Introduction
The paper presents the idea of the new role of knowledge component (KC) in the context of hybrid DSS (Decision Support System) implementation in IT companies. The new role of knowledge in management and organization is closely connected with some changes in implementing a knowledge-management process in/for organizational decision support systems (Young, 1988; Sobolewska, 2020). The basic scope of the paper is to show the importance of knowledge components in knowledge-based organizations in the context of DSS implementation based on the BI system for a future hybrid DSS implementation methodology. The background of the paper is focused on the literature review to show the DSS paradigm, the KM paradigm, and the DSS Meta-Design Methodology with knowledge components in the DDMKCC model. The background showed the new role of DSS in knowledge-based organizations with the idea of a double-loop pattern of knowledge development in/for DSS (Stanek and Sroka, 2000).
The improvement of the knowledge component presented in the theoretical background was verified in a case study based on BI system implementation in a chosen knowledge-based company. The paper presents a case study of BI system implementation for knowledge component improvement within the DDMKCC model (Sprague and Carlson, 1982; Sprague and Watson, 1996; Sroka and Stanek, 2011). The case study is based on a Polish IT company. The following research questions were formulated:
RQ1: How can the knowledge component with the DDMKCC model in BI system implementation be improved in an IT company?
RQ2: What kind of information technology can improve the knowledge acquisition in the DDMKCC model imlemeneted in the BI system in an IT company?
RQ3: How are the enterprise information portals able to improve the knowledge management in an IT company?
The study supported the thesis that BI systems can significantly help improve and develop the knowledge component in knowledge-based organizations.
The case study showed the implementation of a knowledge management system in organizations. There are some key problems in the implementation of such a system because of too many reports, unrevealed facts, and time wasted on digesting reports and messages.
The research results analyses paved the way to overcome the basic problems and showed the concept of smart reporting system implementation due to changing knowledge workers’ expectations.
The paper is divided into five chapters. The introduction describes the aims and the thesis of the paper with the paper’s methodology. The second chapter is based on literature review, presenting the background of DSS development with aspects of the current knowledge management in organizations. The third chapter tackles the research methods with the case study, verifying the research thesis. The fourth chapter presents the research results. Finally, conclusions are presented in chapter five with some recommendations for further research.
Background
Essentials of DSS paradigm
Data remain one of the most important business resources, playing a major role in each and every company. Their actual value, however, depends on how they are used. Therefore, a company pursuing its business goals needs to collect information from best available sources and transform it into knowledge. Well-prepared knowledge can be used to improve the decision-making process in an organization. This process is fundamental in making an organization more competitive. Decisions can be categorized as structured, semi-structured, and unstructured. Structured decision problems have a well-known optimal solution and do not require computerized decision support. For instance, a decision problem involving the choice of the shortest route between two geographical points can be solved analytically with no need to deploy any dedicated systems to help make the right decision. On the other hand, unstructured decision problems have no agreed-upon criteria or solutions, relying critically on the decision maker’s preferences. Between these two types of decision problems – structured and unstructured – there lies a wide strand of semi-structured problems. This is the case in a lot of business decisions that may have some agreed-upon parameters but their solution mostly involves the human factor and relies on human preferences. The processing of semi-structured decisions requires a combination of analytical methods and user-machine interaction to develop alternatives based on pre-defined criteria and eventually reach the optimum solution. To this end, specialized IT systems, namely decision support systems, group decision support systems (Shishany, Kharabsheh and Adams, 2017) and intelligence decision support systems (Skulimowski, 2011), (Turban and Aronson, 2001) with agent systems (Padgham and Winikoff, 2004) are used.
Decision Supports Systems (DSS) are computer-based information systems designed to help managers select one of many alternative solutions to a problem. In a large and sophisticated DSS, it really is possible to automate some of the decision making processes and to speedily analyze an enormous amount of information. Such systems help corporations increase their market share, reduce costs, increase profitability, and enhance quality. Yet, what plays a crucial role in a decision making process is the nature of the problem itself. A DSS is essentially an interactive computer-based information system involving an organized collection of models, procedures, databases, software, communicators, devices, and people, that helps decision makers solve unstructured or semi-structured business problems. The key features of DSS are as follows:
- DSS are able to handle huge amounts of information stored in databases or data warehouses.
- DSS can obtain and process data from many different sources and in multiple formats.
- Using DSS, companies can easily create reports tailored to the user’s needs.
- For data presentation, DSS use both textual and graphical techniques, such as trendlines, tables, charts, etc.
- DSS perform complex, sophisticated analyses and comparisons using advanced software packages.
- DSS can project or indicate the consequences of individual choices.
A general design of a decision support system with orgnizational knowledge component relations and repository is shown in the following studies (Stanek, Sołtysik-Piorunkiewicz and Szary, 2020; Davenport and Prusak, 1998; Davenport, 1994).
Hybrid DSS – the DDMKCC model
The human-computer interaction in decision support systems (DSS) is focused on speeding up the learning process of the user (Klein and Methlie, 1995). A well-designed DSS is one that supports knowledge management in an organization. Since it is a natural property of knowledge that evolves continuously with it, a DSS that is not capable of evolving with the knowledge may not at all bring the expected benefits to the organization. The meta-design approach has had its advocates who undertook to further develop it (Duhon, 1998; Hevner and Hatterjee, 2010; Sobolewska, 2020; Sprague and Carlson, 1982). Due to the volatility and change dynamics of the design space, frequently coupled with a need for organizational change entailed by IT deployment, an integration of current trends in system design and organizational change occurs (Fry, 2009). The meta-design methodology provides a higher-level abstract rules supporting the working methodology framework used in the DSS development process. Meta-design literature supplies a variety of such rules, some of which deserve to be underscored (Hevner and Hatterjee, 2010; Fischer and Giaccardi, 2004) as follows:
- Developing socio-technical environments that support and empower users to engage in the process of system development not only at design time but also at use time,
- Embodying the human ability of autopoiesis in projects for which the “designing the design” process is a first-class activity,
- Supporting social creativity by providing the technical and social conditions for the exchange of ideas during workshops, discussions, debates, brainstorming, co-creation sessions and other forms of intense collaboration,
- Combining art and design in the processes of self-realization,
- Use of meta-analysis for comparing, combining, synthesizing, summarizing, specifying, and generalizing previous studies.
Dan Power (Power, 2002; Power, 2013; Power, 2003) perceives the following advantages of employing the architecture approach within DSS projects: better collaboration; easier planning, implementation and integration with other systems; and an augmented ability to evaluate technology. Clyde Holsapple, one of the fathers of the DSS concept (Burstein and Holsapple, 2008; Holsapple, 2008), commented that “DSS architecture does not define what DSS is; rather, it functions as an ontology that gives a common language for design, discussion, and evaluation of DSS’. This perspective on DSS corresponds with Lambert’s opinion that “the architectural design should set a common level of understanding among technical, non-technical and management participants”. Suresh Basandra (Holsapple, 2008), on the other hand, believes that “system architecture is the process of partitioning a software system into smaller parts”. The above mentioned insights lead to Holsapple’s (Holsapple, 2008) definition of the DSS architecture: “DSS architecture is a general framework that identifies essential elements of a DSS and their interrelationships”. Sprague and Carlson (Sobolewska, 2020) proposed the Data-Dialog-Modeling design paradigm as a DSS architecture.
The evolution of the DSS meta-design methodology revolves around six components. Ensuing research endeavors by (Sobolewska, 2020; Sprague and Carlson, 1982) and business studies by (Turban and Aronson, 2001; Twardowski, Wartini-Twardowska and Stanek, 2011), contributed extensions of the DDM paradigm into the DDMKCC Model (Sprague and Carlson, 1982).
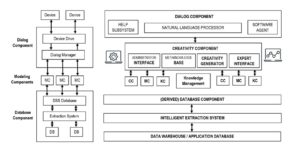
Figure 1: Overview of the DDMKCC Model with a Knowledge-Centric Component.
Source: (Sprague and Carlson, 1982; Sprague and Watson, 1996; Sroka and Stanek, 2011).
The DDMKCC solution includes six, rather than three, components: (1) data (DaC), (2) dialog (DiC), (3) modeling (MC), (4) knowledge (KC), (5) communication (CC), and (6) creativity(CrC). The existing evolution trends include innovative solutions (data fusion, BI) to produce new DSS characteristics, such as situational awareness, cognitive bias, etc.; interface agent (personal assistant, avatar) technology and ergonomic design of the human-machine dialog as a challenge for the user interface subsystem; combining heterogeneous models (a hybrid model) in such a way that their concerted actions produce synergy and increase overall applicability.
The positioning of DDMKCC with a knowledge-centric component (Figure 1) as a DSS meta-design methodology provided an opportunity to revisit the perception of the knowledge component, focusing attention on its role. Now, with the proliferation of BI systems and the Big Data approach, the DSS meta-design methodology strongly emphasizes the knowledge component.
The concept of Business Intelligence systems encompasses issues related to the presentation of corporate data in the form of intelligible and transparent reports delivered to decision makers. As a result, key organizational decisions can be made at a faster pace without having to employ a number of analysts to read information from a large number of diverse sources, e.g. from different transactional systems that do not integrate with one another.
The BI system is described as the processes, technologies and tools needed to turn data into information, and information into knowledge, and knowledge into plans that drive profitable business actions. BI encompasses data warehousing, business analytics and knowledge management. From another point of view, the BI system is defined as the knowledge gained about a business through the use of various hardware/software technologies which enable organizations to turn data into information.
Based on widespread definitions and relevant literature sources, the goals that the Business Intelligence systems are supposed to achieve can be summarized as follows:
- Automation of business operations;
- Informing decision-makers;
- Accelerating and improving decision making;
- Optimizing internal business processes;
- Increasing operational efficiency;
- Driving new revenues;
- Gaining competitive advantages over business competitors;
- Identifying market trends;
- Spotting business problems that need to be addressed.
The basic functionalites of BI systems are to provide historical, current, and predictive views of business operations; to use data that has been gathered into a data warehouse; to work from operational data; and to support reporting with interactive “slice-and-dice” pivot-table analyses, visualization, and statistical data mining. The functionality of a BI system is connected with information gathering from an internal and external sources of the company. The source of all information that is processed and analyzed by the Business Intelligence system is collected in the core of each system – the data warehouse (DW). The DW stores information from a variety of sources, such as spreadsheets, text files, and transactional databases. As BI systems were created to develop reports supporting corporate decision makers, they must be capable of analyzing data from finance, accounting and sales departments. Therefore, the data warehouse primarily sources information from transaction systems, e.g. financial and accounting systems, such as MRP, CRM, etc. Since BI systems are targeted at data visualization and analysis, they can also be fed by: GIS (Geographic Information System) designed for the visualization of geographic data; EIS (Executive Information System) – a type of management information system that facilitates and supports senior executive information and decision-making needs; and MIS (Management Information System) – systems designed to support the management of enterprises (Morawiec and Sołtysik-Piorunkiewicz, 2020). EIS provides easy access to internal and external information relevant to organizational goals. It is commonly considered to be a specialized form of decision support systems (DSS).
The Role of Knowledge Development in IT Companies
In today’s organizations, structured business processes and decision problems are just the tip of an iceberg (Sobolewska, 2020; Szelągowski, 2019). The increasing prevalence of dynamic semi-structured and unstructured processes shifts attention to Adaptive Case Management (ACM) (Svenson, 2010) founded on the effective collaboration of knowledge workers. It is therefore knowledge workers and their performance that have arisen – as predicted by Drucker (Drucker, 1999) – as a key challenge for businesses. The methodology underpinnings for learning and knowledge acquisition by knowledge workers have been addressed by prior DSS studies (Moore and Chang, 1983; Nonaka and Takeuchi, 1995; Osuszek and Stanek, 2015; Stanek, 2010; Stanek, 1998; Stanek, 1999; Stanek and Sroka, 2000; Stanek, Twardowska and Twardowski, 2013; Twardowski, Wartini-Twardowska and Stanek, 2011). What is at stake here is the consistent support involving respective components of the extended DDMKCC paradigm, which are presented as follow:
- The need to enhance analytics (DaC) relates to the metaphor of “blind surgeons” – former stakeholders in a business process who performed their roles while being oblivious of the prevalent trends (Van der Aalst, Weske and Grünbauer, 2005), as opposed to knowledge workers who are increasingly aware of recent developments (with data warehouses, machine learning, Big Data, and Data Lakes on hand) and empowered to revise processes as they go.
- Effective and intuitive human-machine dialog (DiC) is key to making the most of the available technologies, i.e. of computers linked to increasingly standardized ecosystems/platforms and supported by advanced artificial intelligence, visualization and augmented reality tools.
- A knowledge worker who can perform simulations employing an array of computerized models (MC), standardized notations (BPMN, CMMN, UML, EPC) and software applications (Pegasystem, IBM Case Manager, Apian, Tibco) is increasingly aware of the decision context and capable of assessing the relative outcomes of available alternatives.
- Learning processes, drawing on a variety of technologies (BI, knowledge portals), fuel the conversion of data into meaningful information (KC), and trigger knowledge development and validation as well as the creation of meta-knowledge and parameter-based scenarios for future use (cf. Figure 2).
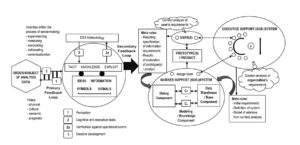
Figure 2. The double-loop pattern of knowledge development in/for DSS.
Source: (Stanek, 2010; Stanek, 1998).
- It is within communication processes (CC), whether face-to-face or indirect, that team projects are executed, group decision-making takes place, relationships are established and nurtured, and knowledge is disseminated (outdated and falsified). The immense number and diversity of available tools will suffice alone to mark the significance of this component. Relevant experiences can be sought in the application of agile technologies and SMAC systems (Social Media, Mobile, Analytics & Big Data, Clouds).
- Creativity and innovation (CrC) represent knowledge workers’ most valuable potential that can be supported and developed using the latest methodologies and technologies (Remy, Vermeulen, Frich and Biskjaer, 2020; Sroka and Stanek, 2011; Stanek and Sołtysik-Piorunkiewicz, 2011a, 2011b).
Based on the data accumulated, cleaned and loaded into the organizational data warehouse, the reporting system creates reports or computes indicators that can be used by decision makers (e.g. KPI – Key Performance Indicators). Further, the information feeds hypotheses that are set forth and validated. In the next step, with time elapsed and increasing experience, an organizational knowledge base is built, forming a repository of know-how unique to the organization, accounting for its market position and competitive advantage.
Business Intelligence systems continue to be a very important group of analytical systems and are likely to remain in use for a long time. However, over time and with increasing volumes of information being processed, they tend to turn into completely new types of analytical AI-driven solutions. The curent studies show the context of AI usage for knowledge workers (Greiner, Jovy-Klein and Peisl, 2020)
In this case study, the trend in the evolution of BI systems is outlined on the example of an intelligent reporting system.
Big Data are made up of information generated by users of web portals, sourced from Internet transaction data and scientific outcomes (biological, astronomical, physical measurements, etc.), contributed by robots searching the Internet (Web mining, Web crawling), etc. Most Big Data have a non-relational structure and are chiefly composed of texts and images; however, they can also include metadata or graphical data tracing connections between websites, and other similar input. Hence, no specific data model is assigned to the concept of Big Data. The most important features of Big Data are very well characterized by the following 5 Vs: Volume – huge volume, Velocity – high-rise speed, Variety – diversity of structure and content, Veracity – reliability and accuracy, Value – actual value for decision making processes.
The implementation of DSS involves a number of technological and organizational aspects relating to business process management. In recent years, systems capable of processing very large data sets – Big Data – have gained an important position, responding to the need for tackling an increasing volume of more and more complex data coming in diverse, as well as non-standard formats that cannot be stored in traditional databases or data warehouses. This requirement implies the use of Big Data systems that can, automatically and in real-time, download, process and analyze huge collections of petabytes of data characterized by great diversity and high variability in time.
According to one of the Big Data’s definitions proposed by V. Mayer-Schönberger and K. Cukier (Mayer-Schönberger and Cukier, 2014), this term includes whatever can be implemented on a large scale and cannot be implemented on a small scale in order to acquire new knowledge or create new value that will change markets, organizations and relations between governments and citizens, etc. The above mentioned definition portrays Big Data as a revolutionary and innovative concept that will alter the functioning of contemporary and future enterprises, institutions and workplaces, and that will be reflected in all facets of human life.
The revolutionary and innovative nature of large data set processing has also been noticed in Poland. The Center for Digital State Studies has brought attention to the linkages and impacts between Big Data or social media and such technology trends as the Internet of Things or mobile solutions.
This new type of systems involves a different approach to data being processed. In setting the standard for analyzing large data sets, Schönberger and Cukier (2014) stress that, with Big Data, precision and accuracy are no longer the key criteria, given the diverse and unstructured nature of data being processed; it will mostly suffice to indicate the general trend of change.
According to e.g. Schönberger and Cukier (2014), as well as Morawiec and Sołtysik-Piorunkiewicz (2020), in processing large data sets, one should move away from searching for causality in favor of discovering relationships. For its operation, Big Data relies on specific algorithms, such as Map-Reduce or Apache Hadoop, and statistical languages such as R, Python or Matlab (Morawiec and Sołtysik-Piorunkiewicz, 2020).
The Research Study
This chapter presents the case study of BI system implementation in an IT company for the sake of refining the knowledge component in line with the DDMKCC model. The research hypothesis was stated as follows: “In IT companies, the knowledge component can be and should be improved in the first place”. The research questions were formulated as follow:
RQ1: How can the knowledge component with the DDMKCC model in BI system implementation be improved in an IT company?
RQ2: What kind of information technology can improve the knowledge acquisition in the DDMKCC model imlemeneted in the BI system in an IT company?
RQ3: How are the enterprise information portals able to improve the knowledge management in an IT company?
The case study of Business Intelligence system implementation involves CONSORG SA. This joint-stock company boasts nearly 30 years of market presence and, throughout this period, has conducted a number of IT and consulting projects for many major Polish companies. Its business profile encompasses the design, implementation and oversight of IT solutions, as well as business process optimization and management support services. CONSORG specializes in the implementation of modern IT solutions in the field of budgeting and controlling, as well as supporting the management of capital groups, with particular emphasis on the issues of the consolidation of financial statements. For these cases, the previous DSS research studies were conducted on CONSORG as well (Stanek, Twardowska and Twardowski, 2013; Twardowski, Wartini-Twardowska and Stanek, 2011). The study is focused on an in-company BI system implementation process at CONSORG SA and describes the subsequent stages of the process.
The knowledge component with the DDMKCC model in BI system implementation in IT companies
System implementations in the IT industry owe their unique character to the environment and the way in which they are performed. IT companies tend to not use so much the services of external companies to deal with all of the software development and implementation process, instead they rely on their own resources and handle the entire process on their own. For this reason, the process differs from the usual practice in other industries. As a rule, system implementations performed in IT companies take less time and expenditure because they do not involve intermediary or service companies.In addition, businesses dealing with IT implementations on a daily basis will already have relevant insights into customers’ requirements, valuable links with software suppliers and, in most cases, ample experience with business-to-business collaboration and project management.
The analytical system implemented at CONSORG SA was primarily addressed and tailored to the needs of top managers, project managers, department managers (in many cases, being the same people acting in different roles), and the sales personnel. The system was expected to enable these actors to monitor project implementation processes on a continuous basis, controlling deviations from plan and analyzing time spent on projects. For top managers solely, the system was supposed to provide reports on budget performance, support strategic decision making, and facilitate projections into future developments based on financial result forecasts for the following reporting period.
The kind of information technology to improve the knowledge acquisition in the DDMKCC model implemented as a BI system in IT companies
CONSORG SA’s system draws on Microsoft technologies and products from the MS SQL Server family, such as OLE DB and Analysis Services – for data analysis, or ASP.NET – for data presentation and visualization. Microsoft SQL Server ranks among the most advanced database solutions available in the market and has been the market’s favorite for years. Bundled with analytics tools, it can effectively work with large sets of diverse data and can serve as a platform underpinning an organization-wide analytical system.
SQL Server supports the R language, a leading data science and analysis solution attuned to the prevalence of Big Data, recently upgraded to provide better data security through the incorporation of such encryption and security technologies. SQL Server’s functions include:
- Always Encrypted – for the encryption of data at read and save time, requiring client applications to run the dedicated Always Encrypted driver for data decryption;
- Row-Level Security – a service protecting records in data tables from being modified by any users other than those specifically authorized to do so;
- Dynamic Data Masking – making it possible to hide some sensitive data from unauthorized users.
Other functions in the SQL Server provide further facilities relating to data access, analysis and reporting (e.g. automated KPI calculation or R Language integration), making it a powerful technology that comes in handy in a wide array of environments.
OLE DB (Object Linking and Embedding Database) is a collection of COM (Component Object Model) objects that makes it possible to retrieve data from various sources. The OLE DB interface aims to provide all database applications with a single method of accessing data from diverse sources. At the same time, OLE DB represents a beta version – an in-development implementation – of the Microsoft-made ODBC (Open Database Connectivity) standard that can handle, alongside SQL queries, requests in other query languages, as well as queries involving non-relational databases and other data sets, such as spreadsheets and object-oriented databases.
Analysis Services form part of the Microsoft SQL Server and are included in the SQL Server Data Tools (SSDT) alongside Integration Services interfacing between data and Report Services – the latter reporting data analyses to Azure cloud services. SQL Server Analysis Services (SSAS) are capable of multidimensional data modeling, including (since 2012) tabular modeling and integrating with Power Pivot. In the BI solution being implemented, SSAS is responsible for generating OLAP cubes from diverse-source data fed into the system and for processing MDX (Multidimensional Expressions) queries built automatically via a graphical interface. For its operation, Analysis Services depend on the DAX (Data Analysis Expression) language – a collection of function libraries employed in calculating KPIs or creating calculated columns. DAX also includes a number of statistical, logical and filtering functions.
In addition, ASP.NET is used as a comprehensive solution for website development in presenting data analyses. A variety of .NET Framework is utilized in the system for website design and web application development. ASP.NET is an open-source software consisting of three component frameworks geared to web application development: Web Forms – a drag-and-drop tool for dynamic website design; ASP.NET MVC – a solution based on the Model View Controller template for use in web application design and mobile application design (e.g. for Android) allowing users unfamiliar with the .NET language to easily develop applications; ASP.NET Web Pages – an integration tool that combines HTML code with cascaded style sheets (CSS) and database layers.
Prior to the BI system implementation, the company’s IT infrastructure was made up of a financial and accounting system and Impuls Evo software by BPSC S.A.
The new BI system was aimed to add the following functionalities:
- A chart of accounts on top of financial and book-keeping records from which data are retrieved for financial analyses and forecasts built using MS Azure and MS Power BI architecture.
- Doc Studio – a data collection system for project planning and assessment of time consumption.
The enterprise information portals of BI system improvement in IT companies
The in-design BI system consisted of four main components, i.e. webStudio, deskStudio, xlStudio, and wordStudio, targeting different users: the tools of webStudio as a web application are designed for key users and/or top managers, because of management portal where data are presented in the form of transparent managerial dashboards; the deskStudio tools for analytical teams, allowing them to create complex reports and present them in the form of charts; the xlStudio tools for budgeting departments staff, helping them enter data from Microsoft Excel spreadsheets into budget forms; and the wordStudio tools for faculty users, generating reports in the form of Microsoft Word documents that can be circulated among the company’s employees.
The subsequent stages of the BI system implementation process were designed with flowStudio, etlStudio, and calcStudio. These subsystems, making up the Business Intelligence solution, were being discussed. The flowStudio tools were used for planning and supervising group work, supporting the execution of business processes and enabling the analysis of deviations from plan. The etlStudio tools were important due to the ETL process. The ETL creator has beed used in the analytical processing of data, allowing users to save these data in a data warehouse. The calcStudio auxiliary tool was used to help generate billings and create a billing matrix through input filtering and transformation.
Arguably, the key system components are the webStudio and deskStudio applications. webStudio is an analytical tool based on OLAP architecture, relying on a multidimensional model (MOLAP) for its operation. Its role consists in creating reports from aggregated warehouse data coupled with data from transaction systems and data entered by users. The program is able to generate parameterized and fully scalable reports for a single user as well as for user groups – in the form of interactive tables, dashboards or key indicators. The application uses the ASP.NET web technology for data presentation.
deskStudio is an analytical tool based on OLAP architecture, largely similar to webStudio. It is a desktop application that uses the OLE DB interface as the source of data for processing and Analysis Services to process data in the form of OLAP cubes. It includes pivot tables functionality for ad hoc analysis. The application is utilized by the company’s analytical team to launch budget performance forecasts, as well as to analyze returns on future projects and analyze the impact of changes and deviations from plan on ongoing projects.
The company’s analysts have thus been given the possibility of visualizing data contained in the warehouse in tabular form, exporting data to Microsoft Excel or Microsoft Word (they can be read in real time using the xlStudio and wordStudio add-ons described in the following sections), creating advanced MDX queries (used in Analysis Services for data management) as well as of writing their own scripts in programming languages supported by the Analysis Services platform (C#, Visual Basic).
Other reports generated in the Business Intelligence system include a tabular presentation of the company’s sales, linked to information on deviations from plan and next-period forecasts; a presentation of revenues from sales as a line chart to depict budget performance or as a bar chart to illustrate operating profit; as well as a presentation of deviations from plan in the form of dashboards and matrix charts.
Taken together, the factors mentioned above, answering the RQ1, RQ2 and RQ3, seem to support the hypothesis that: “In IT companies, the knowledge component can be and should be improved in the first place for knowledge management”
The Research Results and Discussion
The case study of the DDMKCC model based on BI systems implementation in an IT company showed some problems of knowledge management in organization. Too many reports, unrevealed facts, and time wasted on digesting reports and messages – these are the key problems that most knowledge workers struggle with, on an everyday basis. Is there a way to overcome these problems? The research results analyses have the answer for smart reporting system implementation.
The rapidly changing economic conditions and the uncertainties around the COVID-19 pandemic have put businesses in a stint of profound transformations. The business models that have been pursued by businesses are heavily based on data analysis, and the quantity of data has been growing exponentially. Given the excess of information and reports to be analyzed, they need better ways to handle the incoming data and timely make key business decisions. It is smart reporting* that might bring the much-desired solution to the problem.
A smart reporting system should be able to eliminate or mitigate at least some of the weaknesses of traditional reporting. Hence, to be able to reduce the amount of redundant information, the system must know what should be reported and how to make the data more meaningful and intelligible. In addition, it ought to be capable of tailoring the visualization option to best suit each user’s preferences and to offer the optimum path for analysis that will engage the user in the problem rather than walking him through irrelevant facts. Finally, it might be a good idea to make sure that the reports come at the right time, bringing alerts to a minimum.
While it may seem obvious, the question is: how can the system know what should be reported, and what should not? The answer is in making assumptions on the following three areas:
- Analyzing the problem context – where the context is found in the knowledge base;
- Analyzing the relationships – links to the knowledge base;
- The choice of alerts, if applicable – alerts may be indicative of threats as well as of opportunities.
What a smart reporting system must be able to do is to screen a collection of available data for items that should be reported, whether indicative of threats or opportunities. It is basically up to the knowledge base designer to decide what kind of information will be interpreted as reportable. The system can accommodate any number of knowledge bases that may operate standalone or in conjunction, while linkages among them may be based on data types (where an analysis entails mining a dedicated knowledge base) or on sharing a common data source (where a data item triggers an analysis in other independent knowledge bases). Depending on the designer’s choices, reported data items (signals) can be grouped and filtered e.g. by weight. They can also become a starting point for a more in-depth analysis.
Knowledge required to identify problems is stored as a set of business rules that are processed via the open-source engine called Drools – a Business Rules Management System (BRMS). Beside the basic business rules engine (BRE), it comprises a web development and rules management application (Drools Workbench).
The business rules engine includes connectors enabling it to access data from independent sources, such as databases and data warehouses, spreadsheets, etc., to process mathematical models, or to incorporate artificial intelligence mechanisms, such as fuzzy logic and learning systems.
Self-service BI is among the top five BI trends of 2020, hence, an emphasis was placed, by the authors throughout the research process, on ensuring that users (designers) can create their own business rules.
To this end, a proprietary business rules editor was developed to make it possible for designers, using the original Drools format, to compose new rules, whether in text form, the same way they would in natural language, or in graphical form.
In addition to business rules, knowledge bases designers can define visualization rules. As a result, they can influence the way the system will display specific types of information. They can choose to just define a number of individual signals as well as to build signal groupings and develop complete managerial desktops geared to view them. Whichever approach they choose, however, they will never be able to predict the actual configuration of signals generated from a knowledge base. It is the system itself that has to make sure that the data are presented in a manner that not only makes sense to the user but also supports horizontal (across the problems identified) and longitudinal analysis (in-depth to understand the underlying factors). This means that, for example, where too many incoming signals are identified as presentable to the user, they should be split into a number of desktops (views); where the system encounters presentable data lacking visualization rules, it will follow its internal rules to automatically generate visualizations, e.g. it may use a line chart to present a time series. Further, the system will attempt to suit the choice of visualization techniques to the user profile, so the same data may be presented differently to different users.
At the same time, smart reporting may work in a way that resembles classic reporting, i.e. it has to be initiated by the user who either wishes to explore a particular area (whether a single knowledge base or a number of interlinked knowledge bases) or requires comprehensive information on existing problems (which results in throwing up all available analyses).
The reporting function may also run in a continuous mode, even if, for the sake of smooth performance, it will probably not be able to work with all available data sources, instead it will be plugged into a so called data stream, e.g. a transactional system, to monitor records and capture irregularities. If this is the case, reporting is mostly confined to conveying brief messages to the user (by e-mail, via a messaging service, etc.), whereas detailed reports are not delivered unless the user initiates the process. The parctical example of solving the problem based on knowledge management in the organization is tarrying in the field of budgeting and controlling as well as supporting the project management.
Conclusions
The paper highlights the new role of hybrid DSS in an IT company and the concept of Meta-Design Methodology for knowledge component improvement in the DDMKCC model. The extensive literature review performed, the case study conducted, and the research work involved have led the authors to advocate the thesis that the use of BI systems can effectively support the development of the knowledge component in DSS environments. Future efforts should focus on the use of AI and Big Data software to improve human-computer interaction in creativity support, modeling and simulation contexts. More specifically, researchers need to further explore applicable approaches and AI technologies for optimum performance. A central dilemma is likely to be whether computers should think and act like humans, or whether they should be based on rational thinking. It is well known that human decisions can be biased by emotions, so even if people try their best to be objective, they will never be so.
One more thing to realize is that giving more power to machines will always raise fears among people – that machines, once beyond human control, might take over our world and destroy mankind. To mitigate the fears, it should be said, in the first place, that we have not even begun to understand what human consciousness is, so we better think about thatbefore we can put anything like it in computers. Second, we already are governed or tracked by machines in many areas of our lives: computerized systems help us pick a movie, decide if we can get a loan, test our health condition, or predict our purchasing decisions to make sure the right products are in stock.
Advanced computer algorithms have already proved to be able to deliver better results than humans and are becoming more and more common in business applications. Importantly enough, they do not threaten to immediately rid people of their jobs; more likely, they are going to change our world the way that the Industrial Revolution did. As AI grows into new subdomains – Virtual Reality, Expert Systems, Natural Language Processing, Deep Learning, Neural Networks, and Speech Recognition – additional skills and an increasing number of knowledge workers will be needed.
[*] The solution is an outcome of the research and development project entitled “The Development of Advanced Smart Algorithms for Context-Based Selection and Visualization of Content Reported”, conducted by CONSORG and co-funded by the European Union under the RPO WSL Program for 2014-2020.
References
- Van der Aalst W.M.P., Weske M., Grünbauer D. (2005). Case Handling: A New Paradigm for Business Process Support, Data & Knowledge Engineering 53, Elsevier.
- Burstein F., Holsapple C.W. (eds.) (2008). Handbook on Decision Support Systems 1 – Basic Themes, Springer, Berlin-Heidelberg, pp. 163-190.
- Davenport T. H., Prusak L. (1998). Working Knowledge. Harvard Business School Press, Boston.
- Davenport, T. H. (1994). Saving IT’s Soul: Human Centered Information Management. Harvard Business Review, March-April, 72 (2)pp. 119-131.
- Drucker P. (1999). Management Challenges for the 21st Century, New York: Harper Collins, p. 157.
- Duhon, B. (1998). It’s All in our Heads. Inform, September, 12 (8).
- El-Gayar O.E., Deokar A.V., Tao J. (2013). DSS-CMM: A capability maturity model for DSS development processes, [in:] Engineering Effective Decision Support Technologies. New Models and Applications, ed. D. Power, PA: IGI Global, Hershey.
- Fischer G. (2010). End user development and meta-design: Foundations of participation, “Journal of Organizational and End User Computing”, vol. 22, no. 1, pp. 52-82.
- Fischer G., Giaccardi E. (2004). Meta-design: A framework for the future of end-user development, [in:] End User Development – Empowering People to Flexibly Employ Advanced Information and Communication Technology, eds. H. Lieberman, F. Paterno, V. Wulf, Kluwer Academic Publishers, Dordrecht.
- Fry T. (2009). Design Futuring: Sustainability, Ethics and New Practice, Berg, Oxford.
- Greiner Ch., Jovy-Klein F., Peisl T. (2020). AI as Co-workers: An Explorative Research on Technology Acceptance Based on the Revised Bloom Taxonomy, [in:] K. Arai et al. (Eds.): FTC 2020: Proceedings of the Future Technologies Conference (FTC) 2020, Volume 1, pp 27-35, Part of the Advances in Intelligent Systems and Computingbook series (AISC, volume 1288), https://doi.org/10.1007/978-3-030-63128-4_3.
- Hevner A.R., Hatterjee S. (2010). Design research in information systems, Series: Integrated Series in Information Systems, vol. 22.
- Holsapple C.W. (2008). DSS architecture and types, [in:] International Handbooks on Information Systems.
- Keenan P. (2006). Spatial Decision Support Systems: A coming of age,.
- Klein M., Methlie L.B. (1995). Knowledge-based Decision Support Systems with Applications in Business, John Wiley & Sons, Chichester.
- Marston S., Lia Z., Bandyopadhyaya S., Zhanga J., Ghalsasi A. (2011). Cloudcomputing – the business perspective, “Decision Support System”, April 2011, vol. 51, issue 1, pp. 176-189.
- Mayer-Schönberger V., Cukier K. (2014). Big Data: A Revolution That Will Transform How We Live, Work, and Think, American Journal of Epidemiology, Vol. 179, No. 9, pp. 1143–1144.
- Moore J.H., Chang M.G. (1983). Meta design considerations in building DSS, [in:] Building Decision Support Systems, ed. J.L. Bennett, Addison Wesley, Reading 1983, pp. 173-204.
- Morawiec P., Sołtysik-Piorunkiewicz A. (2020). The new role of cloud technologies in management information systems implementation methodology, [in:] K. Arai et al. (Eds.): FTC 2020: Proceedings of the Future Technologies Conference (FTC) 2020, Volume 3, pp. 423-441, Part of the Advances in Intelligent Systems and Computingbook series (AISC, volume 1290), https://doi.org/10.1007/978-3-030-63092-8_29.
- Nonaka, I. & Takeuchi, H. (1995). The knowledge creating company: How Japanese Companies Create the Dynamics of Innovation. New York: Oxford University Press.
- Osuszek L., Stanek S. (2015). Knowledge Management and Decision Support in Adaptive Case Management Platforms, [in:] Annals of Computer Science and Information Systems, vol. 5, Proceedings of the 2015 Federated Conference on Computer Science and Information Systems (FedCSIS), pp. 1539-1549.
- Padgham, Winikoff M. (2004). Developing Intelligent Agent Systems: A Practical Guide, Wiley J.
- Power D.J. (2002). Decision Support Systems: Concepts and Resources for Managers, Quorum Books, Westport, Connecticut.
- Power D.J. (2013). Decision Support, Analytics, and Business Intelligence, Business Expert Press, New York.
- Power D.J. (2003). Defining decision support constructs, [in:] DSS in the Uncertainty of the Internet Age, eds. Bui, H. Sroka, S. Stanek, J. Gołuchowski, AE Katowice, Katowice, pp. 51-61.
- Remy C., Vermeulen L., Frich J., Biskjaer M. (2020). Evaluating Creativity Support Tools in HCI Research, DIS ’20: Proceedings of the 2020 ACM Designing Interactive Systems Conference, pp. 457–476.
- Shishany A., Kharabsheh R., Adams J. (2017). The Future of Group Decision Support System Supported Meetings: Perceiving the Value and the Need for Competitive Strategies,”International Review of Management and Marketing, Econjournals, vol. 7(2), pages 263-271. (Shishany, Kharabsheh, Adams, 2017)
- Skulimowski A. (2011). Future Trends of Intelligent Decision Support Systems and Models, Communications in Computer and Information Science Vol.2,:pp.11-20.
- Sobolewska O. (2020). Knowledge-oriented business process management as a catalyst to the existence of network organizations, Journal of Enterepreneurship, Management and Innovation (JEMI), Volume 16, Issue 1, pp 107-132.
- Sprague R.H., Carlson E. (1982). Building Effective Decision Support Systems, Upper Saddle River, Prentice-Hall, New York.
- Sprague R.H., Watson H.J. (1996). Decision Support for Management, Upper Saddle River, Prentice-Hall, New York.
- Sroka H., Stanek S. (2011). Creativity Support Systems, Wydawnictwo Uniwersytetu Ekonomicznego w Katowicach, SE 88.
- Stanek S. (1998). Links between executive support and worker support: software agents for network management, [in:] Context Sensitive Decision Support Systems, eds. D. Berkeley, G. Widmeyer, P. Brezilion, V. Rajkovic, International Federation for Information Processing IFIP, Chapman&Hall, pp. 227-246.
- Stanek S. (1999). Metodologia budowy komputerowych systemów wspomagania organizacji, Prace Naukowe, AE Katowice, Wydawnictwo Uczelniane AE Katowice, Katowice.
- Stanek S. (2010). Ubiquitous Computing Infrastructure as a Basis for Creativity Support.” In: Frontiers in Artificial Intelligence and Applications, Vol. 212: Bridging the Socio-technical Gap in Decision Support Systems, A. Respicio, F. Adam, G. Phillips-Wren, C. Teixeira, J. Telhada (Eds), IOS Press, pp. 99-108.
- Stanek S., Sroka H. (2000). The Double Loop Pattern of Knowledge Development in/for DSS Research, [in:] Decision Support through Knowledge Management, eds. S. Carlsson, P. Brezillon, P. Humphreys, B. Lundberg, A. McCosh, V. Rajkovic, Department of Computer Systems Sciences, University of Stockholm and Royal Institute of Technology, Stockholm, pp. 349-367.
- Stanek, S., Sołtysik‑Piorunkiewicz, A. (2011a). Analiza porównawcza mind i concept mapperów. W: Wiedza i komunikacja w innowacyjnych organizacjach. Komunikacja elektroniczna, (red.) M. Pańkowska, Uniwersytet Ekonomiczny w Katowicach, Katowice.
- Stanek, S., Sołtysik‑Piorunkiewicz, A. (2011b). Building creative decision support systems for project management. Mind and concept mapping methodologies, [In:] Creativity Support Systems, red. Sroka, S. Stanek, Studia Ekonomiczne, No. 88, Uniwersytet Ekonomiczny w Katowicach, Katowice.
- Stanek, S., Sołtysik‑Piorunkiewicz, A., Szary, M. (2020), The Implementation of DSS in Knowledge-Based Organizations – the New Role of Knowledge Components in DSS Meta-Design Methodology”, Proceedings of the 36th International Business Information Management Association (IBIMA), ISBN: 978-0-9998551-5-7, 4-5, November 2020, Granada, Spain.
- Stanek S., Twardowska J., Twardowski Z. (2013). The DDMKCC Decision Support Architecture in the Light of Case Studies, [in:] Annals of Computer Science and Information Systems, vol. 1, Proceedings of the 2013 Federated Conference on Computer Science and Information Systems (FedCSIS), pp. 1169-1176.
- Szelągowski M. (2019). Dynamic Business Process Management in the Knowledge Economy. Creating Value from Intellectual Capital, Lecture Notes in Networks and Systems, Volume 71 Springer.
- Svenson K.D., (2010). Mastering the Unpredictable: How Adaptive Case Management Will Revolutionize the Way that Knowledge Workers Get Things Done, Meghan-Kiffer Press.
- Turban E., Aronson J.E. (2001). Decision Support Systems and Intelligent Systems, Upper Saddle River, Prentice Hall, New York.
- Twardowski Z., Wartini-Twardowska J., Stanek S. (2011). A decision support system based on DDMCC paradigm for strategic management of capital groups, [in:] Advanced Information Technologies for Management AITM 2011 – Intelligent Technologies and Applications, Research Papers of Wrocław University of Economics no. 206, eds. J. Korczak, H. Dudycz, M. Dyczkowski, Publishing House of the Wrocław University of Economics, Wrocław.
- Young L.F. (1988). Worker Participation Support Systems: A Missing Link in Organizational Computer Support, in Organizational Decision Support Systems (eds Lee R.M., McCosh A.M. and Migliarese P.) IFIP Working Group 8.3., Lake Como.