Introduction
Internet has transformed every activity in all the aspects of the human beings’ life (Abiodun, 2013). Accordingly, ways of transaction or purchasing goods have been replaced by online purchasing (Sharma & Lijuan, 2015). Global reach and several other benefits over traditional shopping motivate people to transact online (Alam & Yasin, 2010 and Jiang et al., 2013). A point which is often overlooked is that, people are now reluctant to use online channels for shopping frequently because of the attendant uncertainty and risks (Cha, 2011; Nazir et al., 2012; Meskaran et al., 2013 and Alfina et al., 2014). Under these circumstances, Kim & Forsythe (2010) show that most customers search the internet for product information only without actually purchasing online.
Online companies can implement new strategies to attract potential customers and retain existing customers to maintain and expand their market shares by identifying consumer characteristics which affect their buying behavior (Ahmad et al., 2010 and Adnan, 2014). Many researchers conclude that online buying intention is affected by both technological factors and socio-cultural factors (Abu-shamaa & Abu-Shanab, 2015). Therefore, various studies have examined and recommended to examine the impacts of certain factors on on-line attitudes and behaviors (Cho & Sagynov, 2015).
In essence, consumers’ online purchasing behavior is very complex and personal (Chen et al., 2015). Further, factors that influence online shopping intention are still a matter of debate, and the results from research in this field are inconclusive, often fragmented and not cohesive (Sahney et al., 2013). Hence, the presentation of a comprehensive, integrated model is necessary. Abu-shamaa and Abu-Shanab (2015) argue that online purchasing intention is affected by both technological and socio-cultural factors. Therefore, the attitudes and behaviors of consumers and retailers need to be examined and re-examined in the future (Cho & Sagynov, 2015).
Requirements of the Conceptual Framework
Previous empirical studies employ technology acceptance theories to measure on-line purchase intention in the context of e-commerce. However, technology acceptance models were developed to measure the acceptability of a new technology by new users in an organization, especially those in the computer field. According to Davis et al. (1989); Ajzen (1991); Davis (1989); Taylor and Todd (1995); Venkatesh and Davis (2000); Venkatesh et al. (2003); and Venkatesh et al. (2012), these theories were utilized in every study that involved technology acceptance in the organizational context.
Furthermore, through a closer examination of the existing literature, four major domain areas, namely, consumer characteristics (Chan et al., 2003; Chang, Cheung & Lai, 2005 and Kandambi & Wijenayake, 2017a), retailer characteristics (Chan et al., 2003; Chang et al., 2005 and Kandambi & Wijenayake, 2017b), web characteristics (Chan et al., 2003 and Chang et al., 2005) and social characteristic (Chan et al., 2003 and Chang et al., 2005) can be identified within the concept of e-commerce. According to Ajzen (1991); Davis (1989); Venkatesh et al. (2003); Taylor and Todd (1995); and Venkatesh and Davis (2000), technology acceptance theories have not considered retailer characteristics, website characteristics and some customer characteristics.
The impacts of several constructs of TPB, TRA and TAM such as attitude, subjective norms and perceived behavioral control have been widely studied, while other important factors have been ignored. Thus, according to the analysis of the gap, there has been no proper theory, so far, to explain online purchasing intention in the context of e-commerce.
Objective
Therefore, the time has come for IS researchers to start building their own theories instead of applying theories from other disciplines (Chan et al., 2003). Therefore, IS researchers should work out behavioral models, declaring what is unique and specific to the context of consumer-based electronic commerce (Chan et al., 2003). The main objective is to develop an integrated conceptual framework to explain online purchasing intention in the context of e-commerce.
Conceptual Framework Development Methodology
The sequential process was carried out to develop a concepual framework by four separate studies (see Figure 1). The initial model (Mα) was developed by an exploratory study based on the thematic literature review in study 1. Second, the modified model (Mβ) was generated based on the initial model (Mα), using the main technology acceptance and e-service quality models named as study 2. Third, the modified model (Mβ) was further modified and generated model (Mσ) in study 3, using the Delphi technique. Finally, model (Mμ) was developed from model (Mσ) in study 4, based on interviews with experts.
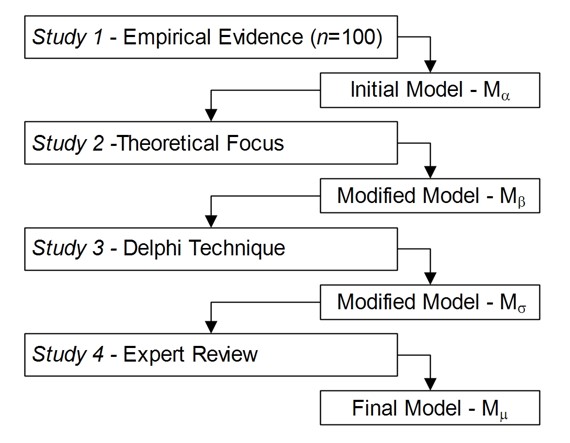
Figure 1: Conceptual Framework Development Process
Source: Developed by the Researcher
Study 1 – Empirical Evidence
Inputs for Study 1
One hundred twelve (n=112) empirical research frameworks were evaluated through a comprehensive thematic literature survey in the exploratory study. That thematic literature review recognized two hundred forty-two (n=242) variables, four significant clusters and relationships among variables with multiple pieces of evidence [hundred (n=100)], and the relationships among the variables were justified by a single piece of evidence [one hundred eighty-one (n=181)] (Kandambi & Wijenayaka, 2017a and Kandambi & Wijenayaka, 2017b).
Variables Identified
Different researchers use different terminologies for the same variable. Variables with the similar working definition are grouped into one category. Among these, three variables (n=3) are in a dependent variable category, and forty (n=40) variables are in an independent variable category.
Table 1: Variable Identification Summary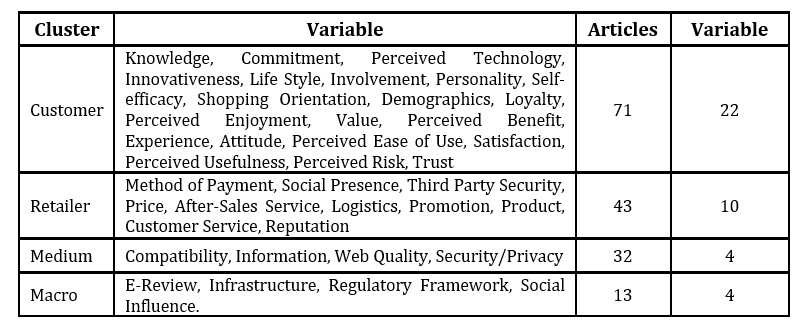
Source: Developed by the Researcher
Twenty-one (n=21) consumer characteristics were identified from seventy-one (n=71) articles. Ten (n=10) retailer characteristics were found from forty-three articles (n=43). Thirty-two (n=32) articles contributed to identify four (n=4) medium characteristics. Thirteen (n=13) articles contributed to identify four (n=4) macro environmental characteristics. Three (n=3) dependent variables (Purchase actual, Purchasing Intention and Repurchase Intention) were extracted.
Cluster Identification
The consumer, the retailer, the web, as a medium of communication between them, and the macro environment, where the dynamics play out, are all involved in the process of ecommerce. The thematic analysis identified four themes, namely, Consumer characteristics as ThemeA with twenty two (n=22) variables, Retailer characteristics as ThemeB with ten (n=10) variables, Medium characteristics as ThemeC with four (n=4) variables, and Macro environmental characteristics as ThemeD with four (n=4) variables.
Retailer characteristics include factors influencing the online consumer behavior such as service quality, privacy and security, control, brand/reputation, delivery/logistics, and after-sales services. Individual or consumer characteristics denote factors specific to the consumer, such as demographics, personality, value, lifestyle, attitude, consumer resources, consumer psychological factors and experience. In addition to personal characteristics, macro environmental characteristics like culture, social influence, peer influence and mass media play an important role in consumer purchasing decisions. Finally, such medium characteristics as compatibility, information, web quality and security/privacy are some of the critical components that affect online purchasing intention.
Identified Relationships
Fifty-one (n=51) different relationships found between these variables were supported by multiple sources of the empirical evidence in the category of consumer characteristics and many were supported by a single source of the empirical evidence. Sixteen (n=16) relationships found between variables belonging to the retailer characteristics were supported by multiple sources of the empirical evidence. Moreover, eleven (n=11) relationships between variables of medium characteristics were supported by multiple sources of the empirical evidence and by a single source of the empirical evidence. Four (n=4) relationships involving variables under macro environmental characteristics are supported by multiple sources of the empirical evidence and by a single source of the empirical evidence, as per the thematic review.
The Process of Study 1
Each cluster was taken separately in the initial stage. The most frequently used variable in the cluster was taken, and the relationship involving that variable and purchase intention was considered at the very beginning. Similarly, the frequency priority of the variables used was utilized to develop the model.
Fifteen (n=15) variables were used and seven (n=7) variables were removed from the initial development from twenty-two (n=22) customer characteristics. Perceived price (Delafrooz et al., 2011 and Kim et al., 2012) does not relate to purchasing intention. Satisfaction (Sharma & Lijuan, 2015) does not have a relationship with purchasing intention, but it has a relationship with (He & Bai, 2011) repurchase intention. Similarly, the researcher could not find any relationship between involvement, commitment and loyalty, with online purchasing intention. The variable knowledge and experience also does not have a relationship with online purchasing, however, it was found to be a moderator in the model development stage. The variable perceived technology represents both perceived usefulness and perceived ease of use, and stood for a combination of PU and PEAU (Ling et al., 2011). Hence, perceived technology was removed from the model development process.
Among ten (n=10) retailer characteristics, seven (n=7) were involved with the model development and three (n=3) variables were removed from the development. The price was removed, since it is not a significant variable (Delafrooz et al., 2011 and Kim et al., 2012). After-sales service was also removed, since it does not have a relationship with purchase intention. Although product has a significant relationship with purchase intention, it worked better as a moderator rather than in a direct relationship. Hence, the remaining seven (n=7) variables were used to develop the model.
Four (n=4) variables from medium characteristics, which had a relationship with purchase intention, were used to develop the model. Similarly, four (n=4) variables from macro environmental characteristics, which had a relationship with purchase intention, were used to develop the model.
The Output of Study 1 – Initial Model Alfa (Mα)
The four partial models described above were integrated to develop the initial model Mα.
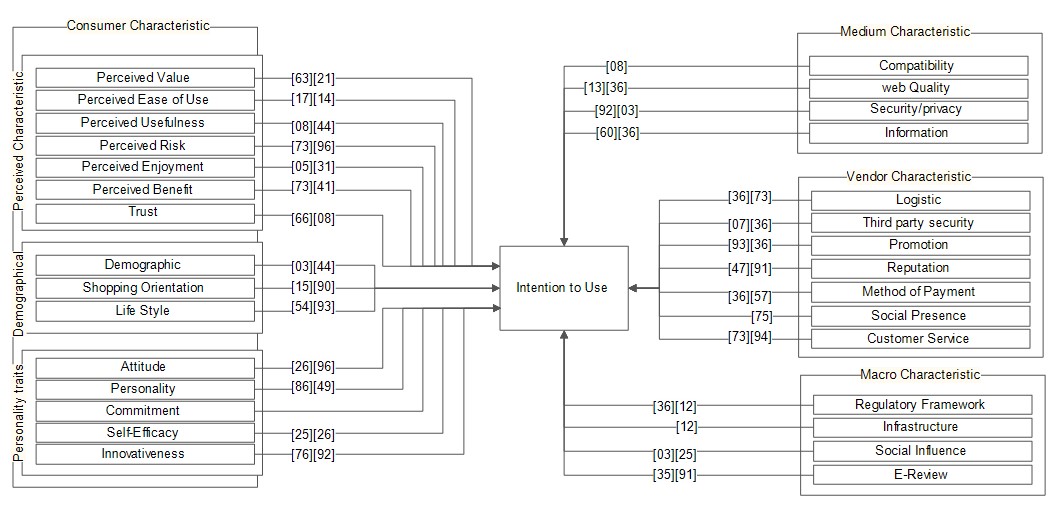
Figure 2: Initial Research Model Mα
Source: Developed by the Researcher
Note: Citation Reference for Relationship – Appendix 1
Study 2 – Evaluation of the Main Extant Theoretical Models
This section evaluates both reviews of Technology acceptance models and E-service quality models. Technology acceptance models were critically reviewed in four ways. They were compared with the proposed model in this study. Through a closer examination of the existing literature, four major domain areas, namely, Consumer characteristic (Chan et al., 2003; Chang et al., 2005 and Kandambi & Wijenayake, 2017a), Retailer characteristics (Chan et al., 2003; Chang et al., 2005 and Kandambi & Wijenayake, 2017b), Web characteristics (Chan et al., 2003 and Chang et al., 2005) and Social characteristics (Chan et al., 2003 and Chang et al., 2005) can be identified in the concept of e-commerce. Extant Technology Acceptance theories do not consider retailer characteristics, website characteristics and some customer characteristics.
Review of Technology Acceptance Models
Table 2: Variables Grid of Technology Acceptance Theories vs. Proposed Model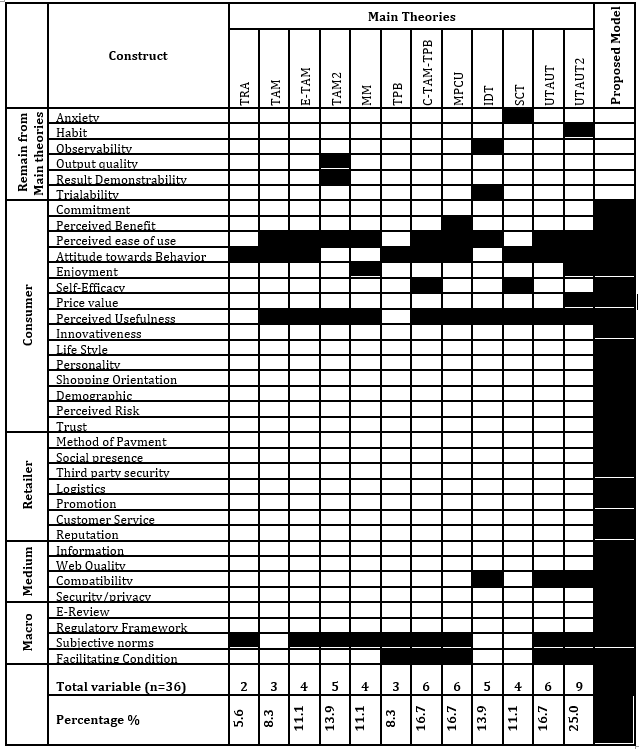
Source: Developed by the Researcher
The above thematic evaluation compared the concept and its constructs belonging to each model. All constructs from each prior technology acceptance model were taken into consideration. Both constructs emerging from the thematic review and those included in prior models were listed, and identical constructs were merged and renamed to remove redundancy. Hence, perceived usefulness (TAM, and combined TAM-TPB), extrinsic motivation (MM), job fit (MPCU), outcome expectation (SCT) and relative advantage (IDT) were merged because they measured the same construct. Hence, perceived usefulness was used to represent all the above constructs because of the similarities between them. Attitude towards behavior, affect, affect towards behavior and instructional motivation were all constructs used to measure the attitude of an individual, according to their working definitions. Hence, attitude was used to represent all these constructs.
Complexity (MPCU) and ease of use (IDT) are similar constructs that measure perceived ease of use (TAM). Hence, perceived ease of use was retained to represent these constructs. Enjoyment, perceived enjoyment and hedonic motivation are all similar concepts according to their working definitions. Hence, the term perceived enjoyment was retained for further work. Finally, psychological attachment (E-TAM), subjective norms (TRA, TAM2, TPB/DTPB, and combined TAM-TPB), social factors (MPCU) and image (IDT) are all determinants of social influence. Hence, social influence was used as the main variable for the further model development.
All major technology acceptance theories discuss only fourteen (n=14) constructs. However, from the themetic evaluation conducted in Chapter 2, thirty (n=30) constructs have been identified as influencing online purchasing. Eight (n=8) out of the fourteen (n=14) constructs from previous theories were similar to some of the thirty variables identified by the thematic evaluation of this study. Moreover, those variables were very commonly used in the research context.
Hence, the total number of constructs identified by both thematic evaluation and prior Technology Acceptance theories are thirty-six (n=36). According to the table, the minimum number of constructs from the available range of constructs was used by TRA, which was two constructs (n=2, p=5.6%). That was the beginning of Technology Acceptance theories. Then TAM and TPB used three constructs (n=3, p=8.3%) per model. Four constructs (n= 4, p=11.1%) were employed by the E-TAM, MM and SCT theories in their models. The TAM2 and IDT models used five constructs (n=5, p=13.9%) to measure technology acceptance. Another three models, C-TAM-TPB, MPCU and UTAUT employed six constructs (n= 6, p=16.7%) in their studies. UTAUT was crafted as an essence of previous technology acceptance theories. It evaluated and integrated all aspects of extant technology acceptance theories. Hence, UTAUT uses the maximum number of constructs associated with its model. UTAUT2 has nine constructs (n=9) employed in its model, and the percentage of variance explained is calculated as twenty-five percent (p=25.0%). It must be emphasized here that the model proposed by this study introduces thirty (n=30) different variables in the context of consumer-based electronic commerce, which explains eighty-three-point three percent of the total variance (p=83.3%).
Review of E-Service Quality Model
E-service quality plays a significant role in the context of e-commerce (Santos, 2003). E-service quality directly influences attractiveness, hit rate, customer retention, stickiness and positive word-of-mouth, and can enhance online purchasing considerably. Hence, the result of this evaluation takes e-service quality model review into consideration.
Table 3: Review of E-Service Quality Model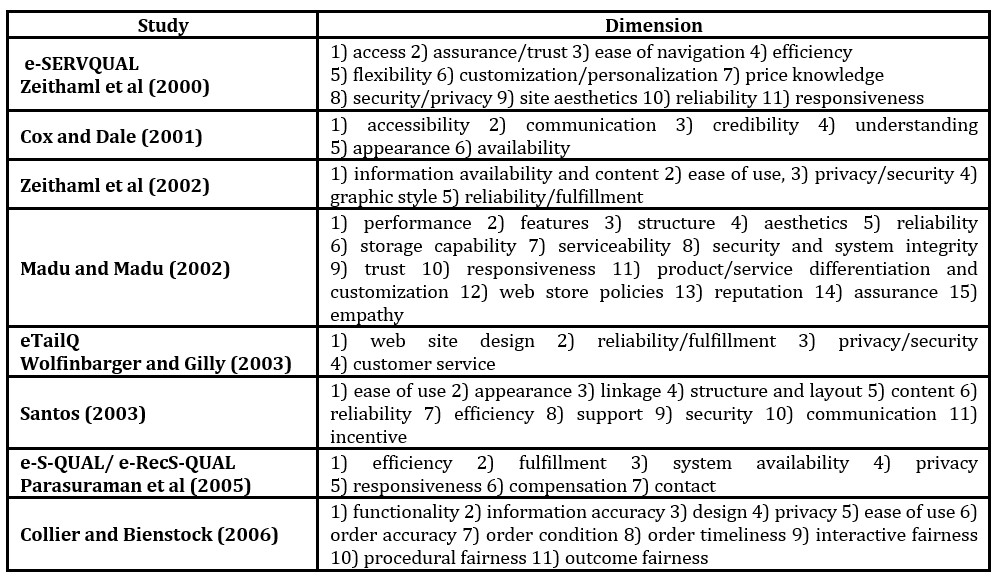
Source: Developed by the Researcher
E-service quality models mainly deal with the dimensions of customer service, privacy and security, website quality and after-sales service. Even the construct E-S-QUAL e-RecS-QUAL, in the model of Parasuraman et al. (2005), can be regrouped into the above four main categories. For example, efficiency and system availability are grouped into website quality, while responsiveness, compensation and contact are grouped into after-sales service. Also, privacy is grouped with privacy and security, and fulfillment is grouped into customer service. Most of the constructs from e-service models can be regrouped based on their working definitions. Web site design, appearance, structure and layout, graphic style, aesthetics, appearance and site aesthetics from all e-service models define slightly different or similar aspects of web design and interface design.
Information accuracy, content and information availability are similar dimensions, which represent or are formulated by the construct ‘information’. Similarly, system availability, accessibility, availability and access represent the construct termed ‘system availability’. Though functionality, linkage, support, storage capability, web store policies and features, have different names, they all represent the functionality of the web site. Further, efficiency and performance of the website represent the speed of the website. Assurance/trust, contact, responsiveness, empathy, reliability, customization/personalization and fulfillment, are all dimensions of customer service, based on their working definitions. Similarly, compensation, outcome fairness, customer service, responsiveness, serviceability, interactive fairness and procedural fairness, all represent the dimension of after-sales service.
Table 4: Variable Grid of E-service Quality Theories vs. Proposed Model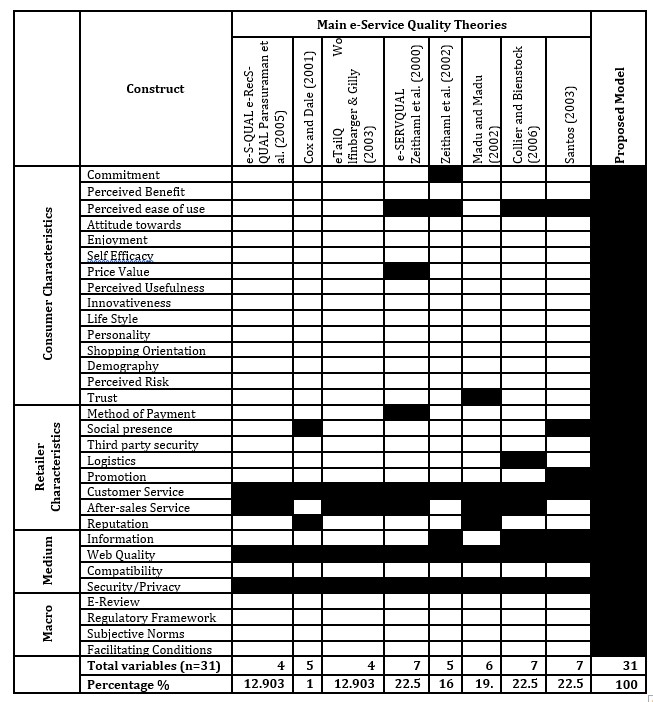
Source: Developed by the Researcher
The evaluation grid shows four different variables clusters, where all e-service models were highlighted. Privacy and security, web quality, information, customer service, and after-sales service can be identified as separate categories, which are used repeatedly in the e-service models. Furthermore, trust, method of payment, social presence, logistics and reputation can be identified as constructs separate from those of the grid. Based on this result, the researcher formulated four main concepts; 1. Transaction safety, consisting of privacy and security, 2. Web quality, 3. E-commerce service, consisting of customer service and after-sales service and 4. All other constructs grouped under vendor quality.
Secondary Model Development: Model Beta (Mβ)
The initial model alfa (Mα) was derived from the empirical evidence found in the literature survey, and it was used to build the secondary model, model beta (Mβ). The result of the evaluation of the Technology Acceptance models and the result of the evaluation of e-Service models are the foundation for developing the secondary model, beta (Mβ). All technology acceptance theories are accepted in the context of the organization. However, e-commerce engages with both technology acceptance and e-service quality, as per the technology acceptance model review. Hence, UTAUT by Venkatesh et al. (2005) was used to measure technology acceptance, and models E-S-QUAL, E-RecS-QUAL by Parasuraman et al. (2005) and eTailQ by Wolfinbarger and Gilly (2003) were used with modification in the context of e-commerce. Personal attributes, advantage expectancy, effort expectancy, facilitating conditions and social influence constructs were adopted from UTAUT by Venkatesh et al. (2005) with an adjustment to the context of e-commerce. Similarly, web quality, transaction safety, vendor quality and customer service constructs were adopted from E-S-QUAL, E-RecS-QUAL by Parasuraman et al. (2005) and eTailQ by Wolfinbarger and Gilly (2003), with modifications.
Modified Model Mβ
Output of study 2 with second order level constructs.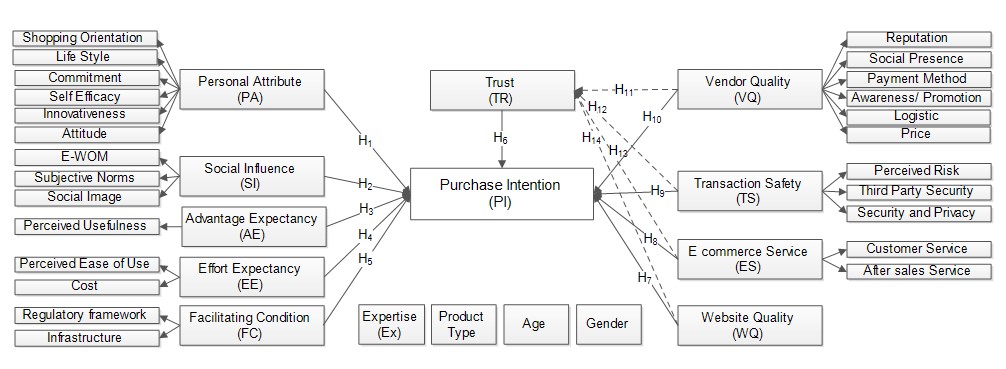
Figure 3: Second Research Model Mβ – Second Order
Source: Developed by the Researcher
Output of study 2 with first order level constructs.
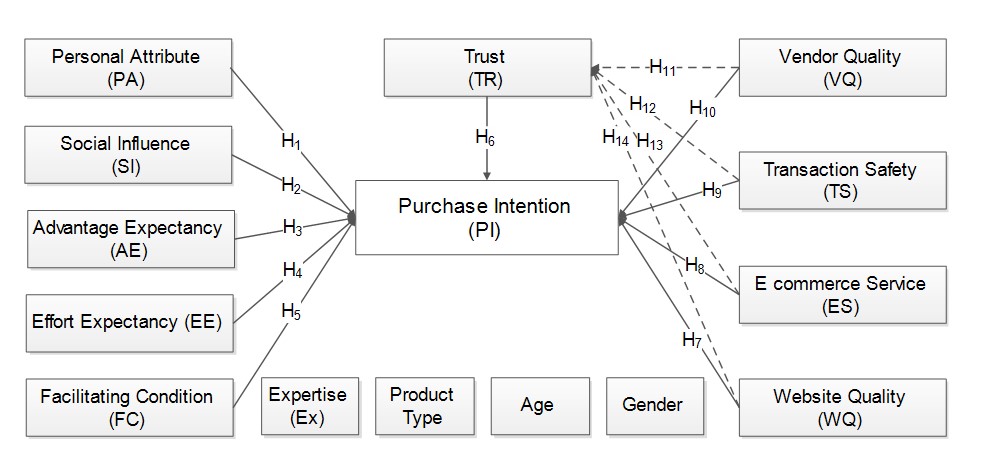
Figure 4: Second Research Model Mβ – First Order
Source: Developed by the Researcher
Study 3 – Model Verification by the Delphi Method
The Delphi technique is a structured brainstorming session with a series of sequential questionnaires with ‘rounds’ and a controlled feedback that seeks to gain the most reliable opinions of a group of experts (Powell, 2003). Hence, this technique is suitable for validating the findings and will contribute to the credibility of the research as well (Powell, 2003). The researcher was not involved in the problem-solving process, but worked as a facilitator. The researcher invited five members from three state universities and one private university. A research assistant was appointed as a coordinator to report the feedback of the panel members.
Delphi Process
Introduction and explanation: The researcher, as a facilitator, welcomed the participants and explained to them the purpose and the procedures of the meeting. The research gap, the objective of the research and the research questions were provided in a document form for reference. Silent generation of ideas (10 minutes): The Facilitator provided each participant with a sheet of paper with the question to be addressed, and asked them to write down all the ideas that came to their minds when considering the question without discussing. Round 1 (15–30 minutes): The first-round questionnaire is usually unstructured and seeks an open response (Powell, 2003). The question arose about factors, their grouping and the relationships between factors to confirm the allocation of marks in the Likert scale. That round helped to identify the issues in the constructs of the model when panel members allocated marks to the Likert scale which ranged from strongly disagree to strongly agree. Panel members had to decide whether to retain, to remove, to add elsewhere or to merge the given constructs. Round 2 (15–30 minutes): The second and subsequent rounds included more specific questions, with questionnaires seeking a quantification of earlier findings. In the second round, each delphi participant received another (second) questionnaire and was asked to review the items summarized by the experts, based on the information provided in the first round. Thereafter, their answers, including all modifications, were collected and the issues were summarized into one sheet, and the copies of that sheet were distributed to all the members for debate. The members were instructed to reproduce the model including the factors that were problematic. Again, there was a mark allocation for the model to extract further issues. As a result of round two, areas of disagreement and agreement were identified. The round did not end until all members of the panel reached an agreement. Round 3 (15–30 minutes): In the third round, each delphi panelist received a questionnaire that included the items and ratings summarized by the experts in the previous round and was asked to revise his judgment. This round gave delphi panelists an opportunity to make further clarifications of both the information and their judgments of the relative importance of the items. In this round, major modifications were not allowed. This round provided a final opportunity for panelists to revise their judgments. The round should end in consensus. Group Discussion (30–45 minutes): Participants were invited to verbalize their explanations or further discuss any of the ideas that their colleagues have brought up, and which might have not been clear to them. The group suggested some new items for discussion and combined items into categories, but no ideas were eliminated.
Analysis of the Rounds
Results of Round 1 (Appendix 2, Table 1): Based on the mark allocation made by each member of the panel for each construct, the mean value was calculated. Each construct that had a mean value below and equal to three (m=3.0) was identified as problematic. Hence, from the first round of the Delphi study, innovativeness, lifestyle, shopping orientation, cost, social presence, price, payment method and logistics were identified as constructs that needed resolution. After the discussion in the second round, it was decided to remove lifestyle, shopping orientation and product type from being designated as moderators. In the second round, after the debate, based on the first-round results, an outcome and unanimous mark allocation were generated.
Results of Round 2 (Appendix 2, Table 1): Based on the mark allocation of each member of the panel for each construct, the mean value was calculated. Each construct that had a mean value below or equal to three (mean=3.00) was identified as problematic. Hence, in the second round of the Delphi study, social presence, payment method and logistics were identified as constructs to be resolved. After the discussion in the second round, it was decided to resend the social presence, payment method and logistics constructs to vendor quality. The third round, after the debate based on the second round results, generated a consensual outcome and mark allocation.
Results of Round 3 (Appendix 2, Table 1): The result indicates that no mean value scored below 3.0. Hence, the delphi panel agreed to the model with the above mentioned modifications.
Summary of the Discussion
Based on the mark allocation, innovativeness, lifestyle, shopping orientation, cost, social presence, price, payment method and logistics were identified as constructs to be resolved in the first round of the Delphi study. After the discussions in the second round, it was decided to stop lifestyle, shopping orientation and product type from being designated as moderators. Then, based on the mark allocation of each member of the panel, social presence, payment method and logistics were identified as constructs to be resolved in the second round of the Delphi study. After the discussion in the second round, it was decided to resend the social presence, payment method and logistics constructs to vendor quality. In the third round, the results indicated that no mean value scored below 3.0. Hence, the Delphi panel agreed to the model with the modifications made in round 2, and this modified model was termed model gamma (Mσ.)
Model Mσ
Output of study 3 with second order level constructs.
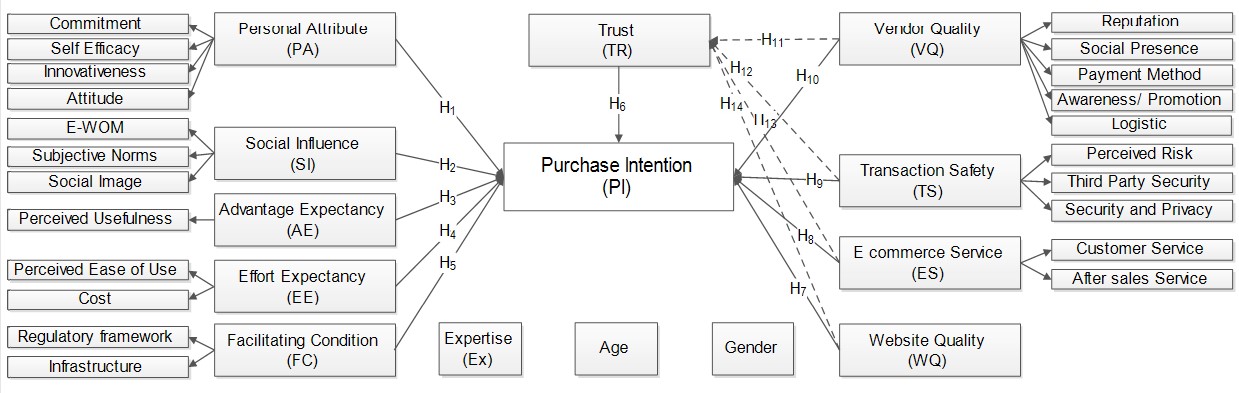
Figure 5: Second Research Model Mσ– Second Order
Source: Developed by the Researcher
Output of study 3 with first order level constructs.
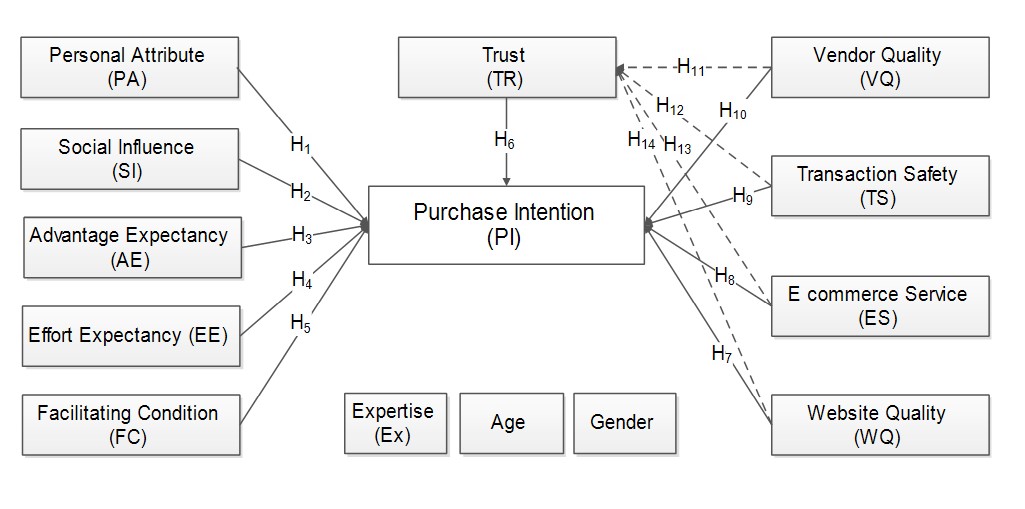
Figure 6: Second Research Model Mσ– First Order
Source: Developed by the Researcher
Study 4 – Nominal Group Technique Involving Industrial Experts
This study was done to reconfirm the output model of the Delphi study using the opinions of industrial experts. Ten industrial experts from the leading online shopping business founders and CEOs were invited for the study. The output model (Mσ) of the Delphi technique in study 3 was tested in this study.
Process
Introduction and Explanation: The researcher explained the purpose and procedures of the meeting to each industrial expert. Then, he asked each expert an open-ended question and recorded it on a voice recorder. That was supposed to help the expert change his focus from his/her business to this study. Then, he/she was allocated 15-minutes to generate ideas related to the question in his/her mind. Then, he/she was provided with the model, all constructs and the associations between the constructs. Then, the facilitator provided them with a sheet of paper with the question to be addressed and asked the experts to write down all the ideas that came to their minds when considering the question. Based on that, each expert was requested to fill in a structured questionnaire to rank the appropriateness of the model according to his/her judgment. Every modification requested by an expert needed validation in writing. The researcher collected the opinions of every industrial expert in the panel separately for the evaluation.
The mean value of eight opinions on the constructs of the model was above three. Hence, no issues were found in the model (Mσ), and it was accepted. There was no modification required to the model generated from study 3 (Mσ), since study 4 generated a model (Mμ) without modifications.
Final Conceptual Framework
Output of study 4 with second order level constructs
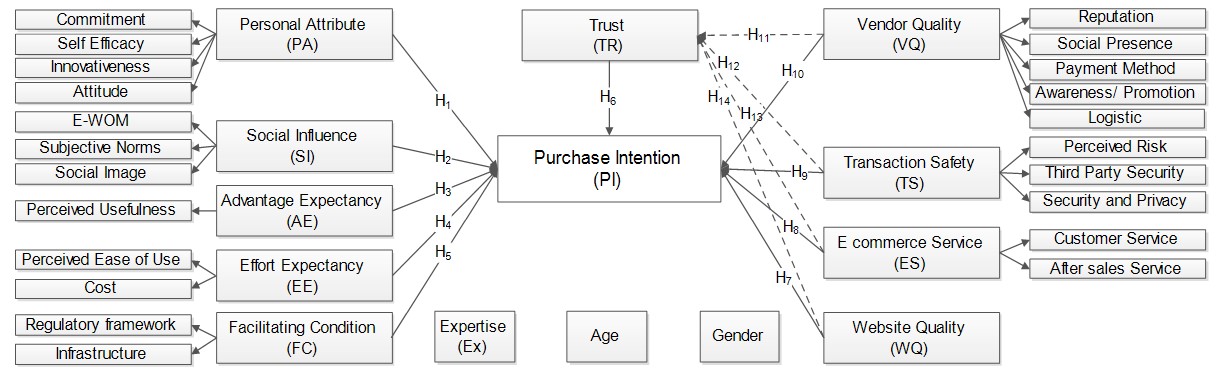
Figure 7: Final Conceptual Framework – Second Order
Source: Developed by the Researcher
Output of study 4 with first order level constructs
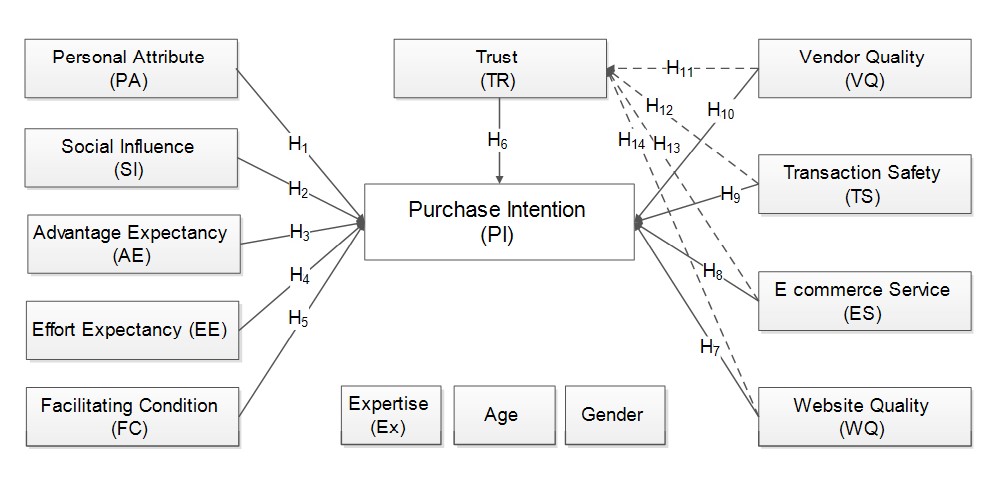
Figure 8: Final Conceptual Framework – First Order
Source: Developed by the Researcher
Table 5: Constructs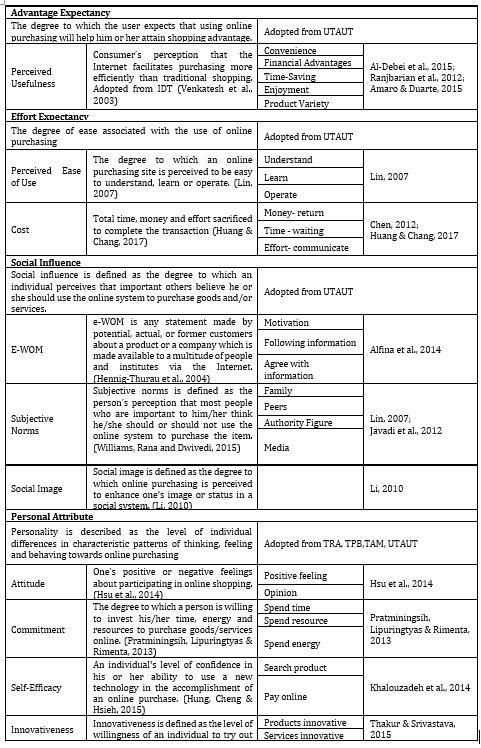
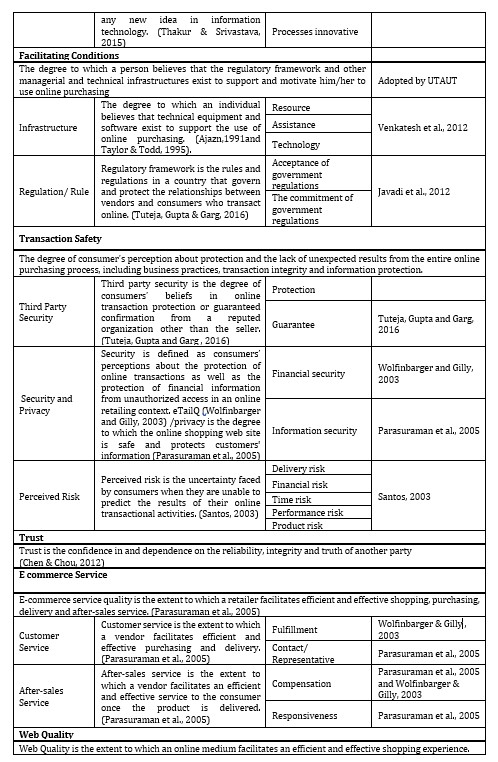
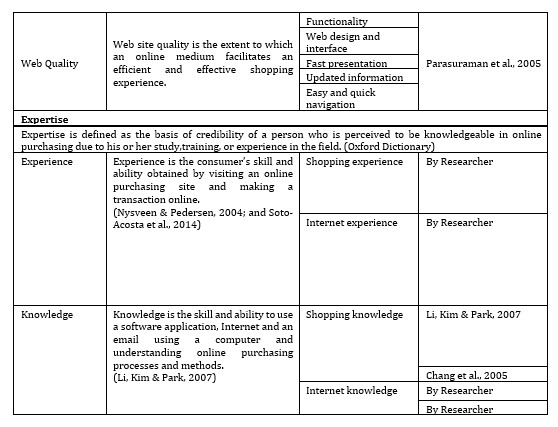
Source: Developed by the Researcher
Purchase Intention
Zhu et al. (2011) defined online purchasing intention as a consumer’s willingness to purchase online. Furthermore, the intention to buy products online is defined by some other researchers as the likelihood that a consumer plans to buy online in the near future. (Chen, 2012). Online purchase intention is also defined as a customer’s willingness and intention to purchase products via the Internet (Meskaran, Ismail & Shanmugam, 2013). Wu et al. (2013) define online purchase intention as the probability and willingness to buy online. Taking all these prior definitions into account, this study defines online purchasing intention as a consumer’s desire or willingness to purchase online in the future.
Discussion
A conceptual framework development study was conducted specifically to find a method to measure online purchasing intention, since there is no comprehensive theory to explain online purchasing intention in the context of e-commerce. In order to solve this problem, the following steps were carried out. Leading peer-reviewed conferences and indexed journals published between the years 2010 and 2015 were used to evaluate the frameworks thematically. The identified factors were grouped as consumer, retailer, medium and external environmental characteristics, and these were used to build the initial model (Mα).
Technology acceptance models and E-service quality models were critically reviewed to identify a major component in the context of e-commerce in study two. Results from study one and study two were integrated, and constructs were regenerated to build the second model beta (Mβ). The results of the delphi method confirm that the second model beta (Mβ), with minor modifications, was used to generate the third model gamma (Mσ). Further, model gamma (Mσ) was tested utilizing the nominal group technique with industrial experts, and those results confirm model gamma, without modifications, and this was then callled model mu (Mμ). The constructs of the model, Personal Attributes, Social Influence, Advantage Expectancy, Effort expectancy, Facilitating Conditions, Vendor Quality, Transaction Safety, E-commerce Services, Web Quality and Trust had a direct association with online purchasing intention. Furthermore, Vendor Quality, Transaction Safety, E-commerce Services and Web Quality had an indirect association with online purchasing intention through Trust. Age, Gender and Expertise were the moderators of the relationships between the constructs of the model and online purchasing intention.
Conclusion
This holistic and integrated conceptual framework addresses the entire e-commerce process. Not only the technology acceptance but also the consumer aspect and environmental aspect considered in the model. This comprehensive model is ready to measure online purchasing intention. Hence, this model should be empirically tested before commercialized.
(adsbygoogle = window.adsbygoogle || []).push({});
References
- Abu-Shamaa, R. and Abu-Shanab, E. (2015) ‘Factors Influencing the Intention to Buy From Online Stores: An Empirical Study in Jordan’, in 2015 IEEE 8th GCC Conference and Exhibition, GCCCE 2015. Muscat, pp. 1–4.
- Adnan, H. (2014) ‘An Analysis of the Factors Affecting Online Purchasing Behavior of Pakistani Consumers’, International Journal of Marketing Studies, 6(5), pp. 133–149.
- Ahmad, N., Omar, A. and Ramayah, T. (2010) ‘Consumer lifestyles and online shopping continuance intention’, Business Strategy Series, 11(4), pp. 227–243.
- Ajzen, I. (1991) ‘The Theory of Planned Behavior’, Organizational Behavior and Human Decision Processes, 50, pp. 179–211.
- Alam, S. S. and Yasin, N. M. (2010) ‘What factors influence online brand trust: Evidence from online tickets buyers in Malaysia’, Journal of Theoretical and Applied Electronic Commerce Research, 5(3), pp. 78–89.
- Alfina, I., Ero, J., Hidayanto, A. N. and Shihab, M. R. (2014) ‘The impact of cognitive trust and e-wom on purchase intention in C2C e-commerce site’, Journal of Computer Science, 10(12), pp. 2518–2524.
- Ariff, M. S. M., Yan, N. S., Zakuan, N., Bahari, A. Z. and Jusoh, A. (2013) ‘Web-based factors affecting online purchasing behaviour’, in IOP Conference Series: Materials Science and Engineering.
- Bandura, A. (1986). Social foundations of thought and action: A social cognitive theory. Englewood Cliffs, NJ: Prentice-Hall.
- Cha, J. (2011) ‘Exploring The Internet As A Unique Shopping Channel To Sell Both Real And Virtual Items: A Comparison Of Factors Affecting Purchase Intention And Consumer Characteristics’, Journal of Electronic Commerce Research, 12(2), pp. 115–132.
- Chan, G., Cheung, C., Kwong, T., Limayem, M. and Zhu, L. (2003) ‘Online Consumer Behavior: A Review and Agenda for Future Research’, in BLED 2003 Proceedings, pp. 194–218.
- Chang, M. K., Cheung, W. and Lai, V. S. (2005) ‘Literature derived reference models for the adoption of online shopping’, Information and Management, 42, pp. 543–559.
- Chen, N. H. and Hung, Y. W. (2015) ‘Online Shopping Orientation And Purchase Behavior For High-Touch Products’, International Journal of Electronic Commerce Studies, 6(2), pp. 187–202
- Cho, Y. C. and Sagynov, E. (2015) ‘Exploring Factors That Affect Usefulness, Ease Of Use, Trust, And Purchase Intention In The Online Environment’, International Journal of Management & Information Systems (IJMIS), 19(1), pp. 21–36.
- Collier, J. E. and Bienstock, C. C. (2006) ‘Measuring service quality in E-retailing’, Journal of Service Research, 8, pp. 260–275.
- Cox, J. and Dale, B. G. (2001) ‘Service quality and e-commerce: an exploratory analysis. Managing Service Quality’, Managing Service Quality: An International Journal, 11(2), pp. 121–131.
- Cummins, S., Peltier, J. W., Schibrowsky, J. A. and Nill, A. (2014) ‘Consumer behavior in the online context’, Journal of Research in Interactive Marketing, 8(3), pp. 169–202.
- Davis, F. D. (1989) ‘Perceived Usefulness, Perceived Ease of Use, and User Acceptance of Information Technology’, MIS Quarterly, 13(3), p. 319.
- Davis, F. D., Bagozzi, R. P. and Warshaw, P. R. (1989) ‘User Acceptance of Computer Technology: A Comparison of Two Theoretical Models’, Management Science, 35(8), pp. 982–1003.
- Davis, F., Warshaw, P. and Bagozzi, R. (1992) ‘Extrinsic and Intrinsic Motivation to Use Computers in the Workplace1’, Journal of Applied Social Psychology, 22(14), pp. 1111–1132.
- Delafrooz, N., Paim, L. and Khatibi, A. (2011) ‘Understanding Consumer’s Internet Purchase Intention in Malaysia’, African Journal of Business Management, 5(3), pp. 2837–2846.
- G.P.H. Kandambi and W.M. J. I Wijayanayake (2017b),” Comprehensive Evaluation on the Influence of Retailer’s Characteristics on Online Purchasing Intention – a Thematic Review “, Journal of Internet and e-Business Studies, Vol. 2017 (2017),
- G.P.H. Kandambi and W.M. Janaka I Wijayanayake (2017a), ” Comprehensive Factor Evaluation on Impact of Consumer’s Characteristic on Online Purchasing Intention in E- Commerce: A Literature Review”, Journal of Internet and e-Business Studies, Vol. 2017 (2017),
- He, J. and Bai, Y. (2011) ‘Assessing the Relationship among Service Quality, Customer Satisfaction and Behavioral Intentions in Online Shopping Environments’, in 2011 Fourth International Conference on Business Intelligence and Financial Engineering, pp. 155–159. doi: 10.1109/BIFE.2011.26.
- Hernandez, B., Jimenez, J. and Martin, M. J. (2011) ‘Age, gender and income: Do they really moderate online shopping behaviour?’, Online Information Review, 35(1), pp. 113–133.
- Hong, I. B. (2015) ‘Understanding the consumer’s online merchant selection process: The roles of product involvement, perceived risk, and trust expectation’, International Journal of Information Management. Elsevier Ltd, 35(3), pp. 322–336.
- Jiang, L. (Alice), Yang, Z. and Jun, M. (2013) ‘Measuring consumer perceptions of online shopping convenience’, Journal of Service Management, 24(2), pp. 191–214.
- Kim, H. W., Xu, Y. and Gupta, S. (2012) ‘Which is more important in Internet shopping, perceived price or trust?’, Electronic Commerce Research and Applications. Elsevier B.V., 11(3), pp. 241–252.
- Kim, J. and Forsythe, S. (2010) ‘Factors affecting adoption of product virtualization technology for online consumer electronics shopping’, International Journal of Retail & Distribution Management, 38(3), pp. 190–204.
- Ling, K. C., Chai, L. T. and Piew, T. H. (2010) ‘The Effects of Shopping Orientations, Online Trust and Prior Online Purchase Experience toward Customers’ Online Purchase Intention’, International Business Research, 3(3), pp. 63–76.
- Madu, C. N. and Assumpta A. Madu (2002) ‘Dimensions of e-quality’, International Journal of Quality & Reliability Management, 19(3), pp. 246–258.
- Meskaran, F., Ismail, Z. and Shanmugam, B. (2013) ‘Online Purchase Intention: Effects of Trust and Security Perception’, Australian Journal of Basic and Applied Sciences, 7(6), pp. 307–315.
- Nazir, S., Tayyab, A., Sajid, A., Ur Rashid, H. and Javed, I. (2012) ‘How Online Shopping Is Affecting Consumers Buying Behavior in Pakistan?’, IJCSI International Journal of Computer Science, 9(3), pp. 486–495.
- Ozguven, N. (2011) ‘Analysis of the relationship between perceived security and customer trust and loyalty in online shopping’, Chinese Business Review, 10(11), pp. 990–997.
- Parasuraman, A., Zeithaml, V. A. and Malhotra, A. (2005) ‘E-S-QUAL a multiple-item scale for assessing electronic service quality’, Journal of Service Research, 7, pp. 1–21.
- Powell, C. (2003) ‘The Delphi technique : myths and realities’, Journal of Advanced Nursing, 41(4), pp. 376–382.
- Rogers, E. M. (1995) Diffuison of Innovations, Macmillan Publishing Co., Inc.
- Sahney, S., Ghosh, K. and Shrivastava, A. (2013) ‘“Buyer’s motivation” for online buying: An empirical case of railway e-ticketing in Indian context’, Journal of Asia Business Studies, 8(1), pp. 43–64.
- Sahney, S., Ghosh, K. and Shrivastava, A. (2013) ‘Conceptualizing consumer “trust” in online buying behaviour: An empirical inquiry and model development in Indian context’, Journal of Asia Business Studies, 7(3), pp. 278–298.
- Santos, J. (2003) ‘E-service quality: A model of virtual service quality dimensions’, Managing Service Quality: An International Journal, 13(3), pp. 233–246.
- Sharma, G. and Lijuan, W. (2015) ‘The effects of online service quality of e-commerce Websites on user satisfaction’, The Electronic Library, 33(3), pp. 468–485.
- Taylor, S. and Todd, P. (1995) ‘Assessing IT Usage: The Role of Prior Experience’, MIS Quarterly, 19(4), pp. 561–570.
- Thompson, R. L., Higgins, C. A. and Howell, J. M. (1991) ‘Personal Computing: Toward a Conceptual Model of Utilization’, MIS quarterly, 15(1), p. 124–143.
- Venkatesh, V. and Davis, F. D. (2000) ‘A Theoretical Extension of the Technology Acceptance Model: Four Longitudinal Field Studies’, Management Science, 46(2), pp. 186–204.
- Venkatesh, V., Morris, M. G., Davis, G. B. and Davis, F. D. (2003) ‘User Acceptance of Information Technology: Toward a Unified View’, MIS Quarterly, 27(3), pp. 425–478.
- Venkatesh, V., Thong, J. Y. L. and Xu, X. (2012) ‘Consumer Acceptance And Use Of Information Technology: Extending The Unified Theory Of Acceptance And Use Of Technology Viswanath’, Forthcoming in MIS Quarterly, 36(1), pp. 157–178.
- Wolfinbarger, M. and Gilly, M. C. (2003) ‘eTailQ: Dimensionalizing, measuring and predicting etail quality’, Journal of Retailing, 79(3), pp. 183–198.
- Zeithaml, V. A., Parasuraman, A. and Malhotra, A. (2002) ‘Service quality delivery through web sites: A critical review of extant knowledge’, Journal of the Academy of Marketing Science, 30(4), pp. 362–375.
Appendix 1
Citation Reference for Relationship
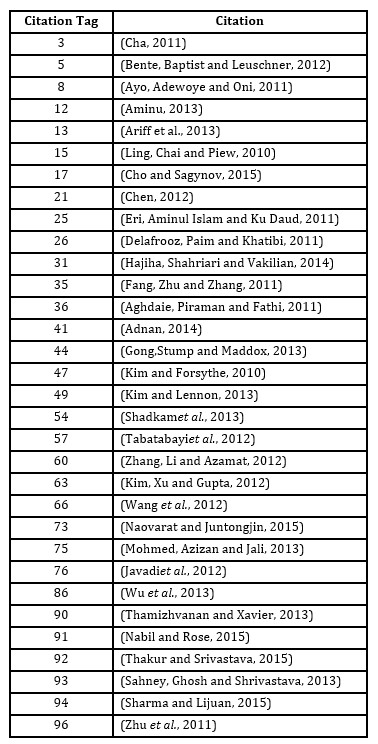
Appendix 2
Results of Round 2
Table 1: Mark Allocation for the Constructs of the Model – Round 1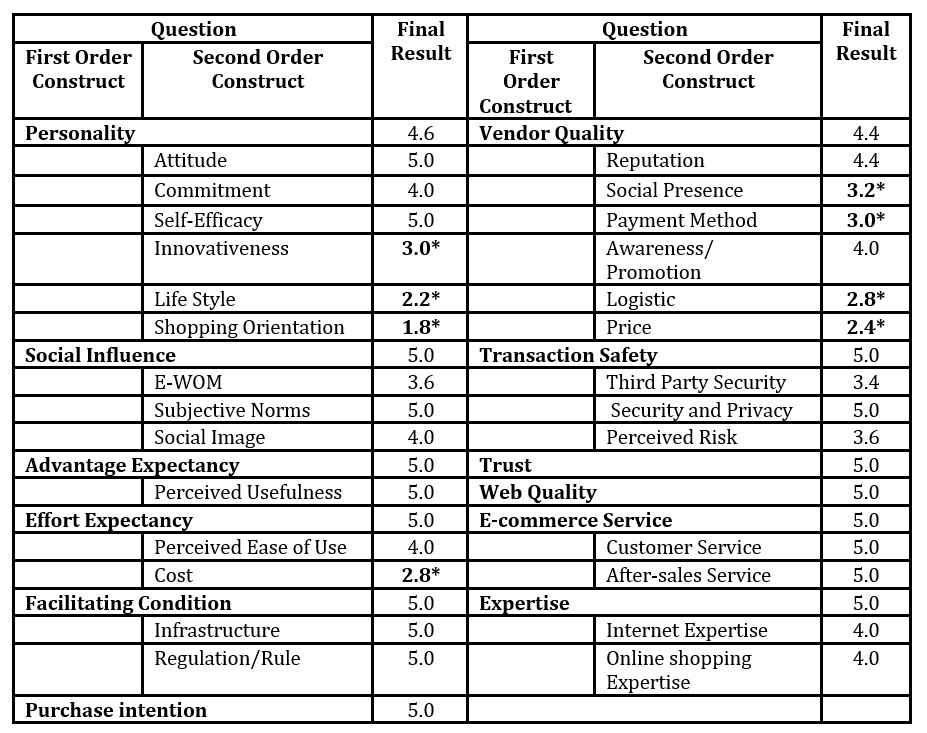
Source: Developed by the Researcher
Table 2: Mark Allocation for the Moderators of the Model –Round 1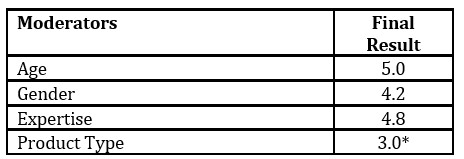
Source: Developed by the Researcher
Results of Round 2
Table 3: Agreement on Modifications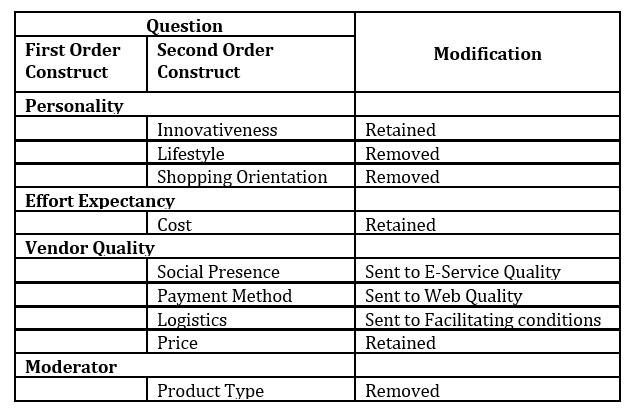
Source: Developed by the Researcher
Table 4: Mark Allocation for the Constructs of the Model – Round 2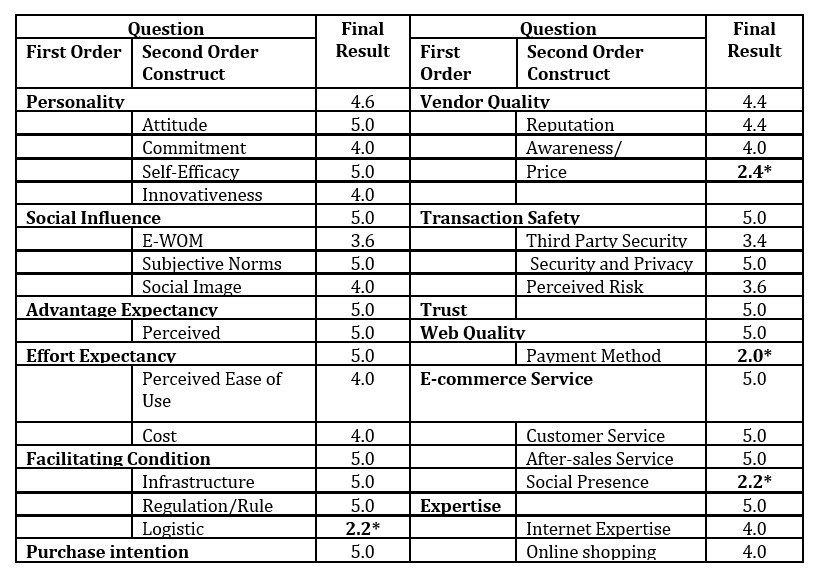
Source: Developed by the Researcher
Table 5: Mark Allocation for the Moderators of the Model – Round 2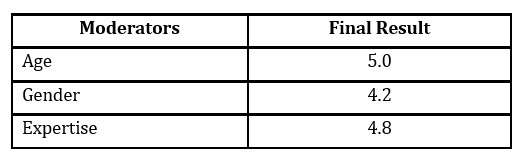
Source: Developed by the Researcher
Results of Round 3
Table 6: Agreement on Modifications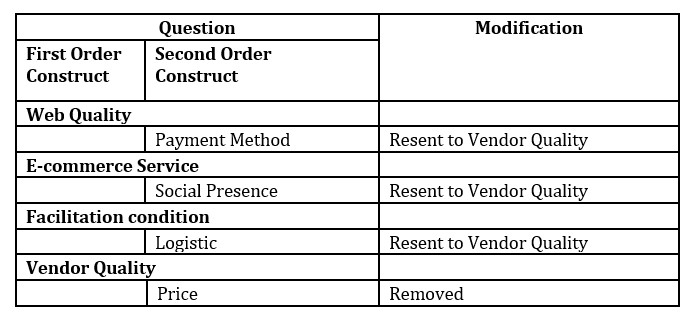
Source: Developed by the Researcher
Table 7: Mark Allocation for the Constructs of the Model –Round 3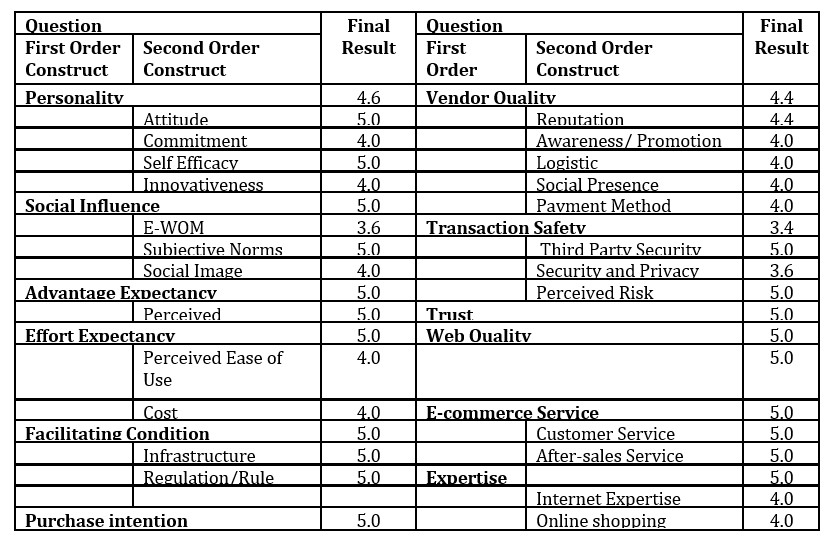
Source: Developed by the Researcher
Table 8: Mark Allocation for the Moderators of the Model –Round 3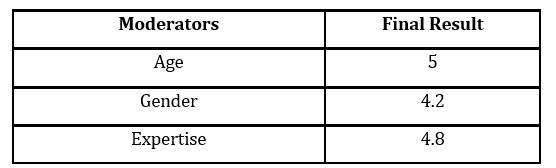
Source: Developed by the Researcher
Appendix 3
Table 9: Results Generated from NGT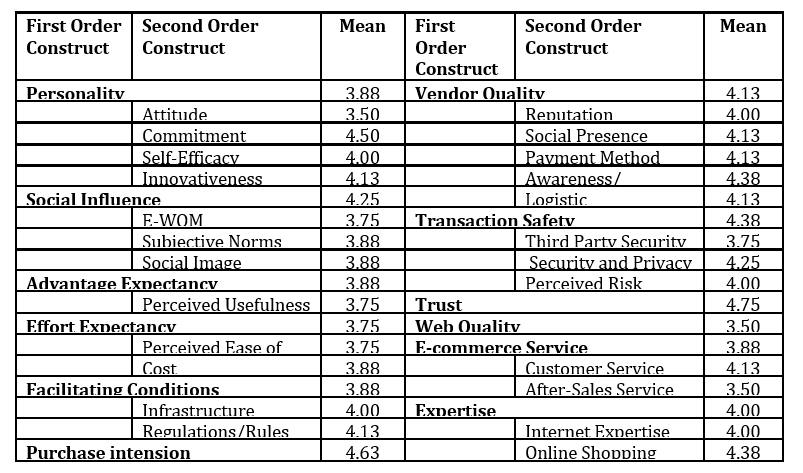
Source: Developed by the Researcher