Introduction
Recent advancement in technologies around artificial intelligence, machine learning, blockchains, the Internet of Things (IoT) and distributed systems affect how organizations execute and design business processes (Mendling et al., 2018). Processes are perennial in any organization, enterprise, or business (Bandara et al., 2007). Effective implementation of Business Process Management (BPM) utilizes processes as a critical link to recent technologies to translate strategy into execution, while delivering sustainable value to an organization (Franz & Kirchmer, 2015). At the same time, the fundamental purpose of conventional BPM is to lower costs and produce more efficient processes (Harmon & Tregear, 2016). Concurrently, a new BPM wave is evolving to deliver business agility, an improved customer experience and digital automation (Kalmijn, 2019), which can foster regulatory compliance, risk mitigation, business continuity and sustainability (Harmon & Treager, 2016). Finally, advancements in machine learning, artificial intelligence and distributed systems can and will influence the design and business process execution of organizations in the future (Mendling et al., 2018).
Through BPM, the entire cross-organizational value chain has the capacity for innovation to transform businesses continually (vom Brocke & Rosemann, 2014). BPM is a discipline where a variety of methods are used to identify (Dumas et al., 2018), model, analyse, measure, and transform processes, improve inefficiencies, optimize, automate and even eliminate manual processes (Jeston et al., 2008). Most business process definitions relate to business goals and value creation (Heindel &Weber, 2020). Copious quantities of data in organizations enable low level event data to be analysed using process models as a ‘lens’, which aids organizations to understand and solve performance and compliance related problems (vom Brocke, & Mendling, 2018). Data sources have also broadened with widespread adoption of mobile or portable devices such as smart phones, smart watches, or tablets (Bachiller et al., 2018). In the business-IT divide, process enforcement technologies are widely acknowledged to have the capability to embrace the so-called “missing-middle” (Davenport, 2019). As technology develops, it can become overly complex (Kiela et al., 2016), however, process-aware information systems (Kalenkova, 2017), or process mining providing holistic insight into actual processes allowing inefficiency identification and uncovering of effort drivers, represent some of the most innovative digital tools supporting the digital transformation of a company (Reinkemeyer, 2020). Event data’s omnipresence in combination with process mining capabilities enables organizations to diagnose a problem, not from fiction but facts (van der Aalst, 2016). Companies such as Walmart, UPS, and Uber leverage technology to create new profitable business models, which is one area where technology has the ability to improve performance without humans (Davenport et al., 2019).
For a company to reap the benefits of BPM, it is vital a firm outlines the business drivers, articulates its process scope, and sets clear goals on deployment strategies. Likewise in the Australian higher education context, several substantial issues are emerging as higher education increasingly becomes diverse, complex, financially challenged and internationally dependent (Brett et al., 2017). A high level of consensus in shared governance is the most important issue in most academic organizations, while international rankings, technology transfer, student accessibility, declining federal budget support and student financial aid are just some of the other issues identified. Regardless of which Australian university lobby group membership – the Group of Eight (Go8), the Australian Technology Network (ATN), the Regional Universities Network (RUA), the Innovative Research Universities (IRU) or the non-aligned, they all share key characteristics (Brett et al., 2017) – namely sector leaders need to explore options to reposition institutions into new disruptive business models (Cawood et al., 2017).
Australian Higher Education
The main drivers of the Australian economy are the healthcare, finance, and higher education sectors (Labour Force Australia, 2020), where higher education forms the focus of this study. In Australia’s economy, education serves as a central pillar, being the heartbeat of communities with a major contribution socially, economically, culturally, and intellectually (Brett et al., 2017). Over the last three decades, Australian universities have experienced significant changes and are at a pivotal time of evolution and development (Brett et al., 2017). However higher education has around 70 years of reported success, such that it has become complacent to change (Fitzgerald et al., 2013). A commitment to research, comprehensive course offerings and a high number of enrolments represent an expensive way to deliver higher education (Cawood et al., 2018), hence no Australian university can survive 2025 with the current business model (Brett et al., 2017). The education sector employs 8% of Australian workers and was the largest service export with total earnings of $20.3 billion in 2015/2016 in international education. The higher education sector alone contributes $30 billion to the country’s Gross Development Product (GDP). In the Times Higher Education (THE) world rankings, thirty-five Australian universities feature, with six in the world’s top 100 (Cawood et al., 2018). In sixty-six years, student numbers increased from around 31,000 in 1949 to 1.4 million in 2015 (Brett et al., 2017).
In the past three decades, major developments have focused on three fundamental academic functions of the university – research and knowledge generation, teaching and learning dissemination, and outreach and engagement (Brett et al., 2017). People in Australia are reasonably innovative, however models that work in the UK, the Netherlands or elsewhere in Europe, may not be applicable here because the level of industry investment is simply not present (Brett et al., 2017). One of the greatest opportunities to strengthen the Australian system is through international partnerships. While the UK and the US are two strong traditional partners, China, Germany and even India have great potential to be transformative for Australia. The growing desire of the current generation in undergraduate learning is a practical experience involving partnerships or service-learning opportunities and to be work ready. Higher education has become a global market; hence the international mobility of students is something university leaders grapple with. Australia is too small not to be international (Brett et al., 2017), hence a major focus is on expanding partnerships globally.
Aims And Objectives
The focus here is an international education study abroad application that has been proven to be one of the key influencers of a higher employability rate of graduates. The problem here is to formulate a novel conceptual framework for a study abroad application process, where establishing process performance is the core target, while identifying the best process scenario to be adopted in an application cycle. In the study abroad application process, several challenges hinder the smooth flow of the process and a quality student experience. Some of the parameters are a need to lodge an application one year before the actual overseas trip; the process requires several documents; there exists a low conversion rate of actual acceptance; a complex eligibility assessment process; a lengthy academic credit application; a complicated host institution application process; and an intricate pre-departure orientation. Hence there is an increasing need to optimize the process to achieve efficient applications, improve the student experience and achieve the organization’s strategic aim to send 50% of students overseas for international education to strengthen employability upon graduation. Given the complexity of the existing process, traditional modelling takes longer to create, impeding analysis and improving the following application cycle. Additionally in the study abroad team, there exist different roles where salary varies. In a study abroad application in a 35-hour work week are 5 Exchange Advisers with a rate of $45.00/hour ($7,875.00/week) and 2 Exchange Coordinators at $52.00/hour ($3,640.00/week). Given an estimate of 20% process improvement, the annual estimated savings would be $118,300.00. Optimizing the process means resources can be re-allocated to higher value work, reducing process overheads. For students, the study abroad application process will be faster, resulting in a better student experience because of a quicker turnaround time. Hence the research questions posited here are:
RQ1: How do ML and AI-based BPM frameworks vary from conventional business process management solutions in higher education, specifically in the study abroad process in terms of reliability, profitability, cost-effectiveness, and trustworthiness?
RQ2: What are the pre-requisites in using data-driven and process mining capabilities of ML and AI to effectively implement BPM frameworks in the higher education study abroad process?
The goal here is thus to create a novel conceptual BPM framework enabled by artificial intelligence (AI) and machine learning (ML). For AI in process mining to work enterprise wide, special techniques, methods, tools, and process form pre-requisites (Akirraju et al., 2020); however recent breakthroughs in process mining enable process miners to uncover a process model automatically. Conformance to a process can be discovered through a predefined algorithm as to whether a process is reflected in the data, whilst uncovering deficiencies in performance, such as bottleneck detection (Schuster et al., 2020) to enhance business processes (van der Aalst, 2016) through gaining insights relating to the actual performance of these processes (Augusto et al., 2019). In this study, the event log from a study abroad application is used to analyse an end-to-end process, which is optimized to achieve the organizational target by improving the student experience through streamlining, removing bottlenecks and the adoption of the best possible process scenario to create an ideal process. The AI and ML novel BPM framework will be used after each study abroad application cycle to evaluate the process of a previous cycle and continuously improve the following cycle. Some of the distinct characteristics of the novel framework aims are its performance-based focus, rapid turn-around and output process-centric capabilities, that are not possible in the existing BPM lifecycle models.
Business Process Management
BPM is now considered a practice in organizations using numerous methods to design a process, perform analysis, measure performance, enhance inefficiencies and transform business processes. BPM is also a systematic approach to achieve coordinated and sustainable organizational goals that capture, shape, execute, document, measure, monitor and steer automated and non-automated processes (Paschek, Luminosu & Draghici, 2017), integrating people’s behaviour, systems, information and entities that produce a business end result to uphold business strategy. Although optional, technologies are generally used in conjunction with BPM, as a crux to developing business strategy (Gartner, 2014), where process support in a wider perspective incorporates analysis such as simulation, verification, and process mining (Weske, 2012). BPM refers to comprehensively improving and managing an organization’s end-to-end integrated enterprise-wide processes, to meet the fruition of three elements critical to performance-based goals driven by customer firms, which are to clarify strategic objectives, align resources and enhance regulation of day-to-day operations (vom Brocke et al., 2017). BPM also enables the identification of problems visually in processes and enables businesses to elucidate business processes improvements and testing prior to implementation. Several value-driven capabilities that BPM delivers throughout the organization are: improvements in cost, productivity and quality, accelerated timelines, improved customer service levels and satisfaction, simplified business processes to drive efficacy and agility. BPM also supports improved risk management and helps achieve compliance, better governance, an enhanced visual perspective on the performance of an organization, reduces costs and improves revenue streams (Rock et al., 2014).
The BPM lifecycle is considered to comprise six processes, namely identifying target processes, task discovery through qualitative or quantitative analysis, conducts process transformation, changes implementation and monitors and controls (vom Brocke et al., 2017; Dumas et al., 2018). At the same time, advancements in ML, AI and distributed systems, will likely influence the design and business process execution of organizations into the future (Mendling et al., 2018). The BPM lifecycle includes extensive options of process change frameworks such as Lean (Modig & Ahlstroem, 2019), NESTT, Six Sigma, TQM (Total Quality Management), CPI and CPM and SOX compliance legislation (van der Aalst, 2016).
BPM has evolved and is now in its third wave. The first wave was conceived in the 1920s and summarized in the theory of management by Fredrick Taylor, where process management was considered an analytical method and procedure. The second wave specifies what manual reengineering of processes can do through one-time activity. The third wave is the on-the-fly creation capability of optimizing redesigned business processes in the organization (Smith & Fingar, 2003). The principal purpose of conventional BPM is to minimize costs and produce more efficient processes. A new BPM wave is evolving to deliver business agility, customer experience and digital automation (Kalmijn, 2019). Another level of business process management is hyper-automation, a business-driven disciplined approach rapidly identifying, automating, and vetting as many business processes as possible. An example of hyper-automation involving the orchestration of technologies, tools and platforms involving AI or ML, includes robotic process automation (RPA), event driven software architecture and iBPMS (Kazamouzis & Stoudt-Hansen, 2020).
Research Model
Our initial step was to conduct qualitative analysis incorporating literature including case studies on the topic of process mining to solve business problems. In qualitative research, the method is inductive. Concepts are created and measured simultaneously within the data gathering process (Neumann, 2014). This step commenced with a survey of academic literature of different case studies from 2016 to 2020 covering process automation applications in BPM and process mining incorporating AI and ML. The analysis covered process mining applications found in books, conference papers, journals and unpublished papers, with a focus on peer reviewed publications. Filters used included the keywords ‘process mining’, ‘BPM’, ‘business process management’, ‘process discovery’, ‘conformance checking’, ‘process reengineering’ and ‘process monitoring’. Every article gathered provided insights on commonly used methodologies, tools or even frameworks.
The result of the data analysis was then used to conceptualize a framework. The initial step was filtering, and screening peer reviewed papers based on the content of the abstract, conclusion, and introduction. An additional prerequisite was that the paper be written in English and cover BPM applications of a specific organization. The next step was to filter papers concerning the specific application of BPM, process mining or process automation. Some 40 papers were selected – all peer reviewed and may be seen after our reference list. Of the 40 papers, 31 comprised book chapters discussing how business process management was used to solve a specific industry problem. The remaining 9 case studies were peer reviewed conference papers in management. The case studies excluded those not covering process management or process improvement initiatives. NVIVO 12 software was used for analysis, where deductive coding was applied to the content of the papers. Codes or nodes were uncovered and labelled as: Process Analysis, Process Discovery, Process Identification, Process Redesign, Process Monitoring and Control and Process Implementation. The steps taken in the qualitative analysis are illustrated in figure 1. As we sought to develop a model, we incorporated design science research (DSR) with its focus on artefacts as an output of the research process. Next, we briefly elaborate on the BPM case studies analysed, then discuss our design science approach to develop the framework for this paper.
BPM Lifecycle Case Studies
Again, qualitative analysis of peer reviewed BPM Case Studies across different industries was conducted. Thirty-one papers were BPM cases (vom Brocke & Mendling, 2018) in digital innovation and business transformation in practice, and an additional nine cases were BPM focused papers. The BPM lifecycle activities – process identification, discovery, analysis, redesign, implementation and monitoring and control (Dumas et al., 2018) were the focus of each case study where details may be found in appendix 2 table 2.1. The NVivo 12 software was used to perform cluster analysis and hierarchical visualization as shown in figure 2 [A] and [B]. In process discovery, several methods can be adopted to capture the current condition of the business process of the organization as illustrated in figure 3 [A]. There exist various methods ranging in different levels of abstraction. Automated process discovery is an emerging approach. Other methods include event driven process chains, process architecture or object-oriented BPM, while other organizations leap directly to the process redesign phase. The main goal observed in most of the case studies was to improve the business process. It is also notable that there are varying models and frameworks adopted to achieve this target. In figure 3 [B], a summary of these frameworks, models and methods is mapped in the spectrum of business process redesign, which shows while most organizations trend towards analytical and transactional methods, there are cases exploring transformational and creative methods and more innovative approaches.
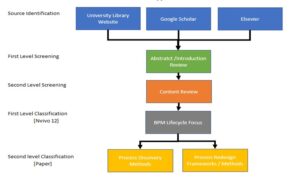
Figure 1: Qualitative Research Flow of BPM Case Studies
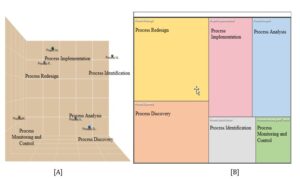
Figure 2: Case Study Analysis [A] and a Cluster [B] Hierarchical Chart using Nvivo 12
Design Science Research
In seeking human knowledge enhancement through the creation of innovative artifacts, design science research (DSR) seeks to enhance science and technology knowledge foundations through the creation of innovative artefacts that solve problems and enhance the environment in which they are instantiated (vom Brocke et al., 2020). A
design artefact can be used to address the quality model and external and internal metrics including quality-in-use metrics (Pries-Heje, Baskerville & Venable, 2008). Six steps are involved in DSR: identifying the problem and motivation, defining the objectives for a solution, analysis for design and development, demonstration, and evaluation (vom Brocke et al., 2020).
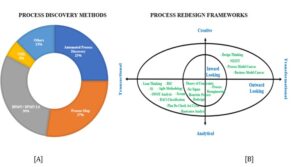
Figure 3 [A] and [B]: Frameworks Adopted for Process Redesign (40 Case Studies)
It is through design science research that procedures, principles and practices provide a nominal process model to provide researchers with a road map. In DSR motivating, designing, developing, demonstrating, communicating, and evaluating the artifact should be consistent (Peffers et al., 2007), where motivating and designing is the initial phase. Developing, demonstrating, and communicating is conducted in the analysis and evaluation phases. Research questions generally evolve around increasing the operational metrics vis-à-vis novel design artifacts (Gregor & Hevner, 2013). Examples of design science research methodology in Information Systems (IS) can also be seen in Baskerville & Vaishnavi (2020) to create a novel approach for benchmarking performance data, and in Winter & Aier (2020) for developing evolution paths for enterprise-wide information systems (e-wIS) using BPM for better quality management (Becker et al., 2020). In this study, we create a novel conceptual framework to introduce a solution to business problems in international education, specifically in the study abroad business process.
DSR played a significant role in performing activities in this study. During the initialization phase for gaining data for extraction, event log data were exported from a process aware information system. In the analysis stage, preparation was a critical step to choose data to be removed from the event log. At this stage, business rules were established. In the process discovery stage, different algorithms were used to explore the most logical process model. The succeeding step was conformance checking, where accuracy of process models generated was measured and validated through a fitness of the model. Finally in the evaluation and performance, checks of the different metrics in the process model were generated. Through DSR, the following significant activity was identified: [1] relevance of performance metrics, [2] effective utilization of process aware information, [3] capability of AI/ ML enabled software, [4] process model extraction in the event log, and [5] identification of process improvement areas. A research framework was then conceptualized (figure 4). In process mining, the fundamental activities are automated process discovery, conformance checking and process enhancement (van der Aalst, 2016). It is through these categories that mining will ensure the model generated is sound, fit, precise, simple, and general, which are the main check items when using an automated process discovery tool. These five features will be measured during conformance checking, where each of the check items is simulated and their performance evaluated. The enhancement step is when process reengineering takes place, as it is conformance checking that enables the identification of bottlenecks in a process and the waiting time between activities which serves as the basis for enhancements.
Process Scenario
Let us now turn our attention to the scenario in this paper. The high-level process architecture of a study abroad application covers the core, support, and management process. The core processes include activities and the student administration team of a student study abroad application (figure 5 and 6). Management processes are responsible for strategy development, partnerships, funding, and internal stakeholders to achieve a study abroad university target, while support processes including systems and application support and business intelligence reporting for oversight on the progress on different projects across the team.
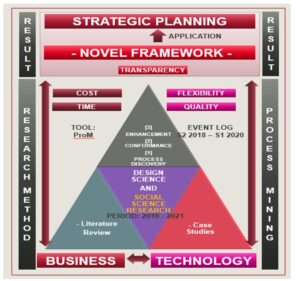
Figure 4: Research Model
Due to the level of abstraction of a process architecture, business processes can be difficult to share, hence it is imperative to construct process models based upon the process architecture as perceived by the modeller (Dumas et al., 2018). Process models can be created through different modelling languages, such as Event-driven Process Chains (EPCs), Petri Nets and Business Processing Modelling Notation (BPMN) (Ishak & Choudhary, 2019). Petri nets are the earliest and most studied process modelling language allowing concurrency, simplicity, and an intuitive graphical notation. However, the longest-established language to model business processes is BPMN (van der Aalst, 2016). The activity to gather and organize information of existing processes and constructing the “As-Is” process model is process discovery. It has been proven that process discovery is a ponderous, tedious and time-consuming practice (Dumas et al., 2018). It is during this phase that a comprehensive depiction of current business processes is specified as an “As-Is” process model (vom Brocke & Mendling, 2018). Figure 6 illustrates the high-level “As – Is” process model of the core processes as shown in figure 5, using BPMN. More detailed process figures are shown in appendix 1 (figures 1-1 to 1.4).
The “As-Is” business process model of a study abroad application is initiated through an information session with the student, organized to discuss the study abroad application process, the requirements, time lines and concerns the student may have (table 1). Application forms which include questionnaires, learning and signature contents are then submitted. Eligibility of the student is then assessed based on a weighted average mark (>50), progression status (a pass progression) and total completed credit points (>24 CP). All eligible students are forwarded to student administration for another assessment which includes available elective units in the course or endorsement from faculty.
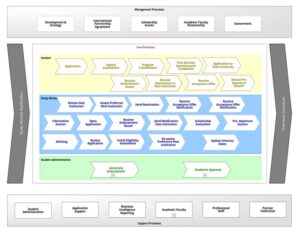
Figure 5: High Level Process Architecture of Study Abroad
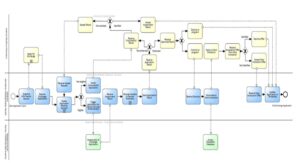
Figure 6: High Level Process Model of a Study-Abroad Application
When the student is endorsed, the study abroad team will then continue the assessment, nominating the student to the specific host institution. Students will apply to a host institution website. When students receive the acceptance letter from the host institution, the status in the study abroad application is changed to ‘Accepted to Host Institution’. An exception process occurs when a student appeals for a failed exchange eligibility, whereupon a preferred host institution is considered as a variant in the ideal process model. Domain knowledge is often held by different stakeholders (Korherr, 2008). In most cases, three different staff are knowledgeable – a process analyst, a subject matter expert and the process owner. The various process discovery methods employed as a guide to gather information are evidence, interview, and workshop process discovery.
Table 1: Steps in the Study Abroad Process (full list in Appendix 1 Table 1.1)
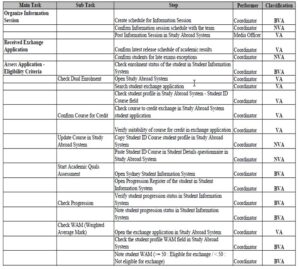
Data Collection
The set of data for process discovery was an event log from a study abroad application, anonymized and converted to XES using ProM Lite 1.3 software containing mandatory columns such as activity ID, activity name and timestamp. The parameters included the following:
- Activity identification is a numeric field referring to each case in the record set.
- Activity name is a text field describing the activity or event.
- Activity timestamp is a text field containing a date and timestamp when the activity execution ended. The column format is “DD-MM-YYYY HH:MM: SS”.
- Resource column is a text field referring to the resource identifier performing the activity; this field has been converted to an equivalent role in the organization for anonymity purposes.
The study abroad application is a cloud-based platform used by students applying to study overseas and the staff that manage the application. The history log records all activities performed in the application from the creation of the application, acceptance by the overseas host institution while abroad and the return phase. In this research, the data are from application creation until acceptance by the host university only – and do not cover activities onwards since these activities have minimal added value in the business process. The source of the event log used is the application process of the study abroad application capturing the end-to-end lifecycle of the student application, as illustrated in figure 7.

Figure 7: Status Transition of Study Abroad Application
The data were from 2018 semester 2 until 2020 semester 1 for semester applications, as well as the winter and summer semester 2018 – 2019 short-term cohort. These data were selected because study abroad applications use commenced last 2018 S2, so a complete application cycle started from 2018 semester 2 and contained a complete history log. While historical data before 2018 semester 2 only contain 1 record per student for tracking purposes. Furthermore, the study abroad program is on hold from 2020 Semester 2 because of the COVID-19 pandemic, while we await Australian government permission to lift travel restrictions. The details in table 2 below represent the summary of data used. The quality of data selected requires reformatting of activity names as there exist irrelevant events, while concept drift, time and date are in separate columns and need to be concatenated into one column, as required by the software (ProM Lite 1.3).
Table 2: Events and Cases Summary of Study Abroad Event Log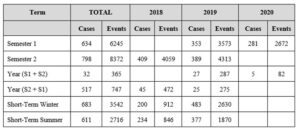
Process Reengineering
With novel digital technologies, existing frameworks are challenged to adapt to the socio-technical impact on individual behaviours, collaborations at the intra and inter-company level, and the need for including automation (Kerpedzhiev et al., 2020). The typical “As-Is” process model can be analysed with qualitative and quantitative methods to identify redundant process steps through value added analysis, examining the origin of waste through waste analysis. Value added analysis involves task decomposition into steps. The step is then analysed and can classify a positive outcome as Value Adding (VA). Steps necessary to the organization, but providing no gain to the customer, may be classified as Business Value Adding (BVA). Steps not belonging to either VA or BVA become Non-Value Adding (NVA) (Dumas et al., 2018). The value-added analysis and classification of steps for the study abroad process is shown in appendix 1 table 1.1 and the excerpt is shown in table 3 below. Waste analysis is the antithesis of value-added analysis and traces steps to identify waste which can be through a specific step or the entire process. Taiichi Ohno developed the Toyota Production System or TPS in the 1970s. One of the key techniques is the reduction of muda – meaning waste in Japanese. The concept is to find waste based on categories involving move, hold, and overdo in the process handoffs (Dumas et al., 2018)
Table 3: List of wastes identified from a Study Abroad Process
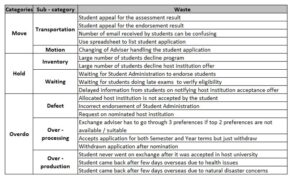
Figure 8 illustrates the revised process model which includes additional data such as cycle time, branching probability and processing time as a sample business process, resulting in total 64.14 of days for the cycle time for study abroad process from the first to last process. In cases where the overall processing time would be relative throughout the cycle of the process, table 4 summarizes the cycle time in the activities of the Study Abroad team with their estimated cycle and process times.
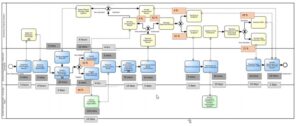
Figure 8: Study Abroad Cycle Times, Processing Times and Branching Probability
Automated Process Model
Recent breakthroughs in process mining enable organizations to automatically discover process models and analyse conformance as to whether the process is reflected in the event log. Detection of deficiencies in performance such as bottlenecks (Schuster et al., 2020), improved business processes using event data (van der Aalst, 2016) and the extraction of insights regarding the actual performance of these processes (Augusto et al., 2019), are also possible.
Table 4: Summary of Cycle and Processing Times for the Study Abroad Process
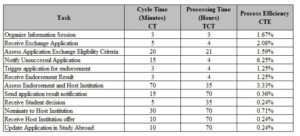
Process mining evolves around business process improvement in a data-driven way (Apromore, 2020), and is a process management approach allowing analytical understanding of business processes based on digital traces captured in event logs (Reinkemeyer, 2020) to extract information (Schuster et al., 2020). An event log contains traces with timestamp-encoded activities undertaken for the execution of a corresponding process (Schuster et al., 2020) or collation of events to execute a business process, where every event refers to a specific activity taking place at a specific timestamp allocated to a unique case (Reinkemeyer, 2020). As a minimum requirement, an event log includes a numeric identifier such as a case ID specifying the ‘activity’ taking place at a precise time – i.e., a ‘timestamp’ of every activity (Reinkemeyer, 2020).
In this digital age, AI is an emerging transformational technology (Chu et al., 2018). The promising capability of ML and AI for prediction in conjunction with process mining results in accurate prediction of erratic processes (Welsing et al., 2020), capable of automatically spotting business process deficiencies and uncovering root cause of issues, while delivering intelligent decisions to enhance process misapplications (Veit et al., 2017). Adopting ML techniques provides consistency, robustness, and precision in predicting convoluted relationships of process sequences, features, or business case characteristics (Mehdiyev, Evermann & Fettke, 2020). Furthermore, explainable AI (XAI) lays the bedrock for prescriptive decision analysis in process mining to mitigate impediments in a data-driven business process intelligence for a significant added value in the organization (Mehdeyiv & Fettke, 2020).
It is process mining that can bridge the gap between analysis of conventional model-based process and data-centred analytical approaches such as ML and data mining. Process mining is not constrained to process discovery of tightly coupled event logs, which makes it viable to confirm conformance, predict delays, deviation detection, substantiate decision making and prescribe process redesign. Process mining techniques, contrary to inactive process models, breathe life into today’s substantial data volumes (van der Aalst, 2016). Discovery uses event logs to construct a graphical illustration of the business process where outcomes are typically a process model, which can either be a classic Petri Net, widely used BPMN, or UML Activity diagrams, and can even be a social network. Conformance relates to the correlation of the event log and actual business process model. Lastly, enhancement identifies improvement points, by utilizing information generated in the event log. Discovered models are a calculated abstraction of real processes, usually focusing on various points of view such as control flows of tasks, data flow of activities, time, resources utilized, or costs spent. The tactical level has a medium-term impact and is based upon extant data where decisions at the operational level have prompted outcomes using running case event data (van der Aalst et al., 2016). Event data are then used to create process models to understand the business further, identify improvement areas or audit, which is made possible through process discovery (Augusto et al., 2018).
Event Logs
A collection of timestamped events or activities is an event log (van der Aalst, 2016), usually stemming from a process aware application. Every event identifies an execution of tasks or processes and can also indicate a message, escalation, or other applicable pertinent events in a specific background of identified process. The event log can be used in most mining techniques. The three attributes as a minimum requirement are a case identifier which is a unique identifier for a set of events, an event class or activity name and a timestamp which includes both date and time. In practice, additional attributes such as resource, cost or other domain specific data are also included. A case identifier is the identification in which case the event happened. An event class is the reference of the event, where the timestamp is the date and time the event occurred (Dumas et al., 2018). The event log of a sample study abroad process is shown in table 5.
Table 5: Excerpt of an Event Log for Study Abroad Application
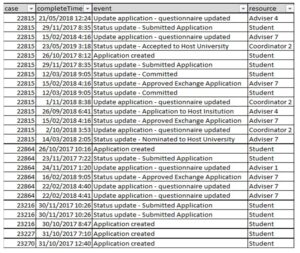
A common format for an event log is a CSV or comma-separated-value. For a more complicated event log containing data attributes in the events, a flat comma delimited file is not an appropriate format, hence an extra functional converted file (the eXtensible Event Stream (XES)) is used to format event logs, which is an acknowledged process mining standard by the IEEE Task Force (Dumas et al., 2018). An XES format is partially depicted in figure 9, which is the processed file of a Study Abroad Event Log. An XES file contains multiple traces, and every trace contains multiple events which contain different attributes in each event (Dumas et al., 2018). The original file was generated directly from a study abroad application in a CSV file. The process mining ProM Lite 1.3 tool was used as the XES converter to generate the equivalent XES file of the study abroad event log.
Framework Formulation
The novel conceptual BPM framework using ML and AI is to be based upon automated process discovery activities to solve business problems. BPM is generally structured through frameworks, describing relevant capability areas for process implementation in organizations (Kerpedzhiev et al., 2020). Again, the process mining tool and operational support application used is ProM Lite v1.3 – a state-of-the-art process mining tool containing plugins for automated process discovery, conformance checking and event enhancement evaluation by means of various algorithms including heuristic, inductive and fuzzy miners that provide data visualization capability. The automated “As-Is” process model (process discovery) will then be used for analysis.
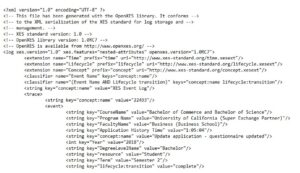
Figure 9: Extract of converted XES format of Study Abroad Event Log
Process mining is a data-driven holistic approach answering questions related to the business process. In this study we seek to answer the following questions about the behaviour of the event log:
- What is the Case ID taking the longest duration from status “Application created” to “Accepted to Host University”?
- What is the Case ID with the shortest duration from status “Application created” to “Accepted to Host University”?
- What activities cause bottlenecks?
- What is the average duration of applications from “Application created” to “Accepted to host University”?
- What are the top three most frequent activities occurring in the process model?
Automated Process Discovery
A plethora of algorithms exist in process mining capturing different levels of abstraction to generate a sound process model, as event logs are typically spaghetti-like with several traces, arcs and events, making them arduous to interpret. Automated process discovery techniques use event logs converted to XES. Using the ProM Lite 1.3 tool, the XES file details of the event log are summarized in table 6. The core processes are the application status indicated in figure 10, which entail the main milestones of the students’ study abroad application, while supplementary processes represent activities added intentionally or ignored. As indicated in table 6, the data set is separated by application cohort to understand the behaviour of every application cycle which has a specific application time frame. By separating different cohorts, the process used in each cohort can be analysed. Details can be drilled down for analysis and then compared to best practice. The algorithms used are the heuristic, inductive, fuzzy and ILP Miners. All algorithms have visualization capability and an animation feature. These highly sophisticated algorithms show how effective this state-of-the-art tool can be used. Let us briefly examine the outputs from each miner.
Heuristic Miner
Use was made here of a heuristic miner and relevant parameters include dependency, conditional, binding and conformance heuristics, where a conditional heuristic has a Cohen Kappa.
Table 6: Summary of Relative Process Occurrences of Study Abroad Process
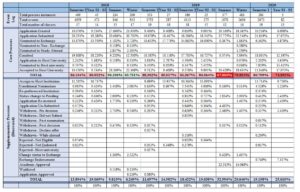
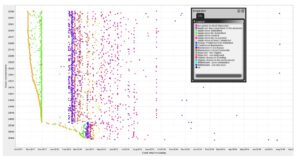
Figure 10: Dot Chart Output of the Study Abroad Process (2018 S2)
An F1 score option is available – including frequency thresholds. The two process models generated by this algorithm are shown in appendix 2 figure 2.1, with directly-follows and dependency graphs. The activity that is mostly executed in the process can be identified easily as “Application Submitted” instead of “Application Created”, meaning study abroad advisers create an application on behalf of the student.
Inductive Miner
The Inductive Miner is a robust process discovery algorithm capable of generating a petri net, a process tree and a BPMN diagram. The state-of-the-art automated process discovery tool satisfies all process model checklists; it has animation capability that shows waiting times, deviations, service, or sojourn times, as indicated in figure 11. The configuration on Inductive Miner – infrequent (IMF) has a 0-noise threshold and a 95% visible path. In addition to the visualization capability of the inductive visual miner, data analysis can be used to dive deep into statistical values of the entire cohort result, which is summarized in appendix 2 – table 2.2 for minimum, average, median, maximum, and standard deviation values. The higher the path settings (in this case 95%), the lower the standard deviation for fitness, which makes it a reliable process model. In the trace duration, the maximum days are 890 days for 2020 Semester, 1, 570 days for Year S1-S2, and 899 days for the winter and summer cohorts.
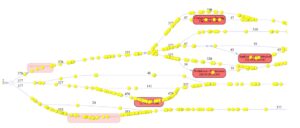
Figure: 11 Excerpt from Inductive Miner Showing Path and Sojourn Times (2019 Summer)
Fuzzy Miner
The Fuzzy miner plugin creates process graphs but not petri nets. The implementation is regarded as practical where insights can be generated, however parallelism or choice is not considered in this algorithm. Generating fuzzy model parameters, such as attenuation, can be set if it is an nth root with radical or linear attenuation and maximal event distance. In appendix 2 – figure 2.2, the fuzzy model for the 2018 Semester 1 cohort is shown; this fuzzy model has a zero-significance node filter, with a fuzzy edge filter of 0.2 cut off and 0.75 utility rate ignoring self-loops. Also used is a concurrency filter set at 0.6 preserve and 0.7 ratio filter concurrency. All 17 traces are shown in the model with 3 orphan activities, making it difficult to understand the precedent activity.
Conformance Checking
To guarantee the conformance of a process model generated from the event log, a different set of data is evaluated by comparing the observed behaviour with the modelled behaviour using an inductive visual miner (IMF Miner) with a 90% path and 100% activities setting, where the result of process model fitness, number of events, and number of log moves, is summarized in table 7. In conformance checking, the replay fitness of the process model is measured. Given that 90% of the path is visible, the model generated has a higher average fitness and a lower standard deviation, making it fit to use. The inductive visual miner was executed using the same parameter with a 90% visible path. The direct-follows miner shows perfect fitness, while the lifecycle miner and all-operators’ miners have the same fitness result as the IMF Miner where the plugin inductive miner – infrequent is used with a 10% noise threshold.
Performance Checking
The data set was evaluated using a petri net with a 0-noise threshold utilizing the MXML legacy classifier and an A* ILP-based manifest replay algorithm.
Table 7: Summary of Standard Deviation for Conformance Checking
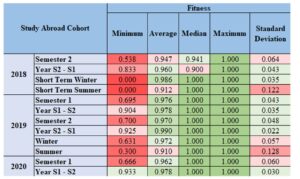
Based on event log completed time, the throughput time is calculated where a bottleneck exists, while further analysis can be done to drill down the element statistics of each task’s throughput, waiting or sojourn time to further compare its performance in the overall duration of the process model. In addition, the level of bottleneck can be identified visually. The summary of throughput time is shown in table 8 which includes best case (minimum) and worst case (maximum) models including the case property. The summary of bottleneck processes found are shown in appendix 2 table 2.3.
Table 8: Summary of Case Throughput Time (A* ILP – Based Manifest Replay Algorithm)
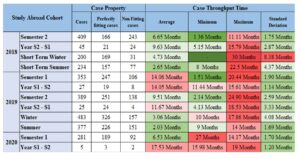
Event Log Probe Questions
One reason for a conventional process map is to grasp the performance of the current process as perceived by subject matter experts and identify areas of improvement, such as automated process discovery. The process model generated through automated process discovery algorithms must address all research questions. All 100% – i.e., all five questions were addressed with sufficient detail. The case ID with the longest and shortest duration can be identified by checking the trace details or through a performance algorithm, determining the bottleneck activities and were summarized in appendix 2 table 2.3. The three most frequent activities can be identified in table 8 where every task shows the ratio is often recurring in each cohort. Several performance metrics of the process can also be further examined, such as service times and waiting times, if further details are required. The succeeding activity would then be a comparison of these data to standard performance metrics of the organization. Discussion of activities desired for addressing problems can then be identified. Subject matter experts played a significant role in extrapolating details to understand the event log and separate the different study abroad cohorts.
Data Quality Assurance
The study abroad application event log contains comprehensive details of activities not directly related to the major milestone of the process. If all activities are included in the data and the actual activity name is used, it will create too much noise which can hamper the event log quality and analysis, because of factors such as [a] the clarity of the output process model; [b] the level of process conformance; [c] analysis of performance results; and [d] difficulty in identifying the correct parameters to be used. Hence as the first author is a subject matter expert embedded in the organization with direct contact to all resources, business rules have been applied to produce a clean event log as noted by Van Eck et al., (2015) that includes information directed to the major activities of a study abroad application. The business rules specifically apply to an activity name where a standard naming convention is used, removing irrelevant activities in the log and anonymizing the resource of each activity. In the case study by Andrews et al., (2017) employing automated process discovery, process mining challenges in this multi-faceted study included legislative changes, key personnel changes, and the semi-structured nature of the business process. Similar challenges also hampered the study abroad team, where an exception had to be considered (Wang et al., 2014) to exclude the Covid-19 affected cohort or to capture organizational process change in every cohort.
Discussion
BPM is already considered a mature domain, whereas process mining is seen as an emerging technology. Nonetheless process mining shows promising results to automatically discover process algorithms, while evaluating conformance and performance. To achieve success in BPM, the performance of the process must be initially measured (Kis et al., 2017). Process performance indicators are typically used for target-oriented analysis of actual process performance (Urnauer et al., 2020). For greater business growth, the potential gain of data driven analyses is through better understood business performance (Wynn & Sadiq, 2019). The rising volatility of current markets results in complex processes where end-to-end definitions of process performance are crucial (Schmitz et al., 2020). Our proposed AI and ML based BPM model is shown in figure 12. The model has 5 levels focusing on performance metrics, process aware information systems, process mining, process innovation and a target output model. It is imperative organisational goals are clear regarding process change implementation for the target process to meet performance metrics. The initial step is to establish performance metrics. The final step is the target process model in the proposed framework to make sure it will not deviate from the target metrics. Establishing performance metrics is the first step, then using process aware information systems containing the event log, followed by process mining activities, will generate the process model automatically through algorithms incorporating a process modeller. Next is process innovation where the process requirement paradigm is used to identify change priorities needed in the process. Lastly, the output process model satisfies the performance metrics established in the initial step.
In a study abroad application, an application cycle is repeated in different terms and has specific targets in every cohort, so setting of performance metrics in a recurring cycle though the previous actual process can be established in the performance metrics and set for the following cycle. In addition, the performance metrics can include the waiting, cycle, processing times as well as the deviation, throughput or resource allocation derived from the devil’s quadrangle (Jansen-Vullers et al., 2007). The devil’s quadrangle is a way of understanding the interaction of different projects’ competing constraints such as time, cost, scope and quality (Jansen-Vullers et al., 2007). Other metrics can also be added if they deliver value to the organization. The study abroad application is temporal, so service, processing or waiting times form a significant part of the performance metrics to be included. One essential component of the framework is a process aware information system (Kalenkova et al., 2015), where the event log is generated and extracted.
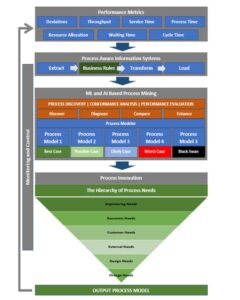
Figure 12: Proposed AI and ML Enabled BPM Framework
The generic procedures of data warehousing for copying data from one source to another – Extract Transform Load (ETL) (Griesemer, 2011), includes an additional business rule definition after Extract; the business rule represents the structured guideline on how the data will be transformed, as raw event logs, directly extracted straight from the system, contain noise or unnecessary activities (Van Eck et al., 2015). Backtracking the process through activities in information systems has massive potential (Menges et al., 2020). A secure, robust business process integration that maintains consistent data is a prime driver of success, where pervasive implementation of process-aware information systems eases the acquisition of digital footprints of a business process (Mehdeyiv & Fettke, 2020).
For study abroad, the process aware information system records all activities undertaken without any filter or exception. In this study, the focus are the major activities relevant to a student application and such activities can be easily identified by a subject matter expert, or semester and short-term coordinators. Through the defined business rules, the transformed event log will be structured and consistent and possess standardized naming conventions and be ready to be loaded as input to a process mining algorithm for automated process discovery. The filtering of relevant activities is descriptive. Output activities are in either BPMN, transition diagrams, process trees or petri nets which are easier to understand and follow, making analysis straightforward. Process mining algorithms, applied in the study abroad application cycle to create process models through automated process discovery and visualize process performance, have shown promising results and can be used instead of manual methods. Configurable parameter options to obtain tolerable conformance levels can be set flexibly. The structure presented in figure 12 is a performance metric focused BPM framework, where process mining algorithms are aligned, as performance metrics play a pivotal role in organization goals and usually trigger business process change initiatives. Process innovation is the end goal of these preliminary activities and ultimately identifies the component of the process most in need of improvement.
The research questions were addressed by comparing a manually created process model with process and cycle times with a probability ratio. Using ML and AI based plugins with state-of-the-art innovative algorithms, we can generate various performance metrics of existing process models using event logs from a process-aware information system. More options are also available in the latter, in terms of visualization and process discovery representation as indicated in appendix 2, which shows a significant difference in terms of the following areas as indicated in RQ1: How do ML and AI-based BPM frameworks vary from conventional business process management solutions in higher education, specifically in a study abroad process in terms of reliability, profitability, cost-effectiveness, and trustworthiness? The automated process discovery is data-driven in that it depicts actual activities and is free from any pre-conceived bias and individual judgment. Process efficiencies are easily identifiable through various actionable visualization options. The automated process discovery output can be checked through conformance algorithms, and configurable parameter options to ensure the output process model is trustworthy. With different performance metrics available, all areas in the process can be met through event logs which represent real time activities in a process aware information system with human intervention. One of the challenges here is creating business rules that make sense of complex activities in the event log. RQ2: What are the pre-requisites in using data-driven and process mining capabilities of ML and AI to effectively implement BPM frameworks in higher education study abroad process? A process aware information system is the main requirement, which is the source of an event log or XES functioning as the input element in the process mining software tool (Kalenkova, 2017). Performance metrics are important to serve as a compass for identifying best or worst-case process models in the event log number of traces. Basic knowledge in using ProM Lite 1.3 is also required.
Conclusion
Creating a process model for different organizations and various domains can be challenging, and requires a specific skill set with additional information requiring investigation during process discovery, analysis, or redesign. The time spent that can be saved when automating process discovery capability is far less than creating a conventional mapping of the process; however, it also requires skill to use a software tool and navigate through the application to capture the information needed. The AI and ML enabled BPM Framework encapsulates a new way of thinking in process-centric change initiatives, where performance objectives form a significant step in any target organization. The phases in the framework (figure 12) are directed specifically at achieving the performance goals at the metrics level. This first step in the framework indicates several metrics can be used as a key performance indicator. In the process mining application stage, the framework shows the process nomenclature where the preferred process model is selected as the ideal process for innovating the existing process. When change is implemented utilizing an ordering of process needs, the output process satisfies the performance goal established. The interesting insight here is that the framework in figure 12 is relatively agile, such that process changes can be justified and implemented in a short period of time, so that performance can be improved to meet the target as often as required.
Limitations and future work
The scope of this study was a study abroad application starting with application commencement until the student is accepted to a host institution. However, the journey of the student mobility experience is only starting at this stage as there are activities involved during student departure, while the student is overseas, when the student completes the exchange program and then returns home. All such activities are considered out of scope and are a limitation of this study; however, they are part of the event log recorded in the system and were thus filtered out. The activity after the student is accepted to host university affects the staff-hours of an exchange adviser. There are special cases where staff support is also needed, and this can consume much time. There are also supplementary activities in the event log, but these were excluded in this study. It would be interesting to examine the end-to-end mobility experience cycle performance compared to a presumed process. The event log used also needs to undergo data cleansing to eliminate noise correctly, meaning good quality data are structured. In addition, the ProM 1.3 machine learning algorithm is not capable of building mathematical models from sample data to make decisions, and hence not capable of prescriptive analytics. Studying overseas as part of a higher education degree has become more attractive to students because of likely higher employability outcomes after graduation. Intake of study abroad applications is expected to increase, particularly with certain countries which are highly preferred by students – such as the USA, UK or the Netherlands. There are also cases where students withdraw from a program while overseas, which could prove helpful to understand trends. One idea is to continue to build an interactive seamless business intelligence application tool using the novel BPM framework integrating different levels. This tool may include a business rules library capable of automatically converting event logs into interactive dashboards to show comparative results between target performance metrics and the deviation of actual data, along with a process modeller library capable of automatic decision making.
References
- Akkiraju, R., Sinha, V., Xu, A., Mahmud, J., Gundecha, P., Liu, Z., Liu, X., Schumacher, J., (2020), “Characterizing Machine Learning Processes: A Maturity Framework”, In proceedings of the 18th International Conference on Business Process BPM 2020, Springer International Publishing, Seville Spain, pp. 25-69.
- Andrews, R., Suriadi, S., Wynn, M., ter Hofstede, AHM., Rothwell, S., (2017), ‘Improving Patient Flows at St. Andrew’s War Memorial Hospital’s Emergency Department Through Process Mining’ in Business Process Management Cases, 1(1), pp. 311–333, Springer International Publishing, Cham.
- Apromore, (2020), “From digital traces to fully-optimized processes”, https://apromore.org/, Date accessed: 12/02/2021
- Augusto, A., Conforti, R., Sumas, M., La Rosa, M., Maggi, F., Marrella, A., Mecella, M., Soo, A., (2019), “Automated Discovery of Process Models form Even Logs: Review and Benchmark”, IEEE Transactions on Knowledge and Data Engineering, 31(4), pp. 686-705.
- Bachiller, Y., Busch, P., Kavakli, M., Hamey, L., (2018), “Survey: Big Data Application in Biomedical Research”, In proceedings of the 2018 10th International Conference on Computer and Automation Engineering, pp. 174-178, Brisbane Australia.
- Bandara, W., Indulska, M., Chong, S., Sadiq, S., (2007), “Major Issues in Business Process Management: An Expert Perspective”, In Proceedings ECIS 2007 – The 15th European Conference on Information Systems, pp. 1240 -1251, St. Gallen Switzerland.
- Baskerville, R., Vaishnavi, V., (2020), ‘A Novel Approach to Collectively Determine Cybersecurity Performance Benchmark Data: Aiding Organizational Cybersecurity Assessment’ in Design Science Research. Cases, pp. 17–41, Springer International Publishing, Cham,.
- Becker, J, Clever, N, Fleischer, S, Höhenberger, S, & Rätzer, S 2020, ‘Using Business Process Management for Effective and Efficient Quality Management: The Icebricks Approach’ in Design Science Research. Cases, pp. 131–154, Springer International Publishing, Cham.
- Brett, A., Croucher, G., Lacy, W., Mueller, R., (2017), “Australian Universities at a Crossroads: Insights from Their Leaders and Implications for the Future”, Melbourne Center for the Study of Higher Education, Melbourne, Australia, “https://apru.org/wp-content/uploads/2018/12/Australian-Universities-at-a-Crossroads.pdf”, Date accessed: 15/10/2020
- Cawood, R., Roche, J., Sharma, D., Jones, L., Kirkhope, J., Ong, A., Mulder, A., Ta, D., (2018), “Can the universities of today lead learning for tomorrow? The University of the Future,” https://www.ey.com/en_au/government-public-sector/can-the-universities-of-today-lead-learning-for-tomorrow”, Ernst & Young, Australia, Date accessed: 15/10/2020
- Cearley, D., Burke, B., Smith, D., Jones, N., Chandrasekaran, A., Lu, S., Karamouzis, F., (2020), “Top 10 Strategic Technology Trends for 2020: A Gartner Trend Insight Report”, Gartner Inc, Connecticut USA, Date accessed: 15/10/2020.
- Chui, M., Manyika, J., Miremadi, M., Henke, N., Chung, R., Nel, P., Malhotra, S., (2018), Notes from the AI frontier: Insights from hundreds of use cases, McKinsey Global Institute, pp. 1-36, USA, last accessed: 26/03/2021
- Davenport, TH., Brynjolfsson, E., McAfee, A., Wilson, HJ., (2019), Artificial Intelligence: The Insights You Need from Harvard Business Review, Harvard Business Review Press, La Vergne.
- Dumas, M, La Rosa, M., Mendling, J., Reijers, HA., (2018), Fundamentals of Business Process Management, 2nd 2018., Springer Berlin Heidelberg, Berlin, Heidelberg.
- Einstein, A., (1954), Ideas and opinions, Broadway Books London Alvin Redman, London United Kingdom.
- Franz, P., Kirchmer, M., (2012), Value-driven business process management: The value-switch for lasting competitive advantage, McGraw Hill Professional, New York.
- Gal, A., Senderovich, A., (2020), “Process Minding: Closing the Big Data Gap”, In proceedings of the 18th International Conference on Business Process Management Forum BPM 2020, Information Systems and Applications, Seville Spain.
- Gartner Inc., (2014), “2020-2022 Emerging Technology Roadmap for Large Enterprises”, Gartner Inc., Connecticut USA
- Gartner Inc., (2020), “Business Process Management (BPM)”, https://www.gartner.com/en/information-technology/glossary/business-process-management-bpm, Date accessed 24/10/2020.
- Gregor, S., Hevner, A., (2013), ‘Positioning and Presenting Design Science Research for Maximum Impact’ MIS Quarterly, 37(2), pp. 337–355.
- Griesemer, B., (2011), Oracle Warehouse Builder 11gR2 Getting Started : Extract, Transform, and Load data to build a Dynamic, Operational Data Warehouse , Packt Publication., Olton, Birmingham.
- Hammer, M., 1990, ‘Reengineering work: don’t automate, obliterate’ Harvard Business Review, 68(4), p. 104–
- Hammer, M., Champy, J., (2001), Reengineering the Corporation: A Manifesto for Business Revolution, fully rev. and updated ed., Nicholas Brealey, London.
- Harmon, P., Tregear, R., (2016), Questioning BPM? 109 Answers by 33 Authors to 15 Questions about Business Process Management, Meghan-Kiffer Press, Tampa, Florida.
- Heindel, T., Weber, I., (2020), “Incentive Alignment of Business Processes” in Business Process Management, pp. 93–110, Springer International Publishing, Cham.
- Ishaq, M., Choudhary, M., (2019), “Automated Consistency Management in BPMN Based Business Process Models”, Intelligence Technologies and Applications, Communications in Computer Science, 932(1), pp 289-300.
- Jansen-Vullers, M., Loosschilder, M., Kleingeld, P., Reijers, H., (2007), Performance measures to evaluate the impact of best practices. In: Business Process Modelling Development and Support Workshop, vol. 1, pp. 359–368. Tapir Academic Press Trondheim
- Jeston, J., (2006), Business Process Management: Practical Guidelines to Successful Implementations, Oxford Butterworth-Heinemann.
- Kalmijn, P., (2019), “Applying Artificial Intelligence in Existing Business Processes”, https://atos.net/en/blog/applying-artificial-intelligence-in-existing-business-processes, Date accessed: 27/08/2020.
- Kalenkova, A., van der Aalst, W., Lomazova, I., Rubin, V., (2017), “Processing Mining using BPMN: Relating Event Logs and Process Models Process Mining using BPMN”, Software System Model, 16(1), pp. 1019-1048
- Karamouzis, F., Stoudt-Hansen, S., (2020), “The Gartner 2021 Predictions: Accelerate Results Beyond RPA to Hyperautomation”, Gartner Inc., Connecticut USA
- Kerpedzhiev, G., König, U., Röglinger, M., Rosemann, M., (2021), ‘An Exploration into Future Business Process Management Capabilities in View of Digitalization: Results from a Delphi Study’ Business & Information Systems Engineering, 63(2), pp. 83–96.
- Kiela, D., Bulat, L., Vero, A., Clark, S., (2016), “Virtual Embodiment: A Scalable Long-Term Strategy for Artificial Intelligence Research”, 30th Conference on Neural Information Processing Systems, Association of Computing Machinery, Barcelona Spain.
- Kis, I., Bachhofner, S., Di Ciccio, C., Mendling, J., (2017), ‘Towards a Data-Driven Framework for Measuring Process Performance’ in Enterprise, Business-Process and Information Systems Modeling, vol. 287, pp. 3–18, Springer International Publishing, Cham.
- Knoll, D., Reinhart, G., Prüglmeier, M., (2019), ‘Enabling value stream mapping for internal logistics using multidimensional process mining’ Expert Systems with Applications, vol. 124, pp. 130–142.
- Ko, R., Lee, S., Lee, E., (2009), “Business Process Management (BPM) standards: A Survey”, Business Process Management Journal, 15(5), pp. 744-791, Emerald Group Publishing Limited West Yorkshire England.
- Korherr, B., (2008), Business Process Modelling: Languages, Goals, and Variabilities VDM Verlang Dr. Muller, Saarbrücken, Germany.
- Kumar, A., (2018), Business process management, Routledge, New York.
- Labor Force Australia, (2019), “Australia Main Industry Drivers”, https://www.abs.gov.au/statistics//
industry/industry-overview/australian-industry/latest-release, Date accessed 24/10/2020
- Lederer, M., Popova, O., Schmid, P., (2019), “Can you see the wood for the trees? Collection and Compilation of Agility Models for BPM”, Proceedings of S-BPM 2019, Doi: https://doi.org/10.1145/3329007.3329016, Sevilla Spain, 11 pages.
- Leemans, S., Fahland, D., van der Aalst W., (2013), “Discovering Block-Structure Process Models from Event Logs – A Constructive Approach”, Proceedings in Application and Theory of Petri Nets and Concurrency: 34th International Conference, PETRI NETS 2013, Milan, Italy, June 24-28, 2013. , pp. 311-329, Springer Berlin, Heidelberg.
- Martin, K., Osterling, M., (2013), Value Stream Mapping: How to Visualize Work and Align Leadership for Organizational Transformation, McGraw-Hill Education, New York.
- Mehdiyev, N., Evermann, J., Fettke, P., (2020), A Novel Business Process Prediction Model Using a Deep Learning Method Business & Information Systems Engineering, 62(2), pp. 143–157.
- Mehdiyev, N., Fettke, P., (2020), Explainable Artificial Intelligence for Process Mining: A General Overview and Application of a Novel Local Explanation Approach for Predictive Process Monitoring. [Manuscript] Archive, arXiv preprint arXiv:2009.02098, Saarbrücken, Germany.
- Mendling, J., (2008), Metrics for Process Models: Empirical Foundations of Verification, Error Prediction, and Guidelines for Correctness (Lecture Notes in Business Information Processing), Springer-Verlag Berlin Heidelberg, Germany
- Mendling, J., Decker, G., Reijers, H., Hull, R., Weber, I., (2018), “How do Machine Learning, Robotic Process Automation, and Blockchains Affect the Human Factor in Business Process Management”, Communications of the Association for Information Systems, 43(1), 297-320, doi: 10.17705/1CAIS.04319.
- Menges, A., Dölle, C., Riesener, M., Schuh, G., (2020), ‘Process Cost Calculation Using Process Data Mining’ in Production at the leading edge of technology, pp. 581–590, Springer Berlin Heidelberg, Berlin, Heidelberg.
- Modig, N., Ahlstroem, P., (2019), This is Lean: Resolving the Efficiency Paradox, Rheologica Publishing, Stockholm Sweden
- Munoz-Gama, J., Carmona, J., van der Aalst, WMP., (2013), Hierarchical Conformance Checking of Process Models Based on Event Logs Application and Theory of Petri Nets and Concurrency, pp. 291–310, Springer Berlin Heidelberg, Berlin, Heidelberg.
- Neuman, L., (2014), Social research methods: qualitative and quantitative approaches, Seventh edition, Pearson new international edition., Pearson, Harlow, Essex.
- Object Management Group: Business Process Model and Notation (BPMN), (2011), Version 2.0. http://www.omg.org/spec/BPMN/2.0, Mildford MA USA
- Paschek, D., Luminosu, C., Draghici, A., (2017), “Automated Business Process Management – In Times of Digital Transformation Using Machine Learning or Artificial Intelligence”, In MATEC Web Conferences, 21(1), p. 04007-04015.
- Peffers, K., Tuunanen, T., Rothenberger, M.A., Chatterjee, S., (2007), ‘A Design Science Research Methodology for Information Systems Research’ Journal of Management Information Systems, 24(3), pp. 45–77.
- Porter M., (1985), Competitive Advantage – Creating and Sustaining Superior Performance: with a New Introduction, First Edition, The Free Press, New York, United States of America
- Pries-Heje, J., Baskerville, R., Venable, J.R., (2008), ‘Strategies for design science research evaluation’, In proceedings of 16th European Conference on Information Systems (ECIS 2008), Galway Ireland
- Reinkemeyer, L., (2020), Process Mining in Action Principles, Use Cases and Outlook, Springer Nature Switzerland.
- Rock, G., Dwyer, T., (2014), What is BPM Anyway? Business Process Management Explained, https://www.bpminstitute.org/resources/articles/what-bpm-anyway-business-process-management-explained#, Date Accessed: 24/10/2020.
- Rosemann, M., Bruin, T., (2005), “Towards a Business Process Management Maturity Model”, In Proceedings of the 13th European Conference on Information Systems, pp. 521-532.
- Rother, M., Shook, J., (2009), Learning to see: Value Stream Mapping to Add Value and Eliminate Muda, Cambridge MA USA.
- Schmitz, S., Renneberg, F., Cremer, S., Gützlaff, A., Schuh, G., (2020), ‘Definition of Process Performance Indicators for the Application of Process Mining in End-to-End Order Processing Processes’ in Production at the leading edge of technology, pp. 670–679, Springer Berlin Heidelberg, Berlin, Heidelberg.
- Schuster, D., van Zelst, S., (2020), “Online Process Monitoring Using Incremental State-Space Expansion: An Exact Algorithm”, In proceedings of the 18th International Conference on Business Process Management BPM 2020, Information Systems and Applications, Springer Nature Switzerland, Seville Spain, 18(1), pp. 147- 164.
- Smith, H., Fingar, P., (2003), “Business Process Management: The Third Wave”, World War Tampa Florida USA: Meghan-Kiffer, 2003(1) pp. 1-10.
- Snow, R., (2021), “9 Hacks to Accelerate Digital Business”, Gartner Inc., Connecticut USA, https://www.gartner.com/smarterwithgartner/9-hacks-to-accelerate-digital-business, Date accessed: 12/02/2021
- Urnauer, C., Gräff, V., Tauchert, C., Metternich, J., (2020), ‘Data-Assisted Value Stream Method’ in Production at the leading edge of technology, pp. 660–669, Springer Berlin Heidelberg, Berlin, Heidelberg.
- van der Aalst, W., Adriansyah, A., van Dongen, B., (2011), ‘Causal Nets: A Modeling Language Tailored towards Process Discovery’ in CONCUR 2011 – Concurrency Theory, vol. 6901, pp. 28–42, Springer Berlin Heidelberg, Berlin, Heidelberg.
- van der Aalst, W., Buijs, J., van Dongen, B., (2012), ‘Towards Improving the Representational Bias of Process Mining’ in Data-Driven Process Discovery and Analysis, vol. 116, pp. 39–54, Springer Berlin Heidelberg, Berlin, Heidelberg.
- van der Aalst, W., Weijters, T., Maruster, L., (2004), ‘Workflow mining: discovering process models from event logs’ IEEE Transactions on Knowledge and Data Engineering, vol. 16, no. 9, pp. 1128–1142.
- van der Aalst, W., (2016), Process Mining Data Science in Action, 2nd ed. 2016., Springer Berlin Heidelberg, Berlin, Heidelberg.
- van der Aalst, W., (2020), ‘Academic View: Development of the Process Mining Discipline’ in Process Mining in Action, pp. 181–196, Springer International Publishing, Cham.
- Van Eck, M.L., Lu, X., Leemans, S.J., van der Aalst, W., (2015), PM2: A Process Mining Project Methodology, Lecture Notes in Computer Science, 9097, pp. 297-313
- Veit, F., Geyer-Klingeberg, J., Madrzak, J., Haug, M., Thomson, J., (2017), ‘The Proactive Insights Engine: Process Mining meets Machine Learning and Artificial Intelligence’ Business Process Management: 15th International Conference, BPM 2017, Barcelona, Spain, September 10-15, 2017, Demos
- vom Brocke, J., Mendling, J., (2017), Frameworks of Business Process Management: A Taxonomy for Business Process Management Cases, Business Process Management Cases, Springer International Publishing
- vom Brocke, J., Mendling, J., (2018), Business process Management Cases: Digital Innovation and Business Transformation in Practice, Springer International Publishing, Cham Switzerland
- vom Brocke, J., Rosemann, M., (2014), Handbook on Business Process Management 1: Introduction, Methods, and Information Systems 2nd Springer Berlin / Heidelberg, Berlin, Heidelberg
- Wang, Y., Caron, F., Vanthienen, J., Huang, L., Guo, Y., (2014), ‘Acquiring logistics process intelligence: Methodology and an application for a Chinese bulk port’ Expert Systems with Applications, vol. 41(1), pp. 195–209.
- Weijters, A., Ribeiro, J., (2011), ‘Flexible Heuristics Miner (FHM)’ in 2011 IEEE Symposium on Computational Intelligence and Data Mining (CIDM), pp. 310–317.
- Weske, M., (2012), Business Process Management: Concepts, Languages, Architectures 2nd Edition, Springer Berlin / Heidelberg, Berlin, Heidelberg
- Winter, R., Aier, S., (2020), ‘Designing Evolution Paths for Enterprise-Wide Information Systems’ in Design Science Research. Cases, pp. 75–104, Springer International Publishing, Cham.
- Wong, J., Tay, G., Chiu, M., Stewart, B, (2020), Success in the Digital Experience Economy Requires Connecting MX, UX, CX, EX, Gartner Inc Connecticut USA, Date accessed: 24/9/2020
- Workflow Management Coalition: XML Process Definition Language, (2012) Version 2.2. In: Technical Report WFMC-TC-1025, Workflow Management Coalition, Lighthouse Point, Florida, USA
- Wynn, M.T., Sadiq, S., (2019), ‘Responsible Process Mining – A Data Quality Perspective’ in Business Process Management, pp. 10–15, Springer International Publishing, Cham.
Case Study References
- Case study 1: Reisert, C., Zelt, S., Wacker, J., (2017), ‘How to Move from Paper to Impact in Business Process Management: The Journey of SAP’ in Business Process Management Cases, vol. 1(1), pp. 21–36, Springer International Publishing, Cham.
- Case study 2: Blasini, J., Leist, S., Merkl, W., (2017), ‘Developing and Implementing a Process-Performance Management System: Experiences from S-Y Systems Technologies Europe GmbH—A Global Automotive Supplier’ in Business Process Management Cases, vol. 1 (1), pp. 37–55, Springer International Publishing, Cham.
- Case study 3: Viaene, S., Van den Bergh, J., (2017), ‘Fast Fish Eat Slow Fish: Business Transformation at Autogrill’ in Business Process Management Cases, vol.1(1), pp. 149–166, Springer International Publishing, Cham.
- Case study 4: Bandara, W., Syed, R., Ranathunga, B., Sampath Kulathilaka, KB., (2017), ‘People-Centric, ICT-Enabled Process Innovations via Community, Public and Private Sector Partnership, and e-Leadership: The Case of the Dompe eHospital in Sri Lanka’ in Business Process Management Cases, vol.1(1), pp. 125–148, Springer International Publishing, Cham.
- Case study 5: Czarnecki, C., (2017), ‘Establishment of a Central Process Governance Organization Combined with Operational Process Improvements: Insights from a BPM Project at a Leading Telecommunications Operator in the Middle East’ in Business Process Management Cases, vol. 1 (1), pp. 57–76, Springer International Publishing.
- Case study 6: Kovačič, A., Hauc, G., Buh, B., Štemberger, MI., (2017), ‘BPM Adoption and Business Transformation at Snaga, a Public Company: Critical Success Factors for Five Stages of BPM’ in Business Process Management Cases, vol.1(1), pp. 77–89, Springer International Publishing, Cham.
- Case study 7: Woliński, B., Bala, S., (2017), ‘Comprehensive Business Process Management at Siemens: Implementing Business Process Excellence’ in Business Process Management Cases, vol.1(1), pp. 111–124, Springer International Publishing, Cham.
- Case study 8: Kim, TTT., Weiss, E., Ruhsam, C., Czepa, C., Tran, H., Zdun, U., (2017), ‘Enabling Flexibility of Business Processes Using Compliance Rules: The Case of Mobiliar’ in Business Process Management Cases, vol.1(1), pp. 91–109, Springer International Publishing, Cham.
- Case study 9: Rosemann, M., (2017), ‘The NESTT: Rapid Process Redesign at Queensland University of Technology’ in Business Process Management Cases, vol.1(1), pp. 169–185, Springer International Publishing, Cham.
- Case study 10: Van Looy, A., Rotthier, S., 2017, ‘Kiss the Documents! How the City of Ghent Digitizes Its Service Processes’ in Business Process Management Cases, vol.1(1), pp. 187–204, Springer International Publishing, Cham.
- Case study 11: Cereja, JR., Santoro, FM., Gorbacheva, E., Matzner, M., (2017), ‘Application of the Design Thinking Approach to Process Redesign at an Insurance Company in Brazil’ in Business Process Management Cases, vol.1(1), pp. 205–233, Springer International Publishing, Cham.
- Case study 12: Karle, T., Teichenthaler, K., (2017), ‘Collaborative BPM for Business Transformations in Telecommunications: The Case of “3”’ in Business Process Management Cases, vol.1(1), pp. 235–255, Springer International Publishing, Cham.
- Case study 13: Marengo, E., Dallasega, P., Montali, M., Nutt, W., Reifer, M., (2017), ‘Process Management in Construction: Expansion of the Bolzano Hospital’ in Business Process Management Cases, vol.1(1), pp. 257–274, Springer International Publishing, Cham.
- Case study 14: Andrews, R., Suriadi, S., Wynn, M., ter Hofstede, AHM., Rothwell, S., (2017), ‘Improving Patient Flows at St. Andrew’s War Memorial Hospital’s Emergency Department Through Process Mining’ in Business Process Management Cases, vol.1(1), pp. 311–333, Springer International Publishing, Cham.
- Case study 15: Thaler, T., Norek, S., De Angelis, V., Maurer, D., Fettke, P., Loos, P., (2017), ‘Mining the Usability of Process-Oriented Business Software: The Case of the ARIS Designer of Software AG’ in Business Process Management Cases, vol.1(1), pp. 291–310, Springer International Publishing, Cham.
- Case study 16: Andrews, R., Wynn, M., ter Hofstede, AHM., Xu, J., Horton, K., Taylor, P., Plunkett-Cole, S., (2017), ‘Exposing Impediments to Insurance Claims Processing: Compulsory Third Party Insurance in Queensland’ in Business Process Management Cases, vol.1(1), pp. 275–290, Springer International Publishing, Cham.
- Case study 17: Matzner, M., Plenter, F., Betzing, JH., Chasin, F., von Hoffen, M., Löchte, M., Pütz, S., Becker, J., (2017), ‘CrowdStrom: Analysis, Design, and Implementation of Processes for a Peer-to-Peer Service for Electric Vehicle Charging’ in Business Process Management Cases, vol.1(1), pp. 337–359, Springer International Publishing, Cham.
- Case study 18: Duelli, C., Keller, R., Manderscheid, J., Manntz, A., Röglinger, M., Schmidt, M., (2017), ‘Enabling Flexible Laboratory Processes: Designing the Laboratory Information System of the Future’ in Business Process Management Cases, vol. 1(1), pp. 361–379, Springer International Publishing, Cham.
- Case study 19: Rau, I., Rabener, I., Neumann, J., Bloching, S., (2017), ‘Managing Environmental Protection Processes via BPM at Deutsche Bahn: FINK: The Information System for Nature Conservation and Compensation’ in Business Process Management Cases, vol.1(1), pp. 381–396, Springer International Publishing, Cham.
- Case study 20: Debois, S., Hildebrandt, T., Marquard, M., Slaats, T., (2017), ‘Hybrid Process Technologies in the Financial Sector: The Case of BRFkredit’ in Business Process Management Cases, vol.1(1), pp. 397–412, Springer International Publishing, Cham.
- Case study 21: Becker, J., Clever, N., Holler, J., Neumann, M., (2017), ‘Business Process Management in the Manufacturing Industry: ERP Replacement and ISO 9001 Recertification Supported by the icebricks Method’ in Business Process Management Cases, vol.1(1), pp. 413–429, Springer International Publishing, Cham.
- Case study 22: Schrepfer, M., Kunze, M., Obst, G., Siegeris, J., (2017), ‘Why Are Process Variants Important in Process Monitoring? The Case of Zalando SE’ in Business Process Management Cases, vol.1(1), pp. 431–448, Springer International Publishing, Cham.
- Case study 23: Leitz, R., Solti, A., Weinhard, A., Mendling, J., (2017), ‘Adoption of RFID Technology: The Case of Adler—A European Fashion Retail Company’ in Business Process Management Cases, vol.1(1), pp. 449–461, Springer International Publishing, Cham.
- Case study 24: Suchy, J., Suchy, M., Rosik, M., Valkova, A., (2017), ‘Automate Does Not Always Mean Optimize: Case Study at a Logistics Company’ in Business Process Management Cases, vol.1(1), pp. 463–483, Springer International Publishing, Cham.
- Case study 25: Schindlbeck, B., Kleinschmidt, P., (2017), ‘Integrate Your Partners into Your Business Processes Using Interactive Forms: The Case of Automotive Industry Company HEYCO’ in Business Process Management Cases, (1)1, pp. 485–501, Springer International Publishing, Cham.
- Case study 26: Kloppenburg, M., Kettenbohrer, J., Beimborn, D., Bögle, M., (2017), ‘Leading 20,000+ Employees with a Process-Oriented Management System: Insights into Process Management at Lufthansa Technik Group’ in Business Process Management Cases, vol.1(1), pp. 505–520, Springer International Publishing, Cham.
- Case study 27: Imgrund, F., Janiesch, C., Rosenkranz, C., (2017), ‘“Simply Modeling”: BPM for Everybody-Recommendations from the Viral Adoption of BPM at 1&1’ in Business Process Management Cases, vol.1(1), pp. 521–540, Springer International Publishing, Cham.
APPENDIX 1
STUDY ABROAD PROCESS DETAILS
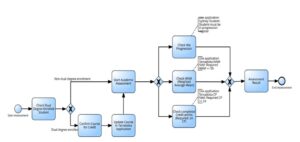
Figure 1.1: Semester Exchange: Expanded “Assess Application – Eligibility Criteria” Process
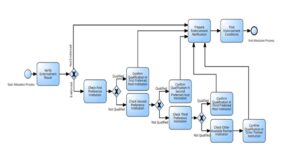
Figure 1.2: Semester Exchange: Expanded process for “Assess endorsement and Host Institution Preference” Process
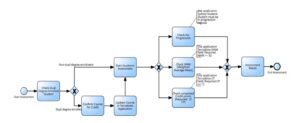
Figure 1.3: Short Term Program Process Map
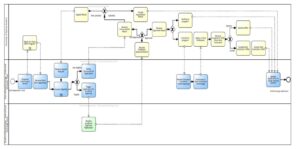
Figure 1.4: Short Term Program. Expanded process for “Assess Eligibility”
Table 1.1: Detailed Activity Steps and Value Classification for Process Map
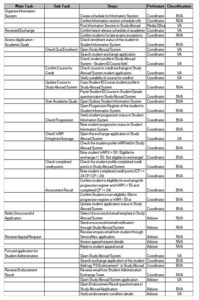
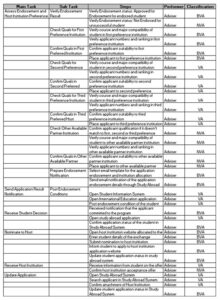
APPENDIX 2 RESULTS
Table 2.1: Summary of Case Studies and BPM Lifecycle Main Focus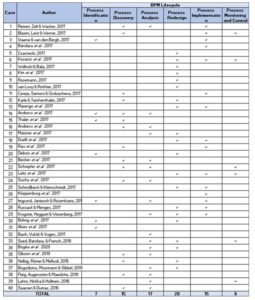
Table 2.2: Summary of Fitness, Duration and Timestamp using Inductive Miner
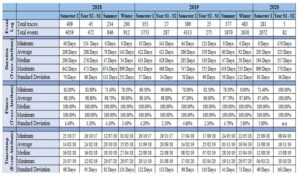
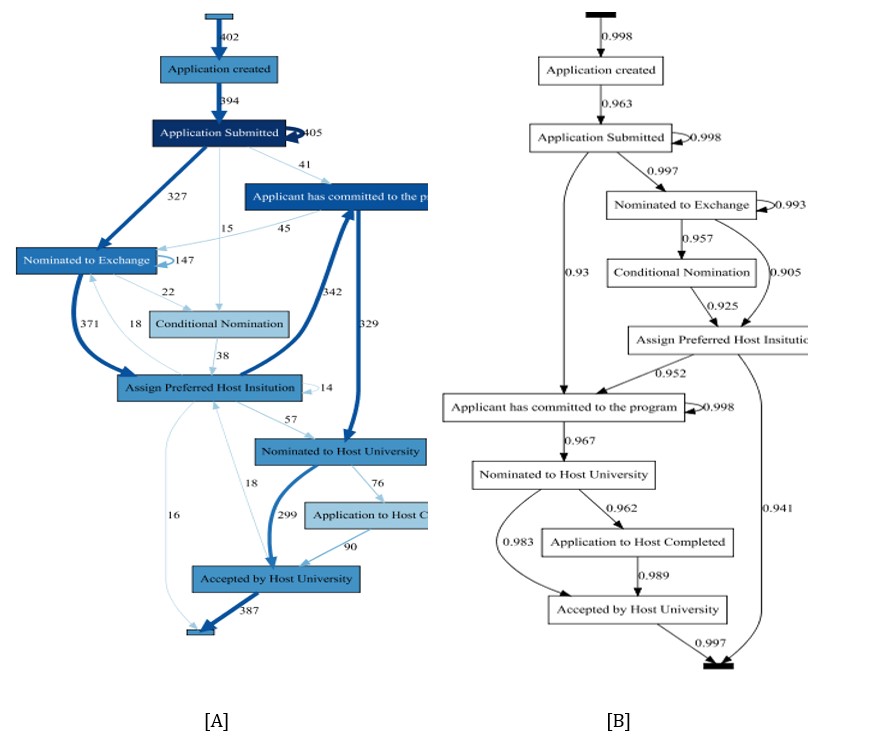
Figure 2.1: Heuristic Miner [A] Directly-Follows Graph [B] Dependency Graph of Study
Abroad Event Log (2018 S2)
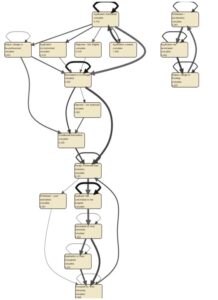
Figure 2.2: Fuzzy Model For (2018 S2)
Table 2.3: Summary of Bottleneck Activities Identified in Performance Checking
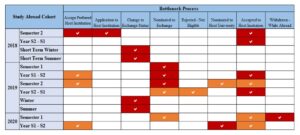