Introduction
Public programmes are primarily viewed in terms of effects with little attention paid to how those effects are produced. Measuring average impacts of public interventions, which is a dominant approach in the evaluation of public programmes, has little to offer to inform policy making: whether the programme can be successfully scaled-up, implemented elsewhere or for other recipients, what to do when a programme does not yield expected effects. Hence, recently more attention is paid to the variation in program effects and mechanisms through which these effects occur (Astbury and Leeuw, 2010). Addressing these kinds of problems means moving beyond the traditional input/output approach and trying to understand what is inside the ‘black box’ of a policy instrument, inspecting the inner-workings of a public intervention.
The typical output (result) indicators used in the evaluation of innovation policy instruments are: the number of patents obtained or applied, number of product or process innovations introduced, employment growth in the supported firms in terms of R&D personnel, etc. However, are they really the primary goals of innovation public policy? Rather not. Innovation policy, as the term suggests, is a policy, a set of instruments, that affect innovation. Innovation can be defined in a broader or narrower manner, however, a key point is that it implies ‘the introduction of new solutions in response to problems, challenges or opportunities that arise in the social and/or economic environment’ ( Edler & Fagerberg, 2017, p.4) From this perspective, the term is applicable to high-tech and low-tech, to manufacturing and services, to the private as well as the public sector (see, e.g. Osborne & Brown, 2013), and, importantly, denotes a qualitative change.
The key concept advanced in this paper is behavioural additionality, which takes account of the difference in behaviour of a target population (firms) owing to a public intervention. Producing the outputs is not only a matter of having the right resources of various types (material, human, capital), this is also a matter of their right combination, coordination in time and space. We can reformulate the above-mentioned examples of output additionality using behavioural lenses: what organisational routines and capabilities have been built/enhanced due to a public intervention, how a public intervention interacts with strategies and capabilities of firms? It can be argued that behavioural additionality is closely related to output additionality, since it creates prerequisites for the improvement of a firm’s economic performance. However, the focus should not be limited to the problem: how public support modifies the manner in which a subsidised project is carried out (the analogy to opening a ‘black box’ of public interventions.). An important question is also about the persistency of the behavioural changes of firms after the intervention (completion of the subsidised project). Moreover, the concept of behavioural additionality captures a change in firm’s orientation (greener or more social focus) as public innovation policy carries horizontal objectives as well.
The paper is organised into five parts. An introduction accentuates the need for complementing (not substituting) the traditional input/output approach to innovation policy evaluation with behavioural additionality effects. The subsequent part deals with the question of how behavioural additionality is conceptualised in extant literature and suggests a common reference frame for evaluation of behavioural additionality at the firm’s level taking into consideration the persistency of policy effects. In the third one – two interrelated lines of inquiry are followed, namely: what are the major obstacles in assessing behavioural additionality effect of public interventions, and how these problems can be overcome. The considerations are based on literature review and evaluation practice. The article ends with concluding remarks and the indication of directions for further research.
A common reference frame for evaluation of behavioural additionality effect at the firm’s level
Behavioural additionality in scholarly literature and evaluation practice is defined in many different ways (for the review – see: Gök & Edler 2011; Kubera, 2018). These can be categorized in the following groups:
- firm-level one-off effects – refer to behavioural changes occurring during a public intervention (e.g., a subsidised project implementation) observed at the firm’s level. They describe situations where public support influences the decision to launch a project (some of the authors refer to this effect as ‘pure additionality’ or ‘project additionality, e.g. Polt & Streicher (2005)); the decision to expand its scope, scale, or the pace in which the project is implemented, as well as other changes in the way supported project is undertaken (e.g. the level of risk involved). In state aid law these effects constitute the so-called “incentive effect’ of aid, (e.g., Falk ,2006; Wanzenböck, Scherngell and Fisher 2013);
- firm-level persistent effects – describe situations where public support influences the firm’s behaviour beyond the frames of the supported project, either by inducing changes in the firm’s strategic management (e.g. integrating technology strategy and R&D strategy into their business strategies), or inducing changes at the operational level (e.g. the application and reporting requirements (greater rigour) are institutionalised into the firm’s further activities); these can relate only to RDI activity or the general conduct of a firm (e.g. Davenport, Grimes and Davies, 1998; Hall and Maffioli, 2008; Clarysse, Wright and Mustar, 2009; Neicu, Teirlinck and Kelchtermans, 2016);
- system-level effects – occur when public support affects behavioural changes of other entities (actors of innovation system), who are not the target population (e.g., knowledge spill-overs, or the so-called hello effect – i.e., when receiving public support influences positively the ability of a firm to attract additional external financing), (e.g., Meuleman and De Maeseneire, 2012; Larrea, Aranguren and Karlsen, 2012; Wu, 2017).
A separate and a very common conceptualisation of behavioural additionality effect which cannot be neatly categorised to one of the three types mentioned above is network additionality (Breschi et al., 2009; Teirlinck & Spithoven, 2012; Cerulli, Gabriele & Poti, 2016). Its prominent role in innovation processes stems from the fact that cooperation with other firms or institutions creates opportunities to gain complementary resources and skills, and as a consequence, can lead to faster development of innovations, improved market access, cost and risk sharing. Network additionality can be viewed as a firm-level one-off effect, when a beneficiary firm enters into collaboration only to carry out the subsidised project, or a firm-level persistent effect (e.g., due to a public intervention, a firm changes the pattern in which it cooperates with others); or system-level effect, in the form of e.g., improved coordination. Moreover, the problem – how changes occurring at the beneficiary firms (target population of a public intervention) modify behaviours of other actors of innovation system is an interesting line of inquiry deserving further investigation (the knowledge spill-over effects, technology diffusion), which are frequently not captured adequately in evaluation of public programmes in the field of RDI (e.g., Autio, Kanninen & Gustafsson 2008).
It follows that present definitions of behavioural additionality effect suffer from conceptual confusion and terminological ambiguity, impending a proper theoretical understanding and hindering empirical research. In order to introduce a common reference frame for the evaluation of behavioural additionality at the firm’s level and incorporating the element of persistency of the changes induced (what is vital from the public policy perspective), in this paper behavioural additionality is conceptualised as a modification of the beneficiary firm’s organisational routines. First, as they are widely acknowledged to be helpful to understand organizational behviour and organizational change. Secondly, as they are the building blocks of the firm’s organizational capabilities (Becker, Lazaric, Nelson and Winter 2005). They can also solve the problem with the confusion between the potential and actual behavior with its ostensive and perfomative dimentions. Research on behavioural additionality effect can be related to one or more of the focal points depicted in Fig.1.

Fig. 1: Focal points in the search for the behavioural additionality of public interventions
Source: Author’s own elaboration
Although all four: organisational routines, organisational capabilities, dynamic capabilities and firm strategy are distinct constructs, they are linked. The term ‘organisational routines” refers to ‘repetitive, recognizable patterns of interdependent actions carried out by multiple actors’ (Pentland and Feldman, 2008, p.235). They can be treated as basic components of organisational behaviour and repositories of organisational capabilities. For that reason, they are relevant for capturing and understanding organisational change. Analysing how organisational routines and capabilities change can help identify the pathways and mechanisms by which internal and external factors affect the organisation’s behaviour (Becker et al., 2005).
Organisational capabilities are larger scale units of analysis in comparison to organisational routines. Following Winter (2003, p.991), Felin et al. (2012) define organisational capability as ‘a high-level routine or collection of routines that together with its implementing input flows, confers upon an organisation’s management a set of decision options for producing significant outputs of a particular type’ (p.1355). Hence, organisational capabilities involve patterned activity which is oriented to relatively specific objectives. They entail deploying resources (inputs), usually in combination, and using organisational processes to produce a firm’s outputs that matter for an organisation’s survival and prosperity. While (operational) capabilities enable an organisation to earn a living at present, dynamic capabilities concern change, as they include: a capacity to identify the need or opportunity for change, to respond to such a need or opportunity and implement a course of action. Hence, they extend, modify or create (ordinary) capabilities (Winter, 2003). They are crucial for developing and maintaining a competitive advantage of the firm and thus have a direct link to the firm’s strategy.
Problems to overcome in behavioural additionality research – putting puzzles together
There are two major hindrances that impede the behavioural additionality research. The first is the confusion between the potential and actual behaviour. The second is called ‘project fallacy’ and denotes ‘the failure to distinguish between a single sponsored project and the longer-term business innovation effort to which it is part’ (Georghiou and Clarysse 2006, p.10).
Hall and Maffioli (2008) while evaluating the impact of technology development funds in Latin America applied subjective indicators – innovation surveys to detect behavioural additionality defined as a more proactive attitude of beneficiary firms towards innovation activities and a firm’s willingness to interact with external sources of knowledge and financing. In a similar vein, Chapman and Hewitt-Dundas (2018) examined the effect of public support on senior managers’ attitudes to innovation, such as support for innovation, risk tolerance and openness to external knowledge. However, we know that potential means that something might chance to happen or not to happen, or being a little more precise, potential is actualised when conditions are right. (Not to mention the common method variance problem involved in self-reporting techniques). On the other hand, though, it should be admitted that numerous public interventions are about changing (forming) attitudes. First, there is an intervention, which causes the attitude change, which, in turn, leads to the behaviour change. It is hard to deny the worth of such studies as they shed some light on the effects of public interventions, but we might have the impression that we have stopped somewhere in the middle, we do not get the full picture of the phenomena under investigation, only a piece of it. Similarly, entering into collaboration, a number of collaborating partners used as indicators of behavioural additionality effect (Aschhoff, Fier & Löhlein, 2006) say little and mean only scale and scope additionality, which can be confused with input/output approach. They create opportunities for spill-over effects, but behavioural change triggers three factors that must be in place simultaneously. Apart from the right conditions (opportunities), there is an emotional factor (motivation) and cognitive factor (knowledge, skills). Public interventions are frequently aimed at only one factor, assuming that the rest are present, what is not always the right approach. It is important for public interventions to yield intended behavioural effects to verify (with a feasible level of confidence) whether these assumptions really hold true.
The recent conceptualisation on organisational routines as generative systems, where the ostensive and performative aspects are distinguished and interlinked, along with artifacts, might be a solution. These three aspects (i.e., ostensive, performative and artifacts) must be in place for a pattern of interdependent actions to be identified as a routine. Public policy interventions produce behavioural additionality effect by creating and/or reinforcing one or more of these aspects.
An ostensive part of a routine refers to the abstract aspect of the routine stored in people’s mind, i.e. an idea, how to act. The performative part is an enactment of the ostensive part in particular time and space. In other words, while the ostensive dimension of a routine is the abstract and generalised understanding of the routine (usually plural, as the understanding of a routine may differ across an organisation), the performative dimension designates a specific action taken by specific people at specific times (Salvato & Rerup, 2011). They are mutually constitutive, with the performances creating and recreating the ostensive aspect and ostensive aspect constraining and enabling the performances (Becker, 2020). A routine does not exist without being enacted, at most we can call it an aspirational or espoused routine.
Artifacts, the third dimension of a routine, can take a variety of different forms, such as written rules, procedures, software and computers or forms of general physical setting (e.g. an outline of an office). They are often used to ensure the reproduction of particular patterns of action. They influence and represent the ostensive and performative dimensions of a routine; however, they cannot be confused with organisational routines as such (Pentland and Feldman, 2008). Standard operating procedures are sometimes used as proxies of routines. While for some questions they may work well, substituting the representations for the routine accentuates the static nature of organisational routines and does not allow capturing their dynamics properly (Feldman, 2016). If we want to get a full picture of behavioural additionality effect of a public intervention, we need to break organisational routines (and capabilities) into parts and map their interrelationships.
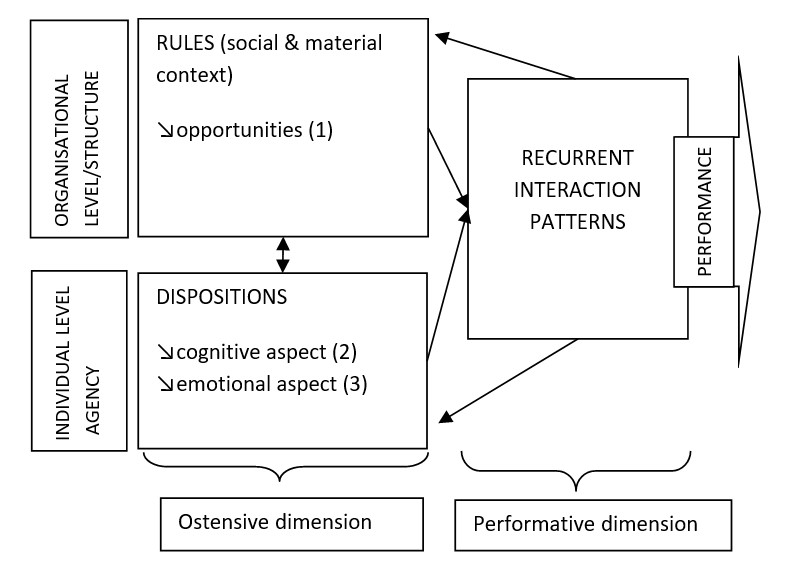
Fig. 2: Internal dynamics of a routine
Source: Author’s own elaboration. (1), (2), (3) – drivers of behaviour change
The next identified hindrance impeding the progress in behavioural additionality research lies in the additionality concept as such. While we are evaluating public interventions, we want to know what was in fact caused by a given intervention not by some other factors. Hence, the focus is on those changes/effects which have been brought about over and above what would have taken place anyway without the examined intervention. Thus, the root problem entails causal explanation. In many situations, it is difficult to separate the effects, in particular behavioural effects, of a subsidised project from the larger ongoing activity of the beneficiary firm (the so-called project fallacy problem).
The prevailing input/output approach in evaluation of public interventions which relies heavily on the successionist model of causation is not suitable for behavioural additionality research. This is because of its inability to capture the contextual features properly. In organisational setting, our actions are conditioned by history and intent (backward- and forward-looking), and power structure, in this sense they are consequential, however, they are also constitutive for organisational structures and processes (they shape the context). Moreover, the successionist approach is unable to explain the causal connection, i.e., the process leading from cause to effect, what happens in-between cause and effect. Generative causation, by contrast, attaches great importance to this transformation, as it tries to provide fine-grained explanation of the behaviour of specific actors (thinking, decision-making, action) in a given context with specific resources, opportunities and constraints (Befani, 2016).
Two approaches are suggested, both of which are grounded in generative causality, to address the problem of ‘project fallacy’, namely: contribution analysis (CA) and process tracing (PT). However, we need to come to terms that they do not measure an impact of a public intervention in a strict sense, but rather increase our confidence that a public intervention had an influence on a target population. In other words, behaviour change might not be caused solely by an intervention (as in the case of attribution). An intervention is one of many factors which contribute to a given change. At best, we can talk about the ‘causal package, in which a given intervention plays a vital role (Mayne, 2012).
CA belongs to theory-based-evaluations, where the starting point is a theory of change, a comprehensive description of a path of how a given intervention is expected to bring about the intended changes. It specifies the activities taken within an intervention and subsequent changes, along with assumptions for each causal link that must hold true for the change to occur and the associated risks as well as other influencing factors. In spite of appearances, this path does not need to be linear, but involves many feedback loops that need to be understood. All these elements of a theory of change are assessed against available empirical evidence. Mayne (2012) developed six key steps in contribution analysis to follow to make the whole approach more systematic.[1]
Moreover, the application of process tracing, its principles and tests, may additionally increase the strength of causal interference made in contribution analysis. PT is a case-based approach to causal inference with a focus on the use of clues within a case (causal-process observations, CPOs) to adjudicate between alternative possible explanations. Miles and Cunningham (2006) write: “The issue at stake is not just a matter of more or less activity at one point in time. Activities have been reshaped, there has been a learning process in the individuals and organisations concerned” (p.160). Of interest are long-term and persistent effects. On the whole, in recent years one can observe that process and practice approaches to organisational topics have gained momentum, ‘de-emphasizing the entity-like features and emphasizing more the continuity of becoming’, ‘dynamic unfolding’ processes. This entails ‘refocusing on enactment rather than representation, on process and potentiality rather than likelihood, and on relationality rather than correlation’ (Feldman 2016, p.26).
The recent incorporation of the informal Bayesian logic into process –tracing allows for assessing the type and strength of inferences we can make using different forms of empirical evidence. Hence, it is not the quantity of observations that affect strength of evidence (unless they are independent), but the probability of observing a given piece of data. The aim is to design data collection so as to maximise our confidence in the existence of the theorised causal mechanisms. If the probability of a given piece of data before and after empirical research is similar, it means that this evidence is weak. Accordingly: the bigger the difference between the two probabilities, the stronger is the evidence (Befani and Mayne, 2014). In what follows, we should assess each piece of potential evidence in terms of its certainty and uniqueness; i.e., whether we have to find a given piece of evidence for the theory to be valid, and whether there are any plausible alternative explanations for finding it. For example, a ‘hoop test’ involves making a prediction with high certainty and low uniqueness, in that there are many plausible alternative explanations for finding the evidence. In this case finding the predicted evidence means little updating of our beliefs, however, not finding this evidence has a disconfirmatory power (Schmitt and Beach, 2015).[2]
Conclusions and directions for future research
In order to ensure policy learning, the traditional input/output approach to evaluation of innovation policy should be complemented with the third type of additionality effect, namely behavioural additionality, which takes account of the difference in behaviour of a target population owing to a public intervention. However, the literature review and evaluation practice reveal that a diverse array of meanings is attached to the term, hindering empirical research and cumulation of knowledge.
Two major hindrances can be identified that impede the behavioural additionality research. The first is the confusion between the potential and actual behaviour, (changing attitudes, our understanding versus changing behaviour, activities undertaken). The second is called ‘project fallacy’ and entails the problem with causal explanation, as in many situations it is difficult to separate the effects of a subsidised project from the larger ongoing activity of a beneficiary firm and this pertains in particular to behavioural effects. Moreover, from the policy perspective, of interest are not only one-off behavioural changes but persistent changes which are not confined to the implementation of a subsidised project.
To remedy the first problem, the definition of behavioural additionality as changes in organisational routines and capabilities is suggested. The recent conceptualisation of organisational routines as generative systems, where the ostensive (abstract and generalized idea how to act) and performative (enactment, real) aspects are distinguished and interlinked, along with artifacts, might help to capture the behavioural changes induced by a public intervention. Common frames and units of analysis can bring greater coherence in behavioural additionality research.
Regarding the second problem, given the complexity surrounding the ways in which firms’ behaviour is modified (context sensitivity, path dependence, power structure etc.), the only viable manner in which we can deal with the problem of ‘project fallacy’ is to strengthen our confidence that a given intervention contributed to an intended behaviour change and that the intervention was a vital part of a ‘causal package’. Contribution analysis by Mayne (2012) combined with process-tracing and its recent developments consisting of the incorporation of informal Bayesian logic are suitable for this purpose.
Nevertheless, there are still many questions concerning the assessment of behavioural additionality effect that remain unanswered and deserve further attention. I would suggest the following directions for future research. First, the concern is about the methods/ research approaches suitable for tracking behavioural effects of public interventions. Case study and ethnographic research is particularly relevant for investigating behavioural additionality, as the main research questions are aimed at ‘how’ and ‘why’ questions, the researcher has little or no control over behavioural events, and the focus of study is a contemporary (as opposed to entirely historical) phenomenon (Yin, 2018). However, the question remains whether can we get beyond the case-study design in the assessment of behavioural additionality effect and still provide rich insights on how firms behave and how the behaviour of firms can be influenced through public policy instruments. Perhaps, modelling approaches could be employed for that purpose to a greater extent. Secondly, behavioural additionality is usually assumed to be positive, that the public intervention will affect the firm’s propensity to innovate so that they can perform better and create competitive advantage, or in the worst case – there is no behavioural additionality, meaning that a public intervention has no persistent impact on the firm’s behaviour. However, too little attention is paid to the case of negative behavioural additionality. Georghiou and Clarysse (2006) mention about negative behavioural additionality providing the example of a public intervention that encourages firms to take risks which they cannot afford. Public interventions are also associated with adverse behavioural effects when they lead to the wrong direction in terms of technology or the market, or encourage firms to enter into alliances which are unproductive and costly. However, defining negative behavioural additionality remains particularly challenging. Even when a subsidised project does not yield desired outputs, we can still talk about positive behavioural additionality when the knowledge and capabilities gained during its implementation turn out to be valuable in subsequent projects, the firm can capitalize on them in other innovation endeavours. Shepherd et al. (2009) describe it aptly: ‘within some failures lie the seeds of subsequent project success’ (p.589). It is argued that not only organisations are more likely to learn from failures than from successes, but also that the knowledge derived from failure depreciates more slowly than when derived from success (Rhaiem and Amara, 2019). Hence, the question is – how can we capture properly these instances of behavioural additionality of public interventions, in particular what time frames should be adopted.
[1] Six steps to be taken to produce a credible contribution story: (1): Set out the cause-effect issue to be addressed; (2) Develop the postulated theory of change and risks to it, including rival explanations; (3) Gather the existing evidence on the theory of change; (4) Assemble and assess the contribution claim, and challenges to it; (5) Seek out additional evidence; (6) Revise and strengthen the contribution story.
[2] The three other tests are: (1) a doubly- decisive test, which combines high uniqueness and high certainty; not finding the evidence downgrades our confidence, while finding it increases our confidence, since there are few plausible alternative explanations for the evidence; (2) a straw-in-the-wind test combines low certainty and low uniqueness which means little updating our beliefs, and (3) a smoking gun test – involves making a theoretically unique prediction but not certain; finding the evidence enables strong confirming inferences, whereas the opposite is not very informative.
(adsbygoogle = window.adsbygoogle || []).push({});
References
- Aschhoff, B., Fier, A. and Löhlein, H. (2006), ‘Detecting Behavioural Additionality. An Empirical Study on the Impact of Public R&D Funding on Firms’ Cooperative Behaviour in Germany’. ZEW Discussion Paper 06-037.
- Astbury, B. and Leeuw, F. (2010), ‘Unpacking Black Boxes: Mechanisms and Theory Building in Evaluation’, Evaluation 31(3), 363-381.
- Autio, E., Kanninen, S. and Gustafsson, R. (2008), ‘First- and second-order additionality and learning outcomes in collaborative R&D programs, Research Policy 37, 59-76.
- Becker, M. (2020), Organisational Routines and Organisational Learning, The Oxford Handbook of Group and Organisational Learning, Argote, L. and Levine, J. (eds.) Oxford University Press.
- Becker, M., Lazaric, N., Nelson, R. and Winter, S. (2005), ‘Applying organisational routines in understanding organisational change’, Industrial and Corporate Change 14(5), 775-791.
- Befani, B. and Mayne, J. (2014), ‘Process-Tracing and Contribution Analysis: A combined approach to generative causal inference for Impact Evaluation’. IDS Bulletin 45(6), 17-36.
- Befani, B. (2016), Causal frameworks for assessing the impact of development programmes. UEA seminar, 9 March 2016
- Breschi, S., Cassi, L, Malerba, F. and Vonortas, N. (2009), ‘Networked research: European policy intervention in ICTs’, Technology Analysis and Strategic Management 21(7), 833-857.
- Cerulli, G., Gabriele, R. and Poti, B., (2016), ‘The role of firm R&D effort and collaboration as mediating drivers of innovation policy effectiveness’, Industry and Innovation 23(5), 426-447.
- Chapman, G. and Hewitt-Dundas, N. (2018), ‘The effect of public support on senior manager attitudes to innovation’. Technovation 69, 28-39.
- Clarysse, B., Wright, M. and Mustar, P. (2009). ‘Behavioural additionality of R&D subsidies: A learning perspective’, Research Policy, 38(10), 1517-1533.
- Davenport, S., Grimes, C. and Davies, J. (1998), ‘Research collaboration and behavioural additionality: A New Zealand case study’, Technology Analysis & Strategic Management 10(1), 55-68.
- Edler, J.and Fagerberg, J. (2017), ‘Innovation policy: what, why, and how’. Oxford Review of Economic Policy, 33 (1), 2–23
- Falk, R. (2006), ‘Measuring the Effects of Public Support Schemes on Firms’ Innovation Activities’, WIFO Working Papers
- Feldman, M. (2016), Routines as Process: Past, Present, and Future, Organisational Routines. How They Are Created, Maintained and Changed, Howard-Grenville, J., Rerup C., Langley A. and Tsoukas H. (eds.), Oxford University Press.
- Felin, T., Foss, N, Heimeriks, K. and Madsen T. (2012), ‘Microfundations of Routines and Capabilities: Individuals, Processes, and Structure’. Journal of Management Studies 49(8), 1351-1374.
- Georghiou, L. and Clarysse, B. (2006). Introduction and Synthesis, OECD. Government R&D Funding and Company Behaviour. Measuring behavioural additionalit,. OECD.
- Gök, A.and Edler, J. (2011). ‘The Use of Behavioural Additionality in Innovation Policy-Making, Research Evaluation 21(4), 306-318.
- Hall, B. and Maffioli, A. (2008), ‘Evaluating the Impact of Technology Development Funds in Emerging Economies: Evidence from Latin America’, The European Journal of Development Research 20(2), 172-198.
- Kubera, P. (2018), ‘Moving beyond the ‘black box’ approach to public interventions promoting research, development and innovation. The concept of behavioural additionality’, Studia i Materiały 2(28), 52-64.
- Larrea, M., Aranguren, M. and Karlsen, J. (2012), New policy approaches to develop innovative territories: Developing trust and behavioral additionality in Gipuzkoa, Innovation, Global Change and Territorial Resilience, Cooke, P., Parrilli M. and Curbelo J. (eds.), Edward Elgar.
- Mayne, J. (2012), ‘Contribution Analysis: Coming of Age?’ Evaluation 18(3), 270-280.
- Meuleman, M. and De Maeseneire, W. (2012), ‘Do R&D subsidies affect SMEs’ access to external financing?’ Research Policy 41, 580-591.
- Miles, I. and Cunningham, P. (2006), SMART INNOVATION: A Practical Guide to Evaluating Innovation Programmes. A Study for DG Enterprise and Industry. ECSC-EC-EAEC Brussels-Luxembourg.
- Neicu, D., Teirlinck, P. and Kelchtermans, S. (2016), ‘Dipping in the policy mix: Do R&D subsidies foster behavioural additionality effects of R&D tax credits’’ Economics of innovation and new technology, 25(3), 218-239.
- Osborne, S. and Brown, L. (eds) (2013). Handbook of Innovation in Public Services, Cheltenham, Edward Elgar
- Pentland, B. and Feldman, M. (2008), ‘Designing routines: On the folly of designing artifacts, while hoping for pattern of actions’, Information and Organization 18, 235-250.
- Polt, W. and Streicher, G.(2005), ‘Trying to capture additionality in Framework Programme 5 – Main findings’, Science and Public Policy 32(5), 367-373.
- Rhaiem, K., and Amara, N. (2019), ‘Learning from innovation failures: a systematic review of the literature and research agenda’, Review of Managerial Science, 1-46.
- Salvato, C. and Rerup, C. (2011), ‘Beyond Collective Entities: Multilevel Research on Organizational Routines and Capabilities’, Journal of Management 37(2), 468-490.
- Schmitt, J. and Beach, D. (2015), ‘The contribution of process tracing to theory-based evaluations of complex aid instruments’, Evaluation 21(4), 429-447.
- Shepherd, D., Covin, J. and Kuratko, D. (2009), Project failure from corporate entrepreneurship: managing the grief process’, Journal of Business Venturing 24(6), 588–600.
- Teirlinck, P. and Spithoven, A. (2012), ‘Fostering industry-science cooperation through public funding: differences between universities and public research centres’, The Journal of Technology Transfer 37(5), 676-695.
- Wanzenbök, I., Scherngell, T. and Fischer, M. (2013), ‘How do firm characteristics affect behavioural additionalities of public R&D subsidies? Evidence for the Austrian transport sector’, Technovation 33, 66-77.
- Winter, S. (2003). ‘Understanding dynamic capabilities’, Strategic Management Journal 24, 991-995.
- Wu, A. (2017), ‘The signal effect of Government R&D Subsidies in China: Does ownership matter?’ Technological Forecasting & Social Change 117, 339-345.
- Yin, R. (2018). Case study research and applications: design and methods. SAGE