G. J. Fogarty (2006). Determinants of perceived usefulness and perceived ease of use in the technology acceptance model: senior consumers’ adoption of self-service banking technologies. Proceedings of the 2nd Biennial Conference of the Academy of World Business, Marketing and Management Development: Business Across Borders in the 21st Century, Academy of World Business, Marketing and Management Development.
- Rotchanakitumnuai, S. (2005). “Exploring the antecedents of electronic service acceptance: evidence from Internet securities trading.” Journal of the Computer, the Internet and Management 13(3): 1-6.
- Schermelleh-Engel, K., H. Moosbrugger, et al. (2003). “Evaluating the fit of structural equation models: Tests of significance and descriptive goodness-of-fit measures.” Methods of Psychological Research Online 8(2): 23-74.
- Schor, J. (2014). Debating the sharing economy. Great Transition Initiative.
- Soota, S. (2016). Taxi! Taxi!: The impact of e-hailing apps on the Singapore taxi industry. Economics. Singapore Clairemont Graduate University. Bachelor of Arts.
- Suhud, U. and N. Hidayat (2015). “A mixed-methods study to explore stage of readiness and intention of micro and small enterprises to adopt mobile money in Indonesia.” International Journal of Business, Economics and Management 1(1).
- Suki, N. M. and N. M. Suki (2011). “Exploring the relationship between perceived usefulness, perceived ease of use, perceived enjoyment, attitude and subscribers’ intention towards using 3G mobile services.” Journal of Information Technology Management 22(1): 1-7.
- Tabachnick, B. G. and L. S. Fidell (2007). Using multivariate statistics. Boston Pearson/Allyn & Bacon.
- Wang, Q. and X. Sun (2016). “Investigating gameplay intention of the elderly using an extended technology acceptance model (ETAM).” Technological Forecasting and Social Change 107: 59–68.
- Wijaya, C. A. (2016). Thousands of Jakarta drivers protest against ride-hailing app. The Jakarta Post. Jakarta, Niskala Media Tenggara, PT.
- Zarrad, H. and M. Derabi (2012). “Online purchasing intention: Factors and effects.” International Business and Management 4(1): 37-47.
- Zervas, G., D. Proserpio, et al. (2014). The rise of the sharing economy: Estimating the impact of Airbnb on the hotel industry. Boston U. School of Management Research Paper. Boston, Boston University School of Management