Introduction
As social media channels make their way from personal to professional lives, and permeate into everyday corporate routines and business workflows, organizations are faced with the need to formulate and implement measurement techniques that can help them derive insights from social media interactions and evaluate the success of their own social media initiatives. Social media analytics is a nascent and emerging field poised to enable such an undertaking — allowing businesses to improve their performance management initiatives across various business functions. Whether it is to measure the effectiveness of promotional campaigns, gather insights about customer needs and preferences, discern brand perceptions, obtain feedback on product performance, or to capture data on market trends, social media analytics have the potential to provide useful information to various lines of business such as marketing and sales, customer relations, public relations and product development.
Despite these recognized benefits and the wide range of vendor offerings and technology capabilities in the social media analytics space, businesses are still struggling with adopting, implementing and institutionalizing methodologies and techniques for an effective social media analytics program (Owyang, 2011; Petrocelli, 2013). This paper addresses this issue by advancing the viewpoint that social media analytics need to be positioned as a business intelligence practice — tying its various monitoring, discovery and predictive capabilities to the tactical execution of social media initiatives and to high level strategic objectives of the organization.
In terms of organization, this paper first provides conceptual definitions of social media and social media analytics. This is followed by examples of contemporary business use-cases and metrics constituting social media analytics practices. Next, the positioning of social media analytics programs within the realm of an organization’s business intelligence practice is elaborated. The paper concludes with qualitative research findings from two recent expert panel sessions. The findings are presented in the form of five key takeaways related to the current landscape for social media analytics, as well as guidelines for firms looking at adopting various technologies and processes to enable social media analytics in their organization.
Conceptual Foundation
From Social Media to Social Media Analytics
Before discussing the scope and specifics of social media analytics, it is important to clarify what is included in our definition of social media, as there is no hard-and-fast definition of the term that everyone agrees upon (Kaplan & Haenlein, 2010), and the term has often been misused as a buzzword (Kietzmann, Hermkens, McCarthy, & Silvestre, 2011). Notwithstanding the lack of a standard definition, most academics and practitioners use the term social media to describe a variety of online channels and platforms that can facilitate collaborative creation and dissemination of information. Common descriptive features of social media include interactive, user-generated, collaborative, shared, social network and rapid information dissemination (Kaplan & Haenlein, 2010). Categories of social media defined by Sterne (2010) include the following: forums and message boards, review and opinion sites, social networks, blogging, microblogging, bookmarking, and media sharing.
Central to the social media, categories listed above are two concepts that differentiate these channels and platforms from other traditional technologies for computer mediated communication — namely, activity streams and social graphs (Hinchcliffe, Kim, & Dachis, 2012). Social media behaviors such as communicating, creating, sharing and collaborating constitute the activity streams of individuals. Furthermore, these interactions occur within the context of ties and relationships among individuals and their social network of friends, followers and fans — representing their social graph of connections.
These ideas of activity streams and social graphs are also significant in the context of social media measurement as they provide the basis for quantifying and evaluating actions and responses that spawn content and conversations on social media platforms and channels. These baseline activity metrics at a granular level can subsequently be used to develop advanced measurement models in a company’s social analytics initiatives — the subject of discussion in the next sections of this paper.
Working Definition of Social Media Analytics
Consistent with the business intelligence perspective of social media analytics adopted in this paper, we adopt the following working definition of social media analytics as proposed by Lovett (2011): “Social Analytics is the discipline that helps companies measure, assess, and explain the performance of social media initiatives in the context of specific business objectives”. This definition highlights the relevance of social media analytics to performance management and business objectives — constructs that define the very notion of business intelligence (Lonnqvist & Pirttimaki, 2006; Watson & Wixom, 2007).
At its core, social media analytics comprises the practice of defining and adopting the right metrics for measuring the success of social media initiatives in organizations. It is a measurement framework that facilitates an integrated viewpoint of an organization’s strategic objectives, its performance measures at an operational level, and various social media metrics that can be tied to those performance measures.
For purposes of this discussion, we differentiate between social media metrics and analytics by situating the latter as a higher-order construct. This viewpoint is consistent with that of other researchers and practitioners (Carlson & Kavanagh, 2011; Sterne, 2010). While metrics are primarily concerned with data, transactions and information from the past, analytics on the other hand draw upon and analyze those metrics to generate insights and inform future strategy. For example, Lovett (2011) proposes a multi-level hierarchy of social media measures ranging from counting metrics to foundational metrics, outcome metrics and business value metrics — each level building on top of the other, and together they constitute a social media analytics program. It should be emphasized though, that actionable analytics require well-designed metrics, and without the right set of descriptive measurements at an operational level, organizations won’t be able to discover patterns and gain insights for effective business strategy.
Common Use-Cases & Performance Metrics for Social Media Analytics
While specific features and capabilities of social media analytics solutions vary a great deal among vendors, most software provides support for standard use-cases that apply across a wide range of businesses.
Algorithms based on social network analysis, natural language processing, complex event processing, and data mining techniques are commonly utilized by social media analytics software to offer a range of analysis capabilities and metrics that can be used by managers for deriving critical business insights, and to facilitate effective decision-making (Melville et al., 2009; Luckham, 2011).
On the outset, many analysts agree that businesses often use social media analytics for a few common reasons. The main business drivers for social media analytics include: identifying advocates and influencers; understanding sentiment; identifying audience segments; measuring brand reputation and share of voice; and determining effectiveness of marketing messages (Lovett, 2011; Petrocelli, 2013; Stodder, 2012). More recently, there has also been an emphasis on including predictive analysis capabilities in social analytics software to allow businesses to discover patterns and anticipate impacts of current metrics, such as sentiment on future social media activities (Petrocelli, 2013; Stodder, 2012).
Table 1 below summarizes some common use-cases and the underlying questions that businesses want to answer through social media analytics, along with analysis techniques that enable these use-cases and sample metrics that may be of interest to management.
As highlighted in the Table 1, effective social media measurement comprises a combination of metrics derived through multiple analysis techniques, and multiple metrics need to be weaved together in order to extract meaning and insights that are relevant to the business. Also, the complexity of analysis techniques varies by the context of business and the scope of tactical or strategic insights desired. A holistic social media analytics program should ideally incorporate multiple use-cases that can enable monitoring, discovery and predictive capabilities to be utilized in the decision-making process.
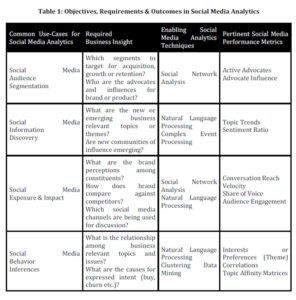
As outlined in Table 1, there are various options available to organizations for implementing and institutionalizing their social media analytics programs. What is important is for social media analytics to be approached as a discipline that transcends just technology tools and applications. It should be considered as a practice and a process that can enable businesses to use social data as a crucial input into business decisions and strategy. Towards this, social media analytics needs to be situated and integrated as part of the overall business intelligence practice in the organization. The next section provides an overview of the requirements and benefits for adopting such an orientation for social media analytics.
Social Media Analytics As a Business Intelligence Practice
For the purposes of this paper, we adopt a business intelligence (BI) perspective of social media analytics. In positioning a social media analytics program as part of an organization’s business intelligence practice, we adhere to the notion that BI can be conceptualized as both, a process and a product (Jourdan et al., 2008). As a process, BI comprises methods and procedures that organizations can use to acquire, analyze and disseminate information for business decision-making (Lonnqvist & Pirttimaki, 2006), and develop useful intelligence to help them compete and grow in their industry (Jourdan et al., 2008). The product viewpoint of BI focuses on the resulting information from analytical procedures that can allow organizations to predict the behavior of various stakeholders and industry players within their business environment (Vedder et al., 1999). Overall, the practice of BI pertains to making effective use of information technology to deliver actionable information for decision-makers (Negash, 2004; Watson & Wixom, 2007).
Core to the practice of BI is the integration between business objectives at the strategy level and key performance indicators (KPIs) at the operational level. KPIs are metrics that are central to the well-being of an organization, and can be used directly to measure or evaluate the success of achieving the business objectives, and to prescribe a course of action (Lonnqvist & Pirttimaki, 2006; Watson & Wixom, 2007).
Recent research on social media analytics has emphasized the need to adopt a BI based approach to collecting, analyzing and interpreting social media data (Heijnen et al., 2013; Murdough, 2009). In practicing social media analytics as a business intelligence initiative, it is important that every social media metric tie to a business metric or KPI (key performance indicator), which in turn should map to a strategic business objective. For example, it is often recommended that social media measurement initiatives be instigated through a high-level consideration of business objectives common to all organizations — raising revenue, lowering costs, or improving customer satisfaction (Sterne, 2010). Similarly, for social media marketing initiatives, the high-level goals are usually tied to brand awareness, lead generation and customer retention (Kelly, 2012). Etlinger et al. (2013) emphasize that business goals can often act as the North Star for businesses, hence helping them formulate and prioritize their social media measurement initiatives.
Overall, the relationship between corporate objectives, supporting business unit metrics or KPIs and social media metrics should be identifiable. Early efforts in defining and elaborating these relationships can help provide a strong foundation for a social media analytics program in the organization (Lovett, 2011; Zeng et al., 2010). Owyang et al. (2010) refer to this exercise as “creating a social media measurement playbook” that aligns an organization on the goals, objectives, expectations and actions of its social media initiatives.
Figure 1 below depicts the relationships among strategy, management and execution levels in the organization. We provide the following simple example to illustrate the configuration and relationships between the three levels shown in the figure. Towards meeting the high-level business objective of improving customer satisfaction, fostering dialog with clients may be a specific business objective for customer relationship management at the strategy level. Among other KPIs that can be used to track the effectiveness in meeting this objective at the management level, the organization may use a metric such as conversation reach (Interactive Advertising Bureau, 2009). At the execution level, such a metric can be calculated by looking at the ratio between the number of people participating in the conversation and the number of people exposed to the conversation (Owyang et al., 2010).
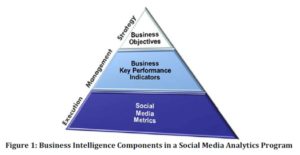
In deliberating social media analytics through a BI lens, it is recommended that in addition to simply defining operational metrics to strategic KPIs, organizations also establish benchmarks at the levels of KPIs and social media metrics. Such an orientation can spell the difference between success and failure of a social media measurement initiative (Fisher, 2009; Murdough, 2009), and it also indicates the maturity of social business intelligence practices and processes in the organization (Etlinger et al., 2013). Continuing with the example cited above, metrics such as conversation reach should ideally not just be reported as an absolute metric, but also as a trend over time, or as a comparative indicator against previous or external benchmark. This will help the organization gain a sense of whether the volume of participants in their conversations is expanding, and whether the conversations are spreading beyond their regular dialog venues.
The preceding sections in this paper have outlined the author’s perspective of social media analytics including conceptual underpinnings as well as suggested orientation for businesses. In order to expand upon some of these guidelines and offer further insights, the next sections in the paper discuss the findings and results from two expert panels that were conducted to gain an understanding of the current state and future prospects of social media analytics.
Expert Insights & Recommendations
Methodology
Qualitative data for this study was collected through two online expert panel sessions consisting of 11 participants in total (six in the first session and five in the second). The participants who were invited to assist with this research were selected as experts in the field of social media analytics by virtue of their industry experience. They were approached and recruited through online discussion forums and practice groups on social networking sites related to discussions about enterprise social media. All participants had multiple years of strategic- and operational-level exposure to social media analytics through their workplace or consulting backgrounds, and three participants were currently leading their own consulting practices related to enterprise social media.
The online panel sessions were facilitated through a hosted solution providing group support system (GSS) functionalityi. Researchers have suggested GSS tools to be a useful technology aid in scenario planning and strategy development (Bryson, 1997; Eden & Ackermann, 1999) . Through the various features available in the GSS, we were able to use a mixture of structured and unstructured mechanisms for data collection in our expert panel sessions. We consistently utilized the nominal group technique (Delbecq & Van de Ven, 1971; Sample, 1984) which allowed participants to create their own list of ideas and submit them for further discussion at their own discretion. The nominal group technique is a structured variation of small group discussion methods, and prevents the domination of discussion by a single person, encourages the more passive group members to participate, and results in a set of prioritized solutions or recommendations (Boddy, 2012; Bryson, 1997; Sutton & Arnold, 2011).
In our online expert panel sessions, the nominal group technique was used in a structured question and answer session format to gather information from participants about the current state of social media analytics, issues and challenges for firms, and recommendations for effective social media analytics strategy. Each online GSS session consisted of multiple rounds of interactions during which panelists proposed, rated, commented, reviewed, and re-rated items related to these topics and related issues.
Findings
The results from the online expert panel session were compiled and summarized into various themes that the participants highlighted as important with respect to the current landscape for social media analytics, as well as guidelines for firms looking at adopting various technologies and processes to enable social media analytics in their organization. The key take-aways from these themes are reported in the following subsections.
Current State of Social Media Analytics: Lack of Business Strategy
All expert respondents in our panel agreed that as a business practice, social media analytics is still in its infancy. Organizations need to put a concerted effort in understanding the potential for using social media metrics and analytics in the context of their business, in defining their business objectives and requirements for utilizing various social media measurements, and in creating an effective implementation strategy for delivering social media analytics as part of day-to-day business intelligence.
One expert compared the current state of social media analytics to the state of World Wide Web (www) in 1995 when only few companies were putting in serious efforts, and most organizations were “merely interested in conversations
about it”.
Several participants also reiterated that organizations need to understand the value that social media analytics can bring in the workflows of many business functions, and this might require various cycles of usage, trial and error, and learning lessons. Development of mainstream social media analytics solutions is dependent on businesses identifying their primary use-cases for social media, testing various tools to enable these use-cases, and mapping the use-cases to business objectives and key performance indicators.
Most participants agreed that with respect to current solutions in the marketplace, there is no dearth of options (free and paid), and a variety of analytics platforms and applications can potentially be utilized by organizations. However, organizations need to spend some time in figuring out which tools provide the intelligence that is aligned with measurements for desired outcomes of their social media initiatives.
Social Media Metrics: Select Few Popular Choices & Standards Still Missing
Almost all panelists agreed that current software suites focus on providing select mainstream social media metrics such as engagement, reach, influence, and sentiment, and businesses have largely been unable to adequately link these and other metrics with key performance indicators for their business objectives. For instance, many businesses are still trying to establish a plausible link between social media activity on their channels and their sales revenue.
Specifically, the panelists highlighted that engagement and reach metrics for social media are typically used by many organizations as a starting point for several reasons. Firstly, marketing professionals who have previously worked with digital marketing campaigns can identify with metrics such as page views, unique visitors, return visits, interaction rate etc. which are commonly reported in social media analytics software in similar and easily understandable terms such as number of fans or followers, number of posts or tweets etc. Linking these metrics to ROI is relatively easier than other more complex and multidimensional analytics. Panel participants suggested that organizations using these metrics while being mindful of the differences between social media channels and traditional websites. Several participants also pointed out to the usefulness of the IAB categorization of social media platforms and relevant metrics for those platforms (Interactive Advertising Bureau, 2009), and recommended its use to organizations getting started with their social media measurement initiatives.
With respect to advanced social media metrics such as sentiment and influence, the panelists highlighted problems and challenges with measuring these composite metrics. Some panelists commented that most algorithms for sentiment analysis are only accurate in the 60% – 70% range, as most software cannot account for detailed cues for sarcasm, context or slang. Other participants agreed and noted that sentiment analysis should primarily be used to gauge the main themes of discussions surrounding a brand, product or service. Similarly with respect to influence metrics, demonstrating cause and effect between individual actions and aggregated outcomes is problematic, and most measurement programs cannot reliably link social media actions to KPIs such as conversion rates in the consumer buying cycle. Most evidence is based on anecdotes, and this withholds the objectivity of any decisions based on influence analysis.
All panelists agreed that a social media analytics program in an organization cannot flourish until the industry agrees upon standard definitions for underlying social media metrics. Until such a time, organizations can focus on defining consistent internal measurements to report the performance of their social media initiatives, but be cautious in trying to infer from cross-company comparisons based on those numbers.
Social Media Measurement and the Elephant in the Room: ROI
Almost all expert panelists acknowledged that return on investment (ROI) remains the key question for social media analytics programs, and that the question keeps getting asked because organizations haven’t yet found a satisfying answer. Specifically, executive level management is very persistent in their demand for social media measurements to uncover and define the ROI of social initiatives in the form of revenue, profits or sales.
Some panelists also indicated that some marketing professionals are experimenting and redefining ROI in terms of social media specific outcomes. For example, some providers and consultants have recommended the use of Return on Influence or Return on Engagement as being more appropriate in the context of social media. However, panel participants concurred that even these new metrics remained “hazy” and “vague” since there isn’t an agreed upon definition. As follow-up comments in the discussion, most participants assented that there currently isn’t a definitive method to measure ROI — in fact the metric may be conceptualized dissimilarly by different businesses. Therefore, it might be useful for businesses to develop their performance dashboards to show ROI from more than one perspective.
Value Driver for Social Media Analytics: Integrated Business Process Workflows
Many panelists repeatedly spoke about the importance and potential of integrated enterprise systems and business process workflows. In order to reap value from social media analytics, organizations need to enable and support business intelligence workflows through the integration of internal data from enterprise systems such as customer relationship management (CRM) with their social listening platforms. With reference to CRM specifically, it was noted that all applications that touch the customer should ideally have a social media component. Additionally, organizations should pursue tighter integration between social media data analysis and other customer intelligence programs such as web surveys. This would allow businesses to validate and act upon consumer needs and sentiments in a more holistic fashion across the customer base.
Future of Social Media Analytics: Advances through Big Data & Predictive Analytics
The panelists in both online expert sessions agreed that the future of social media analytics is closely tied to the exploitation of big data and the emergent field of data science. With unprecedented access to data and computational ability, businesses will finally be able to tap in the abundant structured data in their internal enterprise systems, as well as continuously use social media monitoring tools to scrape unstructured data from the social web. Social networking sites like Facebook are building data centers around the world to collect and process data related to their members’ digital footprints which can potentially be used to aid business decision-making processes — especially in areas related to marketing and brand management. The key, according to expert panelists, would be to effectively utilize big data by overcoming its fragmented nature and by reassembling relevant information and integrating it with other internal sources of data in a time sensitive fashion in order to facilitate real-time business intelligence. Additionally, organizations will need to find effective ways to cull signal from noise and identify the most valuable data and metrics for their business. Only then can businesses work towards an effective analytics program to provide strategic insights.
Many panelists also expressed their opinions about applying predictive analysis techniques to social media data. Many software vendors have started to provide predictive and prescriptive analysis tools alongside descriptive metrics tools. These features can potentially provide guidance on what actions businesses should take in response to other (possibly unsatisfactory) components of the social media metrics scorecard. Many tools show promise in letting analysts discern new market segments, predict sentiment and identify potential customers to be advocates. Overall, the panelists presented a positive outlook for predictive analytics as an advanced technology that can help businesses anticipate rather than react to changing market conditions, and in being more proactive in improving customer relations and pursuing growth opportunities.
Conclusion
“Social media measurement is like driving a modern car. You may have a dashboard with all the lights, toggles, gauges, and metrics, but remember, the most important piece of data to have in front of you is the GPS screen. The GPS screen indicates where you want to go (your objective), where are you now, and how to get there.” (Owyang, 2009)
The statement quoted above by Jeremiah Owyang, a well-known web strategist aptly captures the value and importance of approaching social media analytics as a measurement framework that incorporates strategic objectives and tactical requirements — i.e. as a business intelligence practice.
As organizations get more serious about measuring the effectiveness of their social media initiatives, it is important for them to link their measurement frameworks to high-level business objectives of revenue generation, cost reduction, or operational excellence. Situating a social media analytics program as part of the overall business intelligence strategy provides a practical approach for organizations to get the most of their investments in such initiatives. As evident from the recommendations in the extant literature and key findings from our expert panels, a BI orientation for social media analytics has the potential to provide real-time feedback and actionable insights to help organizations in their decision-making processes. Such an orientation would also enable organizations to improve the tactical execution of social media strategies such as building engagement across various channels and platforms, while directly tying social media metrics to their overarching business objectives.
It is hoped that the discussion in this paper can serve as a basis for establishing a baseline philosophy for businesses partaking various social media initiatives. As a continuation of our research, we aim to utilize our preliminary findings from the literature and the main takeaways from our expert panel findings to develop a more formalized research action plan for social media analytics. Our plans include conducting further empirical research through qualitative interviews and quantitative survey instruments. The expected outcome of our research is a clear delineation of organizational, technological and behavioral factors that affect the adoption and implementation of social media analytics programs in organizations. We aim to utilize our research findings to offer a pragmatic approach to delivering social media metrics linked to strategic business objectives can help enable better delivery of actionable insights to businesses.
Endnotes
i MeetingSphere software (http://www.meetingsphere.com) was used to facilitate the online expert panel sessions.
References
1. Boddy, C. (2012). The nominal group technique: An aid to brainstorming ideas in research. Qualitative Market Research: An International Journal, 15(1), 6-18.
Publisher – Google Scholar
2. Bryson, N. (1997). Supporting consensus formation in group support systems using the qualitative discriminant process. Annals of Operations Research, 71(0), 75-91. doi: 10.1023/A:1018983818299
Publisher – Google Scholar
3. Carlson, K. D., & Kavanagh, M. J. (2011). HR metrics & workforce analytics. In M. J. Kavanagh, R. D.
4. Johnson & M. Thite (Eds.), Human resource information systems: Basics, applications, and future directions (pp. 150-174). Thousand Oaks, CA: Sage Publications, Inc.
5. Delbecq, A. L., & Van de Ven, A. H. (1971). A group process model for problem identification and program planning.The Journal of Applied Behavioral Science, 7(4), 466-492.
Publisher – Google Scholar
6. Eden, C., & Ackermann, F. (1999). The role of GDSS in scenario development and strategy making. String Processing and Information Retrieval Symposium, 1999 and International Workshop on Groupware, 234-242.
Publisher – Google Scholar
7. Etlinger, S., Li, C., & Groopman, J. (2013). Social data intelligence: Integrating social and enterprise data for competitive advantage. (). San Mateo, CA: Altimeter Group.
8. Fisher, T. (2009). ROI in social media: A look at the arguments. Database Marketing & Customer Strategy Management, 16(3), 189-195.
Publisher – Google Scholar
9. Heijnen, J., de Reuver, M., Bouwman, H., Warnier, M., & Horlings, H. (2013). Social media data relevant for measuring key performance indicators? A content analysis approach. Co-created effective, agile, and trusted eServices (pp. 74-84) Springer.
Publisher – Google Scholar
10. Hinchcliffe, D., Kim, P., & Dachis, J. (2012). Social business by design: Transformative social media strategies for the connected company Wiley. com.
Google Scholar
11. Interactive Advertising Bureau. (2009). Social media ad metrics definitions. Retrieved 06/13, 2013, from http://www.iab.net/media/file/SocialMediaMetricsDefinitionsFinal.pdf
12. Jourdan, Z., Rainer, R. K., & Marshall, T. E. (2008). Business intelligence: An analysis of the literature 1. Information Systems Management, 25(2), 121-131. doi: 10.1080/10580530801941512
Publisher – Google Scholar
13. Kaplan, A. M., & Haenlein, M. (2010). Users of the world, unite! the challenges and opportunities of social media.Business Horizons, 53(1), 59-68.
Publisher – Google Scholar
14. Kelly, N. (2012). How to measure social media: A step-by-step guide to developing and assessing social media ROI Que Publishing.
15. Kietzmann, J. H., Hermkens, K., McCarthy, I. P., & Silvestre, B. S. (2011). Social media? get serious! understanding the functional building blocks of social media. Business Horizons, 54(3), 241-251.
Publisher – Google Scholar
16. Lonnqvist, A., & Pirttimaki, V. (2006). The measurement of business intelligence. Information Systems Management, 23(1), 32.
Publisher – Google Scholar
17. Lovett, J. (2011). Social media metrics secrets. Indianapolis, IN: Wiley Publishing, Inc.
Google Scholar
18. Luckham, D. C. (2011). Event processing for business: Organizing the real-time enterprise. Hoboken, NJ: Wiley Publishing, Inc.
Google Scholar
19. Melville, P., Sindhwani, V., & Lawrence, R. (2009). Social media analytics: Channeling the power of the blogosphere for marketing insight. Proc.of the WIN,
Google Scholar
20. Murdough, C. (2009). Social media measurement: It’s not impossible. Journal of Interactive Advertising, 10(1), 94-99.
Publisher – Google Scholar
21. Negash, S. (2004). Business intelligence. Communications of the Association for Information Systems, 13(2004), 177-195.
Google Scholar
22. Owyang, J. (2009). Social media measurement: Dashboards vs GPS. Retrieved 06/15, 2013, from http://www.web-strategist.com/blog/2008/10/16/social-media-measurement-dashboards-vs-gps/
23. Owyang, J. (2011). How corporations should prioritize social business budgets. ().Altimeter Group.
Google Scholar
24. Owyang, J., Lovett, J., Peterson, E. T., Li, C., & Tran, C. (2010). Social marketing analytics: A new framework for measuring results in social media. ().Altimeter Group.
25. Petrocelli, T. (2013). Social media marketing & analytics: Market landscape report. ().Enterprise Strategy Group.
26. Sample, J. A. (1984). Nominal group technique: An alternative to brainstorming. Journal of Extension, 22(2), 1-2.
27. Sterne, J. (2010). Social media metrics: How to measure and optimize your marketing investment. Hoboken, NJ: John Wiley.
Google Scholar
28. Stodder, D. (2012). Customer analytics in the age of social media. ().TDWI Research.
Google Scholar
29. Sutton, S. G., & Arnold, V. (2011). Focus group methods: Using interactive and nominal groups to explore emerging technology-driven phenomena in accounting and information systems. International Journal of Accounting Information Systems,
30. Vedder, R. G., Vanecek, M. T., Guynes, C. S., & Cappel, J. J. (1999). CEO and CIO perspectives on competitive intelligence. Communications of the ACM, 42(8), 108-116.
Publisher – Google Scholar
31. Watson, H. J., & Wixom, B. H. (2007). The current state of business intelligence. Computer, 40(9), 96-99.
Publisher – Google Scholar
32. Zeng, D., Chen, H., Lusch, R., & Li, S. (2010). Social media analytics and intelligence. Intelligent Systems, IEEE, 25(6), 13-16.
Publisher – Google Scholar