Introduction
Substitution age provides more convenience for people around the world with their progress in the realm of technology. Information and Communication Technology (ICT) has brought about fundamental and significant changes in the speed and innovation of education provision in different countries, with several having integrated Information and Communication Technology (ICT) into the planning and implementation of their national education.
Learning management systems (LMS) have been the most popular technology to facilitate e-learning and they are regarded as the most commonly used technology in the field of education.
The learning management systems in this study use a TAM model. TAM includes factor assessors as measures of acceptance of new technologies in learning management systems, such as perceived ease of use (PEOU), perceived usefulness (PU), attitude toward use (ATU) and behavioural intention to use (BIU) a technology.
The study also adopts external variables that affect the acceptance and use of new technologies in learning management systems; for example, computer self-efficacy (CSE), which refers to a person’s judgment of his ability to operate computer applications, together with one’s perception of self-efficacy in performing certain computer-related tasks. It also considers Subjective Norms (SNs); that is, if students feel that people who are important to them believe that they must use LMS, they will tend to do so.
Theoretical Framework
Technology Acceptance Model (TAM)
The Technology Acceptance Model (TAM) is a model of users’ acceptance of information technology systems (Venkatesh, 2003). It was developed by Fred Davis to introduce a theoretical framework based on the theory of reasoned action (TRA). TAM explains the relationship between users and technology to estimate their acceptance of it (Binyamin, Rutter & Smith, 2018).
TAM explains the causal relationship between faith (the benefits of a technology/information systems and consumers’ convenience) and behaviour, as well as the purposes and actual use of technology/information systems (Aditya & Ward, 2018).
According to the TAM model, acceptance of new technologies can be measured by assessing four determinants: perceived ease of use (PEOU); perceived usefulness (PU); attitude toward use (ATU) and behavioral intention to use (BIU). PEOU can be defined as the extent to which a person believes that using LMS requires a minimal cognitive effort, while PU can be defined as the extent of the belief that using LMS will improve one’s performance.
Perceived Usefulness
Perceived usefulness is the extent to which individuals believe that using a technology can help improve the performance of their duties (Gardner & Amoroso, 2004, cited in Kartika, 2009). From these definitions, it can be seen that if people have confidence in their decision-making process, as well as in the technology that brings benefits to the system and is useful for them, then they will use it. Likewise, if a person, who is trusted, does not benefit from it, then they will not use it. Perceived usefulness can be interpreted as a measure of how far individuals believe that the use of a particular technology will improve the performance of their duties (Nursiah, 2017).
Venkatesh et al. (2003) state that there is an important influence of understanding the response of individuals’ benefits in information technology. The same notion is expressed by Shun Wang et al. (2003, cited in Luh Putu, 2013), that the perception of the usefulness is when a person believes that using a system will improve his performance. According to Ward (2018), usefulness is people’s level of confidence that the use of a special system will improve the performance of their jobs. Perceived usefulness is defined as the usefulness of a technology, so that when power to a technology, this will not show the intention of a person to use it.
Based on the above explanations, it can be concluded that perceived usefulness is the extent to which a person believes that using a technology will increase productivity and performance, and will constitute benefits of acceptance of information technology systems. If someone believes in the benefits of an information technology system, he will use it and make a positive contribution
This concept describes the extent to which the use of a technology is believed to bring benefits to the user. The concept of perceived benefits can be grouped into two categories, namely:
- Perceived usefulness to the estimation of the factors, the indicators are:
- Makes the job easier.
- Is helpful (useful).
- Increases productivity.
- Enhances effectivity.
- Improves job performance.
- Perceived usefulness with two opinions, the indicators are:
- Expediency, covering dimensional work easily , is useful and adds to productivity.
- Effectiveness, encompassing the dimensions, enhancing effectiveness and improving performance (Nursiah, 2017).
Perceived Ease of Use
Perceived ease of use is defined as the extent to which prospective users expect an easy implementation of the target system. In other words, prospective users do not expect great difficulty in learning and implementing the use of technologies (Chuttur, 2009). Ease of use is defined as the individual’s perception that the use of a new technology will be free of difficulties or great efforts (Johar & Awalluddin, 2011).
Studies show that PEOU is positively related to the intention to continue in the context of web-based learning (Hamida, 2016). Perceived ease of use is specified as the extent to which potential users expected an easy implementation of the target system. In other words, users do not want to face any difficulties in asking for and using a technology (Chuttur 2009; Surendran, 2013). Perceived ease of use affects usability constructs, attitudes, intentions and use of technology. However, the most significant aspect is the effect on constructing usability, while for the other construct, the effect is not significant (Jogiyanto 2008, p. 115).
Perceived ease of use is defined as the extent to which a person believes that the use of a technology is easy so this person will intend to use that technology. Ease means free from difficulties without having to try hard. From the above statement, it can be concluded that the definition of perceived ease of use is when someone has confidence in the information technology system used and does not face difficulties in operation or require a particular effort to use it .
In other words, the term refers to a person’s level of confidence that the use of information systems is easy and requires no effort. This simplicity will reduce the energy, thought and time spent on learning and using the systems. People who work with information systems work more easily than people who work manually without them.
The indicators in a study conducted by Nursiah (2017) for measuring perceived ease of use are as follows:
- Ease of Studies.
- Work on the desired job with ease.
- Easy to increase user skills.
- Easy to use/operate.
Behavioural Intention to Use
The use of information systems has long hypothesized the existence of behavior intention to use technology. Because behavioural intention is estimated by the attitude toward the action, it is reasonable to predict that a good attitude will lead to a good behaviour. The intention to use a behaviour is also a good predictor for determining the actual use (actual system usage). The behavioural intention to use an information technology is a behavioural tendency to continue its use (Nursiah, 2017)
Behavioural intention to use shows the desire of individuals to reuse the same thing whenever needed (Taylor & Baker, 1994 and Kartika, 2009). The behavioral intention to use is the desire of individuals to reuse the same thing whenever needed (Taylor & Todd, 1995); in other words, a person’s subjective probability of performing a behaviour or one associated with a particular object. Behavioural intention is a measure of the likelihood of someone using e-learning (Surendran, 2012), and is also defined as a person’s subjective probability of performing a certain behaviour (Ducey, 2013).
It can be concluded that the behavioural intention to use is a form of behavioural intention to the trend of using a new system that is applied in the organization. The interest in using the system is an interest in a person as a target of an application system that is implemented. Interest and willingness emerge from an individual’s intention to apply a system, and influence and motivate other users.
Bala (2008) uses multiple measurements of interest/intent, with the following indicators:
- The desire to use.
- Always try to use.
- Sustainable use in the future.
Computer Self-Efficacy (CSE)
The variable which is most widely used to expand TAM in the field of e-learning is computer self-efficacy (CSE). This factor was introduced as a determinant of PEOU by Venkatesh and Davis in 1996 (Benjamin et al. 2018). It measures a person’s upper estimate of his ability to use computer technology. Therefore, if someone feels that he has a great ability in using computer technology, he will be more likely to use the system. For the purposes of this study, the CSE is intended to estimate students’ confidence about their abilities to use the LMS provided by their institution.
Derived from the theory of self-efficacy, CSE shows that a person’s ability to use a computer, affects his perception of ease of use and technology acceptance decisions (Gong, Xu &Yu, 2004). An individual with a strong sense of CSE will tend to be frustrated by technical constraints; therefore, he will be diligent in his efforts to overcome the difficulties and show a greater intention to use technology than those whose CSE is insufficient.
According to Kulviwat et al., cited in Kartika (2009), self-efficacy is the assessment of a person of his ability to organize and decide on the actions required to achieve the desired performance. Bandura (1986), cited in Todd (1995), defines confidence (self-efficacy) as people’s consideration of their ability to organize and carry out a set of activities required to achieve the planned performance.
Computer self-efficacy refers to the rate at which individuals believe that they have the ability to perform specific job tasks or use a computer. It is not related to what a person has done in the past, but it is an assessment of what can be done in the future (Compeau & Higgins, 1995); that is, computer self-efficacy is a belief or confidence in the capabilities and expertise of people to use computers in order to perform tasks using information technology.
Indicators used in computer self-efficacy are magnitude, strength and generalizability (Compeau & Higgins, 1995). Magnitude refers to a person’s level of capability of the correct use of computers, while strength refers to the level of confidence in the ability of the individual to perform computerized tasks. Generalizability refers to the ability to use software packages and systems that are different to those used by individuals with a lower level of generalizability.
Subjective Norm
The perceptions of making decisions about social influences to engage or not in certain behaviours are known as Subjective Norms. The theory of planned behaviour posits is that when decision-makers feel that other people are important in approving or suggesting certain behaviours, they are more likely to engage in these behaviours (Prime, Hasan & Apostolic, 2018).
Researchers use the term social influence as an alternative to subjective norm. Subjective norm shows the extent to which individuals feel that others think they should or should not display certain behaviours. In the study (Benjamin et al. 2018), if students feel that the people who are important to them believe that they should use an LMS, they are more likely to do so. This proves that the subjective norm affects the use of technology among students, especially in developing countries.
Fishbein and Icek (1975) argue that “the subjective norm is the person’s perception that most people who are important to him think he should or should not perform the behavior in question”.
Linan (2008) explains that Subjective Norm or social norms are the individual’s perception of the social pressure from family, friends or the people close to him regarding his decision to display an entrepreneurial behaviour. Based on the above theory, it can be concluded that Subjective Norm is about one’s understanding of the decision to do something based on confidence and supported by an internal and external motivation. Subjective Norm is obtained from the individuals of the surrounding environment, of confidence and motivation of individuals to follow this norm. Fishbein found that subjective norm is generally determined by two determinants as well be an indicator, as follows:
- Perceptions or beliefs about the expectations of certain individuals or groups against a person is the reference to do the behavior or not (normative beliefs)
- Motivation of individuals to meet these expectations (motivation to comply).
Hypotheses
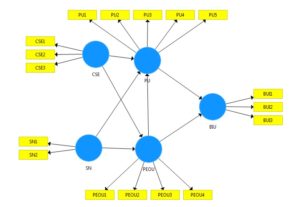
Figure 2: Research Model
Source: processed data
H1: CSE has a positive influence on PU in the use of learning management systems.
H2: CSE has a positive influence on PEOU in the use of learning management systems.
H3: SN has a positive effect on PU in the use of learning management systems.
H4: SN has a positive effect on PEOU in the use of learning management systems.
H5: PEOU has a positive effect on PU in the use of learning management systems.
H6: PU has a positive influence on BIU.
H7: PEOU has a positive effect on BIU.
Research Methodology
Based on the problems formulated by researchers, the purpose of this study is to analyse the influence of computer self efficacy, subjective norm, perceived usefulness and perceived ease of use on the use of learning management systems. The survey method is employed to obtain data and follow the facts directly from the source using a comparative approach. A quantitative approach is also used, in which all the data are implemented in the form of numbers, which are then analysed to generate value statistics. The data used are primary data for the independent variables (computer self efficacy and subjective norm) and the dependent variables (perceived usefulness, perceived ease of use and behavioural intention to use).
Operational Variables
Variables are defined as differentiators, or they create variations in value. Values can be different in the period to an object or for the same person, or in the same period for different objects (kartika, 2010). The operational functions of the variables in this study are divided into two, namely independent and dependent variables.
- According to Sugiyono (2014), an independent variable is a variable that affects, or is the cause of, a change in or the emergence of a dependent variable. In this study, the independent variables are computer self efficacy and subjective norm.
- According to Sugiyono (2014), a dependent variable is a variable that is affected by, or becomes due for, their independent variables. In this study, the dependent variables are perceived usefulness, perceived ease of use and behavioural intention to use.
Table 1: Operational Variables
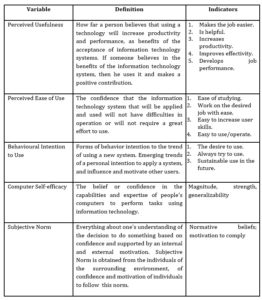
Results And Discussion
An indicator is said to be valid if it has a loading factor above 0.5 against the intended constructs. Output SmartPLS for loading factors provides the following results:
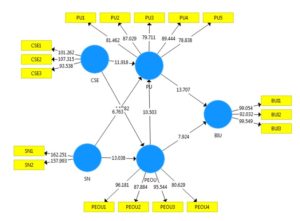
Figure 3: SmartPLS output results
Source: processed data
All the indicators have loading factor values above 0.5 (Hair Jr et al. 2016), so it can be said that the indicators used in this study are valid or have met the convergence validity. Furthermore, the reflective indicators also needed to be tested by a cross loading discriminant validity, with the following results:
Table 3: Loading Factor
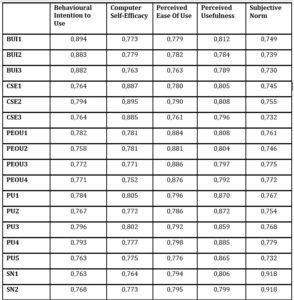
Source: processed data
An indicator is said to be valid if it contains the highest loading factor of the targeted construct loading factors compared to other constructs. A discriminant validity evaluation was conducted in two stages; obtaining the value of cross loadings and comparing the value of the square of the correlation and the AVE construct value or construct correlation with AVE roots. The criterion in the cross loading, that each indicator that measures must be correlated, is higher with its construct compared to other constructs. The cross loading output is shown in Table 3, where it can be seen that the loading factor of each indicator for the construct is higher than other constructs. Another method to perform the discriminant validity is to observe the value of the square root of the average variance extracted (AVE) to the recommended values above 0.5. Table 4 shows the study of AVE values:
Table 4: Reliability and Validity
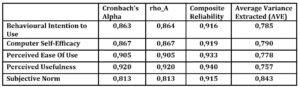
Source: processed data
Table 4 shows that the AVE value of all the variables is above 0.5, with the lowest value being 0.757 for the perceived usefulness construct. Reliability testing was conducted by obtaining the value of the block of composite reliability indicators measuring the construct. Composite reliability results are quite reliable if their value is above 0.3, but these will be more satisfactory if they are above 0.7. Furthermore, the reliability testing may be confirmed by Cronbach’s Alpha; the resulting output has values above 0.3, so they are reliable. In this study, all the constructs have Cronbach’s alpha and composite reliability values above 0.7, meaning they can be used reliably.
Hypothesis Test
Table 5: Path Coefficient (Mean, STDEV, T-Values, P-Values)
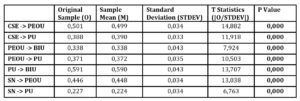
Source: processed data
The influence of CSE on PU is significant, with a t-statistic of 11.918 (> 1.96). The original value estimate was a positive sample of 0.388, which indicates that the direction of the relationship between CSE and PU is positive. Therefore, the first hypothesis (H1) states that computer self efficacy (CSE) has a positive and significant effect on perceived usefulness (PU).
The value of the t-statistic variable of the influence of CSE on PEOU is 14.882 (>1.96). The original value estimate was a positive sample of 0.501, which indicates that the direction of the relationship between CSE and PEOU is positive. Therefore, the second hypothesis (H2) states that computer self efficacy (CSE) has a positive and significant effect on perceived ease of use (PEOU).
The influence of SN on PU is significant, with a t-statistic value of 6.763 (>1.96). The original value estimate was a positive sample of 0.227, which indicates that the direction of the relationship between SN and PU is positive. Therefore, the third hypothesis (H3) states that subjective norm (SN) has a positive and significant effect on perceived usefulness (PU).
The influence of SN on PEOU is significant, with a t-statistic value of 13.038 (>1.96). The original value estimate was a positive sample of 0.446, which indicates that the direction of the relationship between SN and PEOU is positive. Therefore, the fourth hypothesis (H4) states that subjective norm (SN) has a positive and significant effect on perceived ease of use (PEOU). This result is in line with the research of Binyamin et al. (2018), which found that computer self-efficacy and subjective norm are two necessary factors that influence students’ use of LMS, contributing to their academic achievement and performance.
Table 5 shows that the influence of PEOU on PU is significant, with a t-statistic value of 10.503 (<1.96). This is in line with the estimated value of the original sample of 0.371, which indicates that the direction of the relationship between PEOU and PU is positive. Therefore, the fifth hypothesis (H5) states that perceived ease of use (PEOU) has a positive and significant effect on perceived usefulness (PU).
The influence of PU on BIU is significant, with a t-statistic value of 13.707 (>1.96). The original value estimate was a positive sample of 0.591, which indicates that the direction of the relationship between PU and BIU is positive. Therefore, the sixth hypothesis (H6) states that perceived usefulness (PU) has a positive and significant effect on behavioural intention to use (BIU).
Based on the structural test model, the effect of PEOU on BIU is significant, because it has a t-statistic value of 7.924 (> 1.96). The original value estimate was a positive sample of 0.338, which shows that the direction of the relationship between PEOU and BIU is positive. Therefore, the seventh hypothesis (H7) states that there is a positive and significant impact of perceived ease of use (PEOU) on behavioural intention to use (BIU). These results are the same as those of a study by Aditya & Wardhana (2018), which found that perceived usefulness ranked highest; perceived ease of use and behavioural intention to use were in the next category; perceived usefulness had a significant effect on behavioural intention; perceived ease of use significantly influenced behavioural intention; and perceived usefulness and perceived ease of use significantly influenced behavioural intention.
Based on the above discussion, computer self efficacy and subjective norm affect perceived usefulness and perceived ease of use. It is also found that perceived usefulness and perceived ease of use affect behavioural intention to use, especially with regard to technology in teaching and learning. The research shows that each indicator has a positive and significant influence on the other indicators, as evidenced by the t-statistic values and the original values of each hypothesis being positive.
Conclusion
- Computer self efficacy positively influences perceived usefulness, with a significance level of 918 and a positive direction of their relationship.
- Computer self efficacy positively influences perceived ease of use, with a significance level of 882 and a positive direction of the relationship between them.
- Subjective Norm positively influence perceived usefulness, with a significance level of 763 and a positive direction of their relationship.
- Subjective Norm positively influence perceived ease of use, with a significance level of 038 and a positive direction of the relationship between them.
- Perceived ease of use positively influences perceived usefulness, with a significance level of 503 and a positive relationship between them..
- Perceived usefulness positively influences the behavioural intention to use, with a significance level of 707 and a positive direction of their relationship.
- Perceived ease of use positively influences the behavioural intention to use, with a significance level of 924 and a postive direction of the relationship between them.
Suggestions
The research has explained that computer self efficacy and Subjective Norm affect perceived usefulness and perceived ease of use. It has also been found that perceived usefulness and perceived ease of use affect the behavioural intention to use, especially with regard to technology in teaching and learning. Each indicator has a positive and significant influence on the other indicators, as evidenced by the t-statistic and original values of each hypothesis being positive.
At present, the use of technology is common in various fields, including the educational domain, as it is believed to be able to help improve one’s confidence and expertise in matters of learning. For this reason, technology-based learning should be applied in teaching and learning activities. One determining factor for people to be able to hone their ability to apply technology well in learning activities is the perception of one’s own beliefs, the perceived usefulness of the technology, perceptions of the ease of use of technology and the intention to use technology. For this perception, computer self-efficacy and Subjective Norm can also be used as external factors regarding the use of technology for teaching and learning activities.
(adsbygoogle = window.adsbygoogle || []).push({});
References
- Aditya, R., & Wardhana, A. (2018). Pengaruh Perceived Usefulness Dan Perceived Ease Of Use Terhadap Behavioral Intention Dengan Pendekatan Technology Acceptance Model (Tam) Pada Pengguna Instant Messaging Line Di Indonesia, (August). https://doi.org/10.20885/jsb.vol20.iss1.art3 put English translation of title in brackets after the original
- Binyamin, S. S., Rutter, M. J., & Smith, S. (2018). The Influence of Computer Self-efficacy and Subjective Norm on the Students Use of Learning Management Systems at King Abdulaziz University, journal details missing (October). https://doi.org/10.18178/ijiet.2018.8.10.1124
- Chuttur, M. (2009). Overview of the Technology Acceptance Model : Origins , Developments and Future Directions. incomplete
- Compeau, D. R., & Higgins, C. A. (1995). Development of a Measure and Initial Test, journal name?19(2), 189–211.
- Ducey, A. J. (2013). Predicting Tablet Computer Use : An Extended Technology Acceptance Model. incomplete
- Fishbein, & Icek, A. (1975). Belief, Attitude, Intention, and Behavior. Philipines put city: Addison-Wesley.
- Hair Jr, J. F., Hult, G. T. M., Ringle, C., & Sarstedt, M. (2016). A primer on partial least squares structural equation modeling (PLS-SEM). city Sage Publications.
- Johar, M. G. M., & Awalluddin, J. A. A. (2011). THE ROLE OF TECHNOLOGY ACCEPTANCE MODEL IN EXPLAINING EFFECT ON E – COMMERCE don’t use capitals, journal name 3(3). page numbers
- Kartika, S. E. (2009). Analisis Proses Penerimaan Sistem Informasi Icons Dengan Menggunakan Technology Acceptance Model Pada Karyawan Pt.Bank Negara Indonesia (Persero) Tbk. Di Kota Semarang. see comment on first reference
- Linan, F. (2008). Skill and value perceptions : how do they affect entrepreneurial intentions ? journal name, volume/issue missing, 257–272. https://doi.org/10.1007/s11365-008-0093-0
- Nursiah, N. (2017). PENGARUH PERCEIVED EASE OF USE DAN PERCEIVED USEFULNESS TERHADAP BEHAVIOR INTENTION TO USE don’t use capitals – translation – journal name – page numbers all missing, 3(2).
- Perdana, A. A., Hasan, A., & Rasuli, M. (2018). Pengaruh Sikap , Norma Subyektif , Persepsi Kontrol Perilaku dan Etika terhadap Whistleblowing Intention dan Perilaku Whistleblowing ( Studi Empiris di BPKP Perwakilan Riau dan Sumatera Barat ) translation, 11(1), 89–98.
- Surendran, P. (2012). Technology Acceptance Model : A Survey of Literature, journal name2, 175–178.
- Taylor, S., & Todd, P. (1995). Assessing IT usage: The role of prior experience. MIS Quarterly, 561–570.
- Venkatesh, V., Morris, M. G., Davis, G. B., & Davis, F. D. (2003). USER ACCEPTANCE OF INFORMATION TECHNOLOGY : TOWARD A UNIFIED VIEW no capitals – journal name 1, 27(3), 425–478.