Introduction
In the current context, economic development and society are increasingly concerned with optimizing and streamlining resources. For organizations, globalization brings the pressure of demanding customers, of a more complex, dynamic business environment, in which obtaining the competitive advantage and surpassing the competitors is a vital objective for survival. Therefore, more and more of these organizations are intensely concerned with improving processes.
A process is a sequence of steps, which have a beginning and an end, performed to achieve a proposed goal. Starting from this definition, a business process is a logical sequence of connected activities that transforms and transports goods and/or information within the organization, to the client/beneficiary. (Dumas, et al., 2012)
To improve processes, organizations must have some information about both the external and internal environment. It is very important for the organization to know the industry in which it operates, the standards and key success factors of the industry, and of course, to be able to identify, analyze, configure, monitor and control internal processes. Accordingly, it is necessary to classify industries and organizations.
In its simplest form, classification is merely defined as the ordering of entities into groups or classes on the basis of their similarity. (Bailey, 1994)
Therefore, in terms of classification of industries (i.e. taxonomy), this involves grouping companies according to certain similarities or criteria, which differ from one classification to another, such as: raw material used (agro-based industries, mineral based industries, marine-based industries, forest-based industries), size – by how much money is invested and level of technology (small-scale industries and large-scale industries), ownership (private sector, public sector, joint sector industries or cooperative sector industries).
The organizations that operate in these industries can be grouped according to ownership (private, public joint), size (small, medium, large, giant), area of operation (local, regional, national, international), the nature of the behavior of the members of the organization (voluntary associations, military organizations, philanthropic organizations and corporations), etc. (Iacob & Cismaru, 2012)
Classification systems are critical for obtaining accurate measures of economic activity, providing information about the type of goods and services produced in an economy, the kind of jobs available, organizations’ comparative advantage, etc.
The aim of this paper is to examine the literature in the field of management processes and process management, under the scope of maturity models and also to investigate the concept of maturity of management processes and maturity of process management from the perspective of a different approach in the literature. The work is organized as follows. After the introduction, in the fundamental knowledge and related work, we deepen three important pillars for the work: process management, management processes, and maturity models. In the next section, we describe the main goal. In order to achieve our results, we present the research methodology, data and processing. The results of our research are presented and summarized in the Results section. The last section discusses and concludes the main findings.
Fundamental knowledge and related work
In the following, we introduce the concepts that will be later used in the research. We present the theory behind process management, management processes and maturity models and highlight relevant related work that has tackled these concepts.
Process Management
Process management is an integral management concept to guide, organize and manage a company (Meerkamm, 2010). Introduced by Deming in 1986 as a central element of quality management, process management was quickly popularized, being recognized in the late 90’s as BPM. Therefore, it must be established that in the scope of this article, process management refers to Business Process Management (BPM).
BPM is “the art and science of overseeing how work is performed in an organization to ensure consistent outcomes and to take advantage of improvement opportunities” (Dumas, et al., 2018). A business process represents the “specific ordering of work activities across time and space, with a beginning, an end, and clearly identified inputs and outputs.” (Davenport, 1993).
BPM combines knowledge from information technology and management sciences (van der Aalst, 2013). It is typically structured along lifecycle models (as shown in figure 1) assuming that each business process improves through iteration (Macedo de Morais, et al., 2014). These life cycle phases include activities such as process identification, discovery, analysis, improvement, implementation, monitoring and control (Dumas, et al., 2018). Business process management (BPM) receives constant attention from industry (Dumas, et al., 2018) (van der Aalst, 2013).
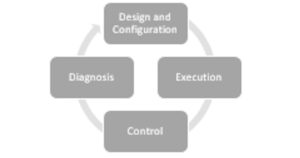
Fig. 1. Business Process Management (BPM) (van der Aalst, et al., 2007)
According to Rosemann, M., & Brocke, as a management discipline, BPM involves two ways of development:
- Process improvement: Initial studies in this area focused on the analysis of existing organizational processes (‘as-is’ state), in order to improve these processes continuously or incrementally. These approaches include Total Quality Management (TQM), Lean Management, Six Sigma, etc.
- Process reengineering: introduced by Hammer (1993), this approach deals with existing organizational processes in terms of efficiency and quality that it creates (added value). This method involves redesigning the whole process, by radically improving it and using information technology as the main component.
Given the diversity of industries, organizations and their processes, the scope of BPM is quite wide, both among organizations and within one organization (Chatman & Jehn, 1994) (Trkman, 2010) (Hammer, 2015) (Harmon, 2015).
In addition, BPM is applied today to different purposes, with a trend from the exploitative to the exploratory capabilities of BPM (Benner & Tushman, 2003) (Rosemann M., 2014). While BPM has traditionally focused on increasing the efficiency and effectiveness of business processes through standardization or automation (exploitation), it also offers opportunities for innovation (exploration) (Brocke & Schmiedel, 2015).
In recent years, several research fields in BPM have emerged: automated service selection and composition (Heinrich, et al., 2018) (Hoffman, et al., 2012), automated planning of process models (Lemos, et al., 2016) (Xu, et al., 2016) and process mining (van der Aalst, 2016) (van der Aalst, et al., 2012).
Management Processes
Generally, in the activity of any organization there are two types of processes: execution processes and management processes.
- Execution processes – are those through which the product/service for the customer is actually made. They are characterized by the fact that the labor force acts directly or indirectly (with the help of equipment, utilities, software systems, etc.) on labor objects, resulting in obtaining products or services, corresponding to the nature of the production processes involved and the objectives set.
- Management processes are generally characterized by the fact that part of the existing workforce in the unit (e.g., C-suite) acts on the other majority party, in order to attract it in an organized way to achieve the established objectives.
In this context, the management process represents a set of interventions through which the manager exercises the management functions. (Mironescu, 2013)
Management processes were first identified and analyzed by Henry Fayol (1916), who proposed five main functions: (i) forecasting and planning, (ii) organizing, (iii) commanding, (iv) coordinating and (v) controlling.
The dynamics of organizations and the business environment lead to numerous classifications of processes depending on their type (management, operational/execution, support), hierarchy (strategic, tactical, operational), their complexity (simple/static, generic/hybrid, complex /dynamic) etc. Although the opinions of practitioners and researchers seem to differ when it comes to the classification of business processes, in terms of management processes there is some consensus on (a) their concern for the future performance of the organization and on (b) the fact that they are super-ordinate to the other categories of business processes. (Bititci, et al., 2011)
Table 1. Classification of business processes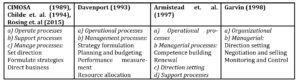
Source: Author’s Own Work
Table 1 shows some proposed classification of business processes with emphasis on management processes. Considering the information presented, it can be seen that the management processes cover a wide area, being dispersed throughout the organization, exceeding the functional boundaries (or departments).
Given the purpose of this paper, management processes are considered to be strategic business processes, which aim to optimize the use of human, financial, material and information resources, in order to achieve the organization’s objectives in the most efficient way. Based on the literature reviewed, we elected to proceed with the following definition:
“The management process consists of all the phases that determine the objectives of the organization, the resources and work processes necessary to achieve their objectives and executors, which
controls and integrates the work of staff using specific methods and techniques.” (Nicolescu & Verboncu, 1999)
In conclusion, management processes are vital to an organization, strongly influencing its performance.
Maturity Models
Maturity, as a degree of measurement, is used to assess the capability of an organization, regarding different dimensions (projects, people, policies and procedures, technology, etc.). The concept became very popular with the advent of the Capability Maturity Model (CMM), proposed by the Software Engineering Institute at Carnegie Mellon University (1986), to evaluate the software development process.
The basic principle of a maturity model (MM) is to describe maturity stages across different relevant domains (Röglinger, et al., 2012). Usually, MMs are “multi-stage models that describe typical patterns in the development of organizational capabilities” (Comuzzi & Patel, 2016). The concept of maturity stages building on each other in order to offer an effective tool for analysis and measurement has its roots in Crosby’s quality management process maturity grid (1979). (Wendler, 2012)
Despite the increasing number of publications about maturity-related topics, a clear definition of the term ‘maturity model’ is often avoided, most of these publications describing the purpose and functioning of the models.
In the Oxford English Dictionary, ‘maturity’ is described as “The state of being mature; fullness or perfection of development or growth”.
In the context of maturity and assessment models, a broad definition of maturity can be adopted, including both behavior and capability. According to Andersen & Jessen, the concept of maturity in the business community is best explained as the sum of action (ability to act and decide), attitude (desire to be involved), and knowledge (an understanding of the impact of availability and action).
Thus, from the point of view of these definitions, it can be stipulated that the objective of maturity models is to present stages for the measured elements (e.g., software systems capability) in accordance with their scope, having as reference their “state of being mature”. Therefore, they constitute a quantitative evaluation of qualitative features.
For each maturity level, the MM describes corresponding stages for relevant domains. These stages should be logically connected and generalizable to identify the correct maturity level of an organization. Röglinger, Pöppelbuß, & Becker (2012) distinguish three application-specific purposes of MMs:
- The descriptive purpose: MMs are used as a diagnostic tool in order to assess the current maturity state of an organization (‘as-is’ state).
- The prescriptive purpose: MMs outline the desirable state (high maturity) and specify a development path for organizations to achieve this state (‘to-be’ state).
- The comparative purpose: MM allows to compare different organizations through the same assessment tool.
Other maturity models were developed, and their approaches are presented in table 2.
Table 2. Overview of the different concepts of the maturity models (Fraser, et al., 2002) (Broens, et al., 2007) (Rosing, et al., 2015)
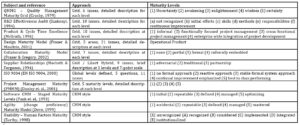
Maturity models describe and determine the state of perfection (fluffiness, completeness – maturity) of certain capabilities. As it can be seen in table 2, the application of this concept is not limited to a specific domain. The basic purpose of maturity models is to define simplified stages / levels of maturity, including the characteristics of each stage, through different sets of criteria.
Maturity of Process Management
Over a hundred maturity models have been developed in various fields, most of which are inspired by the Capability Maturity Model (CMM). Also, in business process management (BPM) an array of maturity models has been suggested (Hammer 2007, Lee et al. 2007, Rosemann and de Bruin 2005, Weber et al. 2008). In the BPM field, two types of maturity models can be identified: process maturity models and BPM maturity models. The former refers to the state of the processes in general or to distinct types of processes, the latter to the BPM capabilities of a company.
However, the overall structure of maturity levels is similar. Most of them define a framework with 5 levels (from 1 – initial & uncertainty up to 5 – optimized & continuous improvement) and generally distinguish the following 6 areas (Rosing, et al., 2015): strategic alignment, governance, methods, information technology, people and culture.
Once an organization has identified the level of maturity at which it is located, depending on the purpose of the chosen maturity model, the areas where improvements are needed can be identified, a set of steps to reach a higher level can be followed or the positioning of a dimension can be analyzed in relation to other dimensions, either internal or external.
Maturity of Management Processes
When discussing the maturation of management processes, the diversity of industries and the dynamics of organizations have led to the creation of maturity models specific to a particular field. Thus, one can distinguish in literature, among others, different maturity models developed for analytics (Big data maturity models – i.e., TDWI MM, Van Veenstra’s model), change management (e.g., Prosci’s Change Management MM), human resources (People Capability Maturity Model – PCMM), project management (e.g., Organizational Project Management Maturity Model – OPM3, Portfolio, Program and Project Management MM – P3M3).
Hence, when searching for management process MM, it can be seen that management has no standards by which to assess the maturity of their business ‘management processes’ alone.
All these developed models concern the management processes from the perspective of the development level of certain capabilities/core elements (i.e., the six core elements of BPM proposed by Rosemann and Brocke, 2010). However, no maturity model evaluates the flow of a management process (i.e., the process of developing vision and strategy, defining the business concept and long-term vision, conducting organization restructuring opportunities, etc.).
Maturity of Process Management versus Maturity of Management Processes
Considering the information presented in our study on the two concepts, ‘maturity of process management’ and ‘maturity of management processes’, we noticed that oftentimes they are not explicitly approached. In general, when talking about the maturity of the organization, most models define maturity from the perspective of BPM. Although the maturity of process management often involves the evaluation of processes at the organizational level, from the perspective of human resources, IT systems, portfolio, etc., it does not explicitly include management processes, and the maturity of management processes seems to be an area that does not receive a lot of attention. Thus, although the two concepts are linked, discussing organizations and related processes, they seem to be generally approached in different areas.
Therefore, we state the following hypothesis:
Research hypothesis: Although the maturity of management processes and the maturity of process management seem to be two similar concepts, in literature they address different topics.
Methodology
In order to test our hypothesis, we have decided to conduct quantitative analysis. Due to the growing adoption of Machine Learning (ML) techniques in all areas of research, not just Artificial Intelligence (AI) fields, we have decided to implement Clustering, a type of ML algorithm, in order to process multiple research papers and extract latent knowledge that can confirm or deny our premise.
Data
We built the dataset, because we couldn’t find one that would suit our needs. Therefore, the data come from primary sources. The data were collected from Elsevier, Science Direct. The articles were searched by 6 keywords: management processes (MP), process management (PM), process management & management processes (PM&MP), maturity model (MM), process management maturity (PMM) and management processes maturity (MPM). Further filters were applied:
- Publishing Year: 2015 – 2021,
- Article type: Research article and
- Subject areas: Business, Management and Accounting.
The dataset consists of 2,661 observations, containing metadata about research articles. 2015 was chosen as the starting year of the studied period from the perspective of the novelty of the research, this analysis preferring more recent work.
The attributes by which the articles are indexed are: Article Title, Searched Keyword, No. Citations, Publishing Year, Abstract and Keywords.
Preprocessing
We clustered the articles by the context of their abstract. Therefore, Natural Language Processing (NLP) concepts must be implemented. NLP is an area of AI that focuses mainly on tackling written and spoken language. The NLP technique that will be used is Tokenization.
Tokenization is the process of transforming a text into a vector, in order for it to be processed by AI algorithms.
The initial dataset consisting of 2,661 articles was reduced to 2,604, because 57 articles did not have an abstract. The dataset was further trimmed to 2,571 articles, because 33 articles weren’t written in English.

The tokenizing technique used is Term Frequency – Inverse Document Frequency (TFIDF). This technique was first introduced in Information Retrieval (IR) and is a Bag – of – Words approach, meaning it doesn’t account for the order in which the words appear.
(1) TFIDF weighs the number of term-occurrences c(w) in a certain document against the occurrences of that term within the corpus of all documents d(w), relative to the total number of documents D. It is a good indicator for the relevance of a term (Aggarwal & Zhai, 2012).
We decided that this tokenization method is sufficient for our preliminary analysis and more complex techniques, such as word2vec, are not necessary.
Before applying TFIDF vectorization, we removed stop words and punctuation from the corpus. Stop words add no value to the information gain, such as “the”, “a”, “an” etc.
Clustering
We used k-means++ as our clustering algorithm because it offers excellent time complexity and good cluster purity (Rodriguez, et al., 2019). k-means++ is an improvement of the k-means algorithm (Arthur & Vassilvitskii, 2007), which aims to partition n observations into k clusters in which each observation belongs to the cluster with the nearest mean (cluster centers or cluster centroid). k-means clustering minimizes within-cluster variances (squared Euclidean distances) (Jain, 2010).
k-means++ is an algorithm for choosing the initial values (or “seeds”) for the k-means clustering algorithm. k-means++ specifies a procedure to initialize the cluster centers before proceeding with the standard k-means optimization iterations.
Because k-means++ is an algorithm that partitions the data in a given number of clusters, a technique to determine the best number is required. We use a common approach, the elbow method.
The elbow method is a graphic tool. It measures the within-cluster distance for an interval of clusters, in our case from 2 to 10, and plots it on a graph. The point at which the slope plateaus is the optimal number of clusters. The distance function used is squared Euclidean distance.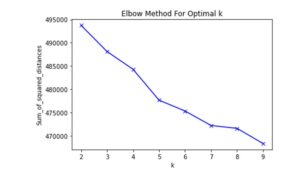
Figure 2. Elbow method, Source: Authors’ own work
Figure 2 displays the elbow graph obtained from our dataset. k represents the number of clusters by which the points are partitioned. For our analysis, we used 7 as the number of clusters, considering it is the point where the slope gets the closest to plateauing.
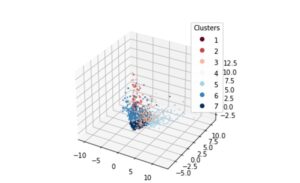
Figure 3. 3D representation of the clusters, Source: Authors’ own work
It can be noted from figure 3 that the clusters aren’t very well defined. It can be seen that they tend to merge. This was to be expected, due to the similar nature of the keywords by which the articles were searched for.
Results
As stated before, the articles were partitioned in 7 clusters. In the following, statistics for each cluster will be presented, in comparison with the Searched Keyword.
Table 3. Count of articles by Searched Keyword and Cluster
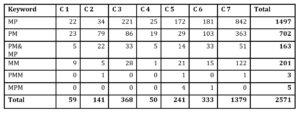
Source: Authors’ own work
From table 3 multiple pieces of information can be extracted. First, it can be observed that, by Searched Keyword, most articles were found for management processes, followed by process management. Process management maturity and management process maturity returned the least number of articles, 3 and 5, respectively.
Furthermore, the cluster with the greatest number of articles is cluster 7, which contains over 53% of the total. It is followed by cluster 3 and cluster 6.
It can be noted that 80% of the articles found by the management process maturity Searched Keyword belong to cluster 5. In contrast, all three of the process management maturity articles belong to different clusters: 2, 5 and 7, respectively. In the following, an analysis of average number of citations will be presented.
Table 4. Mean Citations of articles by Searched Keyword and Cluster
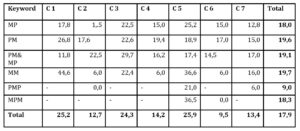
Source: Authors’ own work
From table 4 it can be noted that the articles have, on average, the same number of citations when they are grouped by Searched Keyword. The only keyword that returns significantly different results is process management maturity (9), 9 – 10 citations less than the other keywords.
When looking at the number of citations’ distribution by clusters, they can be grouped in three classes. Cluster 1, 3 and 5 have a similar number of citations on average: 25,2, 24,3 and 25,9. Cluster 2, 4 and 7 have a similar number of citations on average: 12,7, 14,2 and 13,4. A third class is composed only of cluster 6, which has the least number of citations on average: 9,5.
Table 5. Distance between clusters (Cluster Centers)
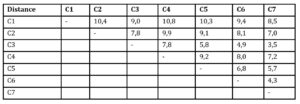
Source: Authors’ own work
Table 5 presents the distance between clusters, calculated between cluster centers. The maximum distance is 10,8, between cluster 1 and cluster 4. The minimum distance is 3,5, between cluster 3 and cluster 7. The average distance between clusters is 7,8.
In order to better understand how k-means clustered the data, feature importance was performed. The results are presented in figure 4. The values on the X axis represent the feature importance score.
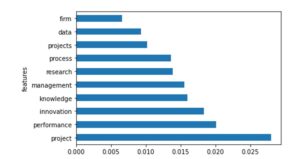
Figure 4. Clustering Feature Importance, Source: Authors’ own work
The most important word by which the articles were clustered is project, followed by performance, innovation, knowledge and management. It can be noted that some neutral words in the base of our study are used, such as research and data.
Table 6 presents the 10 most frequent words by cluster. From it, characteristics about each cluster can be extracted. It can be deduced that articles in cluster 1 focus on big data (top 2 words). Comparatively, articles in the second cluster are on the subject of business process management (top 3 words). Articles in cluster 3 talk about knowledge management (2nd and 3rd most frequent words), while articles in cluster 4 focus on digital services (top 3 words). Project management is the main topic of articles in cluster 5 (top 3 words), performance and innovation management the topic of articles in cluster 6 (top 3 words) and cluster 7 articles discuss management models and information.
Table 6. Top 10 words by word frequency grouped by cluster
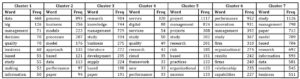
Source: Authors’ own work
Looking at table 6, it can also be understood why k-means found project and performance the two most important features to cluster the articles. They both are high frequency words that are found in a select portion of the database. This phenomenon is the clearest for project. It is found in abundance in cluster 5, but it doesn’t crack the top 10 for any other cluster.
Another interesting observation can be made. Cluster 5 (project management) contains the majority of articles with Search Keyword “management processes maturity”. For articles with Search Keyword “process management maturity”, the three clusters are 2, 5 and 7, with the most frequent words: business process models (cluster 2), project management (cluster 5) and information management model (cluster 7). All things considered, the sample is too small to consider these results significant, but the observation still had to be made and is an interesting one.
Discussion & Conclusions
After presenting and analyzing the results, we can return to our hypothesis. We believe that the answer can be found in the clustering of the articles and what each cluster represents. In this sense, articles representative of management processes maturity (a) were partitioned mainly to cluster 5. Research articles representative of process management maturity (b) were dispersed in clusters 2, 5 and 7. Furthermore, (a) focus mainly on project management, while (b) tackle business process models, project management and information management models. Inferring from these findings, it can be concluded that the two concepts, while apparently similar, are quite different. One is more focused, while the other is used in a more varied manor. This is also backed by research and common sense. Management processes maturity will naturally be tied to project management, while process management maturity has a larger base of application, seeing how there are a plethora of process types, not just management.
Future work could consist of a larger database, adding more Search Keywords, such as business process management, different clustering algorithms and more complex AI algorithms, such as Neural Networks.
In closing, we believe that this article can serve as the foundation for future research into the field of BPM comparative analysis.
Acknowledgements
This paper was co-financed by POLITEHNICA University of Bucharest and The Bucharest University of Economic Studies during the PhD program.
References
- Aggarwal, C. C. & Zhai, C. X., 2012. Mining Text Data. New York: Springer Science & Business Media.
- Andersen, E. S. & Jessen, S. A., 2003. Project maturity in organisations. International Journal of Project Management, 19 Noiembrie, Issue 21, pp. 457 – 461.
- Arthur, D. & Vassilvitskii, S., 2007. k-means++: The Advantages of Careful Seeding. New Orleans, Society for Industrial and Applied Mathematics, pp. 1027-1035.
- Bailey, K. D., 1994. Typologies and Taxonomies. An Introduction to Classification Techniques (Sage University Paper series on Quantitative Applications in the Social Sciences, series no. 07-102). Thousand Oaks, CA: Sage.
- Benner, M. J. & Tushman, M. L., 2003. Exploitation, exploration, and process management: the productivity dilemma revisited. Academy of Management Review, pp. 238-256.
- Bernstein, L. & Wild, J. J., 1999. Analysis of financial statements. 5th ed. New York: McGraw-HiIl.
- Bititci, U. S. et al., 2011. Managerial processes: business process that sustain performance. International Journal of Operations & Production Management, 31(8), pp. 851-891.
- Bodzioch, S. & Orgiela, M. R., 2009. New approach to gallbladder ultrasonic images analysis and lesions recognition. Computerized Medical Imaging and Graphics, 33(2), pp. 154-170.
- Brocke, J. v. & Schmiedel, T., 2015. BPM-Driving innovation in a digital world. Berlin: Springer International Publishing.
- Broens, T. et al., 2007. Determinants of succesul telemedicine implementations: a literature study. Journal of Telemedicine and Telecare, 13(6), pp. 303-309.
- Chatman, J. A. & Jehn, K. A., 1994. Assessing the relationship between industry characteristics and organizational culture: how different can you be?. Academy of Management Journal, pp. 522-553.
- Comuzzi, M. & Patel, A., 2016. How organisations leverage Big Data: a maturity model. Ind. Manag. Data Syst., pp. 1468-1492.
- Cronemyr, P. &. D. M., 2013. Process management 1-2-3 – A maturity model and diagnostics tool. Total Quality Management & Business Excellence, p. 933–944.
- Davenport, T. H., 1993. Process Innovation: Reengineering Work Through Information Technology. Boston: Harvard Business School Press.
- De Bruin, T. F. R. K. U. &. R. M., 2005. Understanding the main phases of developing a maturity assessment model. s.l., s.n., pp. 8-19.
- de Oliveira, O. J., Reis, R. L. & Mathias, M. A. S., 2017. Maturity models: identifying the state-of-the-art and the scientific gaps from a bibliometric study. Scientometrics, Volume 110, pp. 643-672.
- Dumas, M., La Rosa, M., Mendling, J. & Reijers, H. A., 2018. Fundamentals of Business Process Management. Berlin: Springer.
- Dumas, M., Rosa, M., Mendling, J. & Reijers, A. H., 2012. Fundamentals of Business Process Management. 1st ed. Tartu(Estonia): Springer.
- Fisher, D. M., 2004. The business process maturity model: A practical approach for identifying opportunities for optimization. BPTrends, pp. 1-7.
- Fraser, P., Moultrie, J. & Gregory, M., 2002. The use of maturity models / grids as a tool in assesing product development capacility: a review. Cambridge, UK, IEMC, pp. 244-249.
- Froger, M., Bénabenb, F., Truptil, S. & Boissel-Dallier, N., 2019. A non-linear business process management maturity framework to apprehend future challenges. International Journal of Information Management, Volume 49, pp. 290-300.
- Gassmann, O., 2006. Opening up the innovation process: towards an agenda. R&D Management, pp. 223-228.
- Gorog, M., 2016. A broader approach to organisational project management maturity assessment. International Journal of Project Management, 34(8), pp. 1658-1669.
- Gottschalk, P., 2009. Maturity level for interoperability in digital government. Government Information Quarterly, pp. 75-81.
- Hachaj, T. & Ogiela, M. R., 2011. A system for detecting and describing pathological changes using dynamic perfusion computer tomography brain maps. Computers in Biology and Medicine, 41(6), pp. 402-410.
- Hammer, M., 2015. What is Business Process Management?. Berlin: Springer.
- Harmon, P., 2015. The scope and evolution of business process management. Berlin: Springer.
- Heinrich, B., Schiller, A. & Schön, D., 2018. The cooperation of multiple actors within process models: an automated planning approach. Journal of Decision Systems, pp. 238-274.
- Hoffman, J., Weber, I. & Kraft, F. M., 2012. SAP speaks PDDL: exploiting a software-engineering model for planning in business process management. Journal of Artificial Intelligence Research, pp. 587-632.
- Iacob, D. & Cismaru, D.-M., 2012. Organizatia inteligenta: Zece teme de managementul organizatiilor. 2nd ed. Bucharest, Romania: Comunicare.ro.
- Jain, A. K., 2010. Data clustering: 50 years beyond K-means. Pattern Recognition Letters, pp. 651-666.
- Laudon, K. C. & Laudon, J. P., 2002. Management information systems – Managing the digital firm. New Jersey: Prentice-Hall International: Inc..
- Lemos, A. L., Daniel, F. & Benatallah, B., 2016. Web service composition: a survey of techniques and tools. ACM Computing Surveys (CSUR), p. 33.
- Macedo de Morais, R., Kazan, S., Inês Dallavalle de Pádua, S. & Lucirton Costa, A., 2014. An analysis of BPM lifecycles: from a literature review to a framework proposal. Business Process Management Journal, 20(3), pp. 412-432.
- Meerkamm, S., 2010. The Concept of Process Management in Theory and Practice – A Qualitative Analysis. Berlin: Springer.
- Mironescu, R., 2013. Elemente Fundamentale de Management. Bacău(România): Alma Mater.
- Moradi-Moghadam, M. S. H. &. M. M., 2013. A novel model for business process maturity assessment through combining maturity models with EFQM and ISO 9004:2009. International Journal of Business Process Integration and Management, p. 167–184.
- Neff, A. A. et al., 2013. The influence of IT governance on service processes in multi-business manufacturing enterprises: performance impacts from structures, processes, and relational mechanisms. Chicago, Association for Information Systems, pp. 1-9.
- Nicolescu, O. & Verboncu, I., 1999. Management. Bucharest: Editura Economică.
- Ogiela, L., 2013. Semantic analysis and biological modeling in selected classes of cognitive information systems. Mathematical and Computer Modelling, pp. 1405-1414.
- Ogiela, L., 203. Data management in cognitive financial systems. International Journal of Information Management, pp. 263-270.
- Ogiela, L. & Ogiela, M. R., 2011. Semantic Analysis Processes in Advanced Pattern Understanding Systems. Berlin, Springer, pp. 26-30.
- Ogiela, M. R. & Ogiela, L., 2012. Cognitive Systems Monographs: Advances in Cognitive Information Systems. Berlin: Springer-Verlag.
- Orgiela, M. R. & Orgiela, U., 2014. Secure information management using linguistic threshold approach. London: Springer-Verlag.
- Paulk, M. C., Curtis, B., Chrississ, M. B. & Weber, C., 1993. Capability maturity model for software. IEEE Software, pp. 18-27.
- Pöppelbuß, J. R. M., 2011. ‘What makes a useful maturity model? A framework of general design principles for maturity models and its demonstration in business process management’. s.l., s.n.
- Power, B., 2007. Hammer’s process and enterprise maturity model. BPTrends, pp. 1-4.
- Rodriguez, M. Z. et al., 2019. Clustering algorithms: a comparative approach. Plos One.
- Röglinger, M., Pöppelbuß, J. & Becker, J., 2012. Maturity Models in Business Process Management. Business Process Management Journal, pp. 328-346.
- Röglinger, M., Pöppelbuß, J. & Becker, J., 2012. Maturity Models in Business Process Management. Business Process Management Journal, pp. 328-346.
- Rohloff, M., 2009. Process management maturity assessment. s.l., s.n.
- Rosemann, M., 2014. Proposals for future BPM research directions. s.l., s.n., pp. 1-15.
- Rosemann, M. D. B. T. &. P. B., 2006. A model to measure business process management maturity and improve performance. Business process management, pp. 299-315.
- Rosing, M., Scheer, A.-W. & Scheel, H., 2015. The Complete Business Process Handbook. Body of Knowledge from Process Modeling to BPM. 1 ed. Waltham(Massachusetts): Elsevier Inc..
- Trkman, P., 2010. The critical success factors of business process management. International Journal of Information Management, pp. 125-134.
- van der Aalst, W., 2016. Process Mining: Data Science in Action. Berlin: Springer.
- van der Aalst, W. et al., 2012. Process mining manifesto. Business process management workshops, pp. 169-194.
- van der Aalst, W. M., 2013. Business Process Management: A Comprehensive Survey. International Scholarly Research Netices Software Engineering, pp. 1-37.
- van der Aalst, W., Netjes, M. & Reijers, H., 2007. Supporting the full BPM life- cycle using process mining and intelligent redesign. Contemporary issues in database design and information systems development, pp. 100-132.
- Wendler, R., 2012. The maturity of maturity model research: A systematic mapping study. Information and Software Technology, 54(12), pp. 1317-1339.
- Xu, Y. et al., 2016. Context-aware QoS prediction for web service recommendation and selection. Expert Systems with Applications, pp. 75-86.