Introduction
Supply chain management (SCM) from today is not the same as the past few years. Recently it has been empowered by newer technologies such as Robotics, Artificial Intelligence, Big Data Analytics, Blockchain, Internet of Things, and Cloud Computing. A further improvement in operational efficiency will depend on these technologies within “intelligent” connected factories. The process improvement is not driven by human initiatives within specific fields but by the ample integration of computers and machines that encompasses the entire process operations. Information technology (IT) was perceived as strategic relevance, process control, research and development, customer service, coordination, costs. At the same time, IT is redesigning the competition’s environment and establishing new rules focused more on efficiency, productivity, speed, quality, and customer orientation. Enterprise resource planning (ERP) systems are purchased to reorganize the business transactions, and TCP/IP networks simplify the communication within an organization. Advanced databases, in correlation with data mining techniques, analyze the purchasing patterns of clients in the expectation of increasing into a potential market segment. Technical efficiency is a significant and valuable economic measure of organizational performance. Artificial Intelligence (AI) algorithms and Machine Learning (ML) methods are utilized in real-world scenarios in SCM. ML is a technology used to “teach” the machines how to function with data more efficiently, simulating the learning concept of rational beings and can be integrated with AI algorithms, reflecting the methods of rational characteristics such as genetics, statistics, and probabilities, based on cases [1]. Applying AI algorithms based on the ML approach, it is possible to extract and explore information to classify, predict, associate, optimize, group, and identify patterns. AI is a concept divided into the main fields of application, such as natural language processing, automatic programming, robotics, computer vision, automatic theorem proving, intelligent data retrieval, and is defined as a group of core ideas that underline many of these applications [2]. The core principle of intelligent SCM is using AI by machines to complete complex tasks, reduce costs, and improve the quality of goods and services [3]. It makes the manufacturing industry intelligent and capable of addressing modern challenges like customizable requirements, reduced time to reach the market, and increasing sensors used in equipment [4]. The use of flexible robots combined with AI allows for easier manufacturing of different products and can analyze large volumes of real-time data gathered from various sensors [5]. It is essential when developing new technologies to have the ability to put a value on efficiency measures for an intelligent and interconnected SCM.
The efficiency of IT solutions in Supply Chain Management
SCM analytics has been presented as an effective method to analyze, disseminate, collect and use Big Data information. Effectiveness, efficiency, responsiveness, flexibility, and sustainability are the main five dimensions to measure accurately [6]. The key characteristics of an efficient SCM are: agility, alignment, and adaptability [7]. Assuring connectivity, trust, transparency, and communication within an SCM is challenging [8] and may lead to a dramatic effect on SCM flexibility, sustainability, and resilience, along with other vital characteristics. Flexibility is the measure of control and adaptability of an SCM against changes in market demand. Agility can react to such unexpected situations quickly and maintain efficiency, availability, and capability. Resilience delivers a comprehensive perspective on the SCM performance, which is described as its capability to maintain sustainable behavior when exposed to disruptive events [9]. (See Fig. 1.)
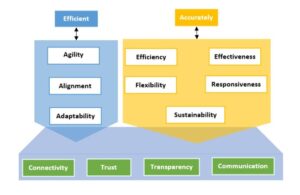
Fig 1. Performance framework in Supply Chain Management
Cloud Computing
Some examples on how Cloud Computing increases efficiency are: easy data recovery (data and information records that are stored in the cloud, are easier to retrieve – compared to when they are stored at a local level and avoid expensive means of data recovery); efficient remote working (provides flexibility to workforce, employees can access and share files and documents anywhere at any time); reduced hardware, software and operational costs (can help the SCM to cut the cost of purchasing, maintaining and upgrading IT equipment and systems, save operational costs and reduce the level of IT expertise); increased collaboration and productivity (teams efficiently collaborate and execute tasks faster, chat and work with colleagues, can access and work simultaneously on documents); first-class tools and technology (can provide access to the latest technology); easy-to-scale IT infrastructure (quickly scale the IT requirements when required, and adapt with cloud computing); improved accessibility (provides access anytime, anywhere); undivided attention to innovation (can provide an environment for innovation and brings effectiveness and efficiency, because the innovation provides a competitive edge and helps to outperform the competition). (See Fig 2.)
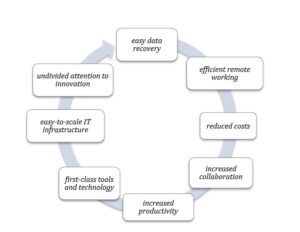
Fig 2. Efficiency characteristics with Cloud Computing in Supply Chain Management
Big Data analytics
Big data extend above the ability of average database software tools to analyze, capture, store, and manage them effectively. One of the most challenging purposes in SCM is to improve operational efficiency by providing better decision-making in terms of operational and strategic resource planning and improving performance and process quality, as operational efficiency is about providing high-quality services most cost-effectively. Utilizing the capabilities of Big Data technologies to optimize the utilization of resources and reduce operational costs can be an essential advantage for any logistics provider [10]. Some examples of how Data Analytics increases efficiency are: finding new ways to improve the efficiency (analyze historical data and current trends, systems can highlight areas of improvement, revealing ways SCM can do better and predicting success rates); measuring and reporting real-time data (Historical data can help SCM become more efficient, by measuring the changes implemented and their efficacy, being able to display the results in real-time); better understanding of customers; identification and elimination of SCM inefficiencies; (enhance operational efficiency and deliver better services to customers and assist to analyze market trends and suggest optimum inventory levels); delivery optimization (the delivery routes can be analyzed and automatically changed according to new real-time information on traffic conditions, weather, or any other incidents. The automated control of large fleets of moving delivery resources can be utilized effectively using the real-time processing capabilities of Big Data technologies. [11]); transport route optimization (optimal route discovery and shortest path problems can likewise be solved by analyzing Big Data and using data-driven approaches. [12][13]); smart logistics (the more extensive traffic information, the more efficiently the logistics and transport flows can be self-managed and optimized); anticipatory logistics (prediction of demands before requests and orders are placed, which boosts the service quality and process efficiency and leads to shorter delivery times). (See Fig 3.)
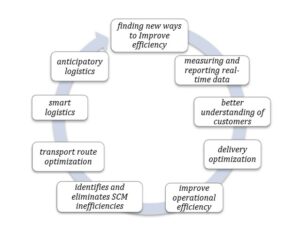
Fig 3. Efficiency characteristics with Data Analytics in Supply Chain Management
Robotics
With so much pressure on finding a way to drive efficiency, SCM is applied to Robotic Process Automation (RPA) to help them achieve efficiency. By adopting RPA, SCM can enhance efficiency, minimize operational costs, improve quality, and allow the automation of business processes. Because RPA can work 24 hours out of seven days a week, it is increasing production and accuracy by dropping cycle times and decreasing the need for ongoing training, can work with structured and semi-structured data efficiently, offering the best results in each execution. RPA can raise the efficiency and decrease costs; some examples of how RPA increases efficiency are [14]: higher efficiency, faster processing time, finding flaws in the system (collection of data on every process and reports them as key analytics), more invested employees, high accuracy and quality, consistency (all tedious and repetitive tasks and processes can be automated which helps to eliminate the risk of human error). (See Fig 4.)
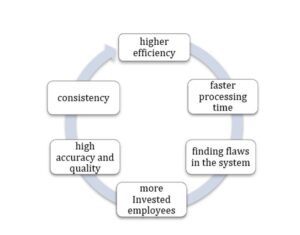
Fig 4. Efficiency characteristics with Robotics in Supply Chain Management
Internet of Things
Logistics can be managed with IoT to improve efficiency within SCM [15]. Some examples on how IoT increases efficiency are: improved efficiency of SCM (the cost reduction techniques, spending patterns, and material efficiency will continue to be the top priorities in SCM); remote operation of machinery (IoT enables the technicians to operate machinery from a distant location so that they could avoid potentially hazardous work conditions); visualization of the logistic patterns (track quality, quantity, and cost of the resources, comparison of the plans vs actual delivery and monitor and inspect the delivered product [16]); develop a decision-support system (to generate a production and supply plan for the supplier and buyers of steel rebar [17]); ready-mix concrete operations (by following the position of trucks and observing the motion and status of concrete conveyances in real time [18]); remote monitoring (monitoring the tools and the equipment on a job site [19]); improved production quality (providing real-time data that can help SCM to maximize its potential); reduce machine downtime (facilitates quick detection of possible system and equipment failure); maintenance of equipment. (See Figure 5)
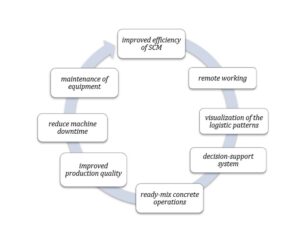
Fig 5. Efficiency characteristics with the Internet of Things in Supply Chain Management
Artificial Intelligence
Some examples of how Artificial Intelligence increases efficiency are: increased security (enhanced protective and secured measures in the network of the business, detecting anomalies); better customer support (technology is reducing the cost by hiring fewer customer service agents); enhancing productivity (automating tasks such as data entry, accounting and payroll administration, and access all the necessary customer data quickly to improve the efficiency and reduce errors); improving forecasting capabilities (detects seasonality and makes references about increasing and decreasing levels of production); hyper-personalized manufacturing (products and services that are highly relevant to individual consumers); optimizing manufacturing processes (monitor the quantities used, cycle times, temperatures, lead times, errors, and downtime to optimize production runs). (See Figure 6)
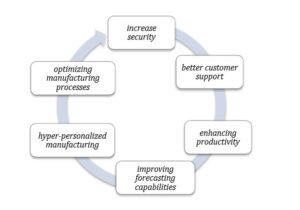
Fig 6. Efficiency characteristics with Artificial Intelligence in Supply Chain Management
Blockchain
Blockchain technology is defined as a “chain of blocks,” each “block” stores a group of data about its past, present, and future which plays a crucial role in connecting with the previous block and the following block to be a part of the chain. The role of blocks is to record, validate, and distribute the transactions among other blocks, and it cannot be removed or altered as this would change every subsequent block. This technology could disrupt difficult and time-consuming traditional governance models to improve sustainability outcomes [20] and, by distributing data across a network, can eliminate information asymmetry and create novel platforms for interactions. Some examples of how Blockchain increases efficiency are: the increased transparency (data can be shared transparently on a secure and tamperproof platform); lower transaction cost (reduces the complexity of the process and the associated costs and can significantly reduce the transaction cost of administering energy-related contracts); increased reliability (improved reliability of the overall system); increased security (customers’ energy-saving data, information will be encrypted); increased customer trust (make the process automated rather than manual); increased market success (can bring security, transparency, trust, reduced administrative cost, authorization of energy savings, and payments in an automated way). (See Figure 7)
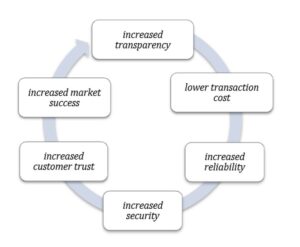
Fig 7. Efficiency characteristics with Blockchain in Supply Chain Management
Analysing The It Solutions Efficiency
IT improvements enabled the SCM to enhance the efficiency of the processes through information sharing and advanced IT utilization [21] which could improve the operational efficiency and expedite competitive advantage. As a result, the employment of IT enhances operational performance. Technology selection is an essential part of an enterprise’s performance optimization technologies; besides the competitive advantages created to realize profit growth, they add capability improvement and realize sustainable development. The integrated consideration of production, inventory, and delivery processes can improve the overall manufacturing performance.
In SCM, IT solutions are in-demand to guarantee effective integration of manufacturers and customers [22][23] and provide the implementation of various services, such as SCM solutions (demand management, product life cycle management, Etc.), logistics solutions (inventory management, optimization, purchase, Etc.), vertical specialization (by type of economic activity), platform (Cloud Computing, SaaS, Etc.); base cost (free of charge), transactional (subscription); servicing of other economic activities (production, retail, manufacturing, mechanical engineering Etc.) and additional solutions (Blockchain, asset management, Big Data management, Etc.). Implementing IT solutions in SCM provides increased efficiency, and there is a gradual decrease in administrative, operational, and other costs within the enterprise [24]. The core principle of intelligent SCM is the use of AI in SCM to complete complex tasks, reduce costs, and improve the quality of goods and services [25]. AI technologies are permeating the manufacturing industry and merging the physical and virtual worlds. They are transforming the manufacturing industry into an innovative and capable industry of addressing modern challenges like customizable requirements, reduced time to reach the market, and increasing sensors used in equipment [26]. AI methods can analyze large volumes of real-time data gathered from various sensors [27]. In an environment with a high volume of data, more accessible technologies can be further developed to achieve the goal of intelligent and self-learning SCM [28]. Investing in technology (Cloud Computing, Data Analytics, Artificial Intelligence, Internet of Things, Robotics, and Blockchain) improves the efficiency of the existing operations by substituting labor, reducing inventory, or eliminating waste. (See Figure 8)
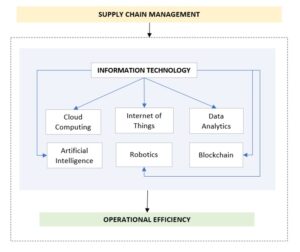
Fig 8. Information Technology efficiency value in Supply Chain Management
Conceptual Frameworks of the IT solutions in SCM were designed; these were divided based on the technology’s ability to acquire operational efficiency once implemented in SCM. The operational efficiency for the most relevant technologies used in SCM is Cloud Computing, Big Data Analytics (BDA), the Internet of Things (IoT), Artificial Intelligence (AI), Robotics Process Automation (RPA), and Blockchain. The conceptual frameworks are split based on the efficiency characteristics that are similar through the technologies and not similar through the technologies.
Conceptual framework on the distinct efficiency characteristics of technologies
Blockchain technology will be possible to provide a secure, transparent, and trusted digital transaction platform. AI can increase efficiency, as it provides hyper-personalized services and products and is improving the forecast capabilities. Cloud Computing technology has a significant impact on SCM processes optimization. It can increase the collaboration between networks; it is an easy tool for data recovery, is energy efficient, and has an easy-to-scale IT infrastructure; this Cloud Computing opens the doors for many innovations in SCM. Data Analytics transforms traditional logistics into smart logistics and has the power to make anticipations while measuring and reporting data in real-time. IoT is helping the SCM to increase operational efficiency, and the factory machine downtime is reduced while providing a decision support system. Robotics or Robotic Process Automation has significantly improved the productivity in SCM; this technology is impacting the employees, and they are capable of being more invested in work activities; besides this, RPA has high accuracy, quality, and consistency. (See Figure 9)
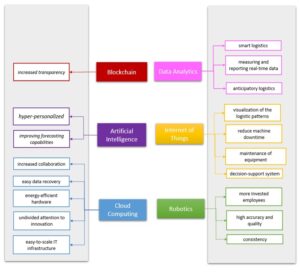
Fig 9. Conceptual framework on the distinct efficiency characteristics of technologies
Conceptual framework on the similar efficiency characteristics of technologies
Increased security of the SCM is better achieved with Blockchain and AI, while Cloud Computing and IoT are helping the SCM to be more efficient while employees can work remotely. With Data Analytics and Robotics that are identifying and eliminating the inefficiency, the processes are more optimized. Cloud Computing and AI technologies increase productivity, and with Blockchain technology, the overall costs are reduced. Data Analytics, Blockchain, and AI are increasing customer support and trust and are increasing the market success. (See Figure 10.)
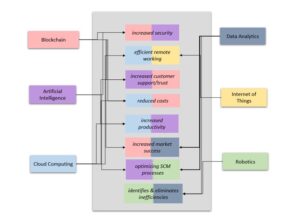
Fig 10. Conceptual framework on the similar efficiency characteristics of technologies
Conclusion
Technology and software will continue to revolutionize the SCM in the coming decades. AI programming will improve, and device functionality will increase. The adoption of technology will require a paradigm shift away from thinking of SCM in terms of specific functional areas and toward a complete connection of all functional areas into the creation of one dynamic and organic ecosystem, aided by advanced analytics software, machine learning, and AI where greater operational efficiency will be achieved. The subject of quality is an essential concept for the efficient management of the inventory in SCM.
References
- Mitchell, T. M. (1997). Machine Learning. New York: McGraw-Hill. ISBN: 978-0-07 -042807-2
- Nilsson, N. J. (2014). Principles of artificial intelligence. Morgan Kaufmann Editors.
- Bahrin, M. A. K., Othman, M. F., Azli, N. N., & Talib, M. F. (2016). Industry 4.0: A review on industrial automation and Jurnal Teknologi, 78(6-13), pp:137-143.
- Zheng, P., Sang, Z., Zhong, R. Y., Liu, Y., Liu, C., Mubarok, K., … & Xu, X. (2018). Smart manufacturing systems for Industry 4.0: Conceptual framework, scenarios, and future perspectives. Frontiers of Mechanical Engineering, 13(2), pp:137-150.
- Ustundag, , & Cevikcan, E. (2017). Industry 4.0: managing the digital transformation. Springer Editors. Available from: https://www.springer.com/gp/book/9783319578699
- Adivar B, Hüseyinoğlu IÖY, Christopher M. A quantitative performance management framework for assessing omnichannel retail supply chains. J Retail Consum Serv 2019;48:257–69. doi:10.1016/J.JRETCONSER.2019.02.024.
- Lee HL. The Triple-A Supply Chain. Harv Bus Rev 2004;82:102–13.
- Wang Y, Wallace SW, Shen B, Choi T-M. Service supply chain management: A review of operational models. Eur J Oper Res 2015;247:685–98. doi:10.1016/J.EJOR.2015.05.053.
- Farsi M, Hosseinian-Far A, Daneshkhah A, Sedighi T. Mathematical and Computational Modelling Frameworks for Integrated Sustainability Assessment (ISA). Strateg. Eng. Cloud Comput. Big Data Anal., Springer International Publishing; 2017, p. 3–27. doi:10.1007/978-3-319-52491-7_1.
- Vesna Radonjic Dogatovic Branka Mikavicaa, Aleksandra KosticLjubisavljevica. Big data: Challenges and opportunities in logistics systems. 2015
- Wei M.Jeseke, M.Grner. Big data in logistics a dhl perspective on how to move beyond the hype. Technical report, DHL Customer Solutions and Innovation, December 2013
- Li, X. Li, and J. Yin. Tord problem and its solution based on big trajectories data. IEEE Transactions on Intelligent Transportation Systems, 17(6):1666–1677, June 2016
- Cao, H. Guo, J. Zhang, D. Niyato, and U. Fastenrath. Finding the shortest path in stochastic vehicle routing: A cardinality minimization approach. IEEE Transactions on Intelligent Transportation Systems, 17(6):1688–1702, June 2016
- UiPath, Benefit of RPA: Efficiency (2015), https://www.uipath.com/blog/rpa/benefit-of-rpa-efficiency (linked accessed at September 2021)
- GRAHAM IMMERMAN, Improving Operational Efficiency with Industrial IoT, MACHINEMETRICS, 2019, https://www.machinemetrics.com/hubfs/MachineMetrics%20Ebook:%20Improving%20Operational%20Efficiency.pdf
- Irizarry, J., Karan, E. P., & Jalaei, F. Integrating BIM and GIS to improve the visual monitoring of construction supply chain management. Automation in Construction, 31, 241-254, 2013.
- Tserng, H. P., Yin, S. Y., & Li, S. Developing a resource supply chain planning system for construction projects. Journal of Construction Engineering and Management, 132(4), 393-407, 2006
- Lu, M., Dai, F., & Chen, W. Real-time decision support for planning concrete plant operations enabled by integrating vehicle tracking technology, simulation, and optimization algorithms. Canadian Journal of Civil Engineering, 34(8), 912-922, 2017
- Sasaki, T., & Kawashima, K. Remote control of backhoe at construction site with a pneumatic robot system. Automation in construction, 17(8), 907-914, 2008.
- Downes, L.; Reed, C. Blockchain for Governance of Sustainability Transparency in the Global Energy Value Chain; Legal Studies Research Paper. No. 283/2018; Queen Mary University of London, School of Law: London, UK, 2018.
- Kim, S. W. (2012) An investigation of Information Technology investments on buyer-supplier relationship and supply chain dynamics. Asian Journal on Quality, 13(3), 250-267
- Douglas M. 2020 Logistics IT Market Research Survey. Inbound Logistics. 2020. URL: https://www.inboundlogistics.com/cms/article/2020-logistics-it-market-research-survey.
- Gavurova B., Bacik R., Fedorko R., Rigelsky M. Analytical view of online marketing tools in the dimension of marketing campaigns’ personalization in Slovakia. Marketing and Management of Innovations. 2018. No 2. Р. 186—200.
- Nitsenko V., Mukoviz V., Sharapa O. Accounting of transaction expenses of economic entities. Scientific Bulletin of Polissia. 2017. No 4 (12), Part 2. Р. 71—78.
- Bahrin, M. A. K., Othman, M. F., Azli, N. N., & Talib, M. F. (2016). Industry 4.0: A review on industrial automation and Jurnal Teknologi, 78(6-13), pp:137-143.
- Zheng, P., Sang, Z., Zhong, R. Y., Liu, Y., Liu, C., Mubarok, K., … & Xu, X. (2018). Smart manufacturing systems for Industry 4.0: Conceptual framework, scenarios, and future perspectives. Frontiers of Mechanical Engineering, 13(2), pp:137-150.
- Ustundag, , & Cevikcan, E. (2017). Industry 4.0: managing the digital transformation. Springer Editors. Available from: https://www.springer.com/gp/book/9783319578699
- Lee, J., Kao, H., Yang S. (2014), “Service innovation and smart analytics for Industry 4.0 and big data environment”, Proceedings of the 6th CIRP Conference on Industrial Product-Service Systems, v. 16, p. 3-8